AI Ethics #10 : Truth decay, fighting hate speech, data custodians, trends in ML scholarship, future of privacy and security in ML, and more ...
Our tenth weekly edition covering research and news in the world of AI Ethics
Welcome to the tenth edition of our weekly newsletter that will help you navigate the fast changing world of AI Ethics! Every week we dive into research papers that caught our eye, sharing a summary of those with you and presenting our thoughts on how it links with other work in the research landscape. We also share brief thoughts on interesting articles and developments in the field. More about us on: https://montrealethics.ai/about/
If someone has forwarded this to you and you want to get one delivered to you every week, you can subscribe to receive this newsletter by clicking below
We have two sessions coming up that will provide insights into the European Commission AI Whitepaper, you can find more details on that towards the end of this newsletter under the “Events” section or take a look here!
Our sessions on Publication Norms for Responsible AI were packed with insights from community members that joined us from many countries from around the world, we went into the depths of the questions trying to find answers to some of the thorniest challenges when it comes to publishing about high-stakes research.
“ … one important aspect in upholding ‘responsible AI’ is the consideration of when and how to publish novel research in a way that maximizes benefits while mitigating potential harms.” - Partnership on AI.
In research summaries this week, we look at troubling trends in machine learning scholarship, how social biases in NLP systems act as barriers for persons with disabilities, and the future of privacy and security for machine learning systems.
In article summaries, we cover how AI advances are helping to combat hate speech online, how algorithms algorithms associating appearance and criminality have a dark past, how “truth decay” is harming America’s coronavirus recovery, how ethics in technology is too big a word, thinking like a data custodian is rather than a data owner, and the pitfalls of futuristic background checks.
Our learning communities have received an overwhelming response! Thank you everyone!
We operate on the open learning concept where we have a collaborative syllabus on each of the focus areas and meet every two weeks to learn from our peers. We are starting with 5 communities focused on: disinformation, privacy, labor impacts of AI, machine learning security and complex systems theory. You can fill out this form to receive an invite!
Hoping you stay safe and healthy and looking forward to seeing you at our upcoming public consultation sessions (virtually!) and our learning communities! Enjoy this week’s content!
Research:
Let's look at some highlights of research papers that caught our attention at MAIEI:
Troubling Trends in Machine Learning Scholarship by Zachary Lipton and Jacob Steinhardt
With the explosion of people working in the domain of ML and the prevalence of the use of preprint servers like arXiv, there are some troubling trends that have been identified by the authors of this paper when it comes to scholarship in ML. Specifically, the authors draw attention to some common issues that have become exacerbated in the field due to a thinning experienced reviewer pool who are increasingly burdened with larger numbers of papers to review and might need to default to checklist type of patterns in evaluating papers. A misalignment of incentives when it comes to communicating and expressing the results of the papers and the subject matter associated with them in terms that might draw the attention of investors and other entities who are not finely attuned to pick up flaws in scholarship. Lastly, a complacency in the face of progress whereby weak arguments are seen as acceptable in the face of strong quantitative and empirical evidence also makes this problem tough to handle.
The authors point to some common trends that they have observed such as the use of suitcase words that have multiple meanings and no consensus in how they are used which leads to researchers talking across each other. Conflation of terms and overloading existing technical definitions thus making results and the subject matter more impressive than it actually is also hampers the quality of scholarship. Using suggestive definitions where terms have other colloquial meanings and can be misconstrued to infer that the capabilities of the systems are far beyond what is actually presented is especially problematic when such research gets picked up by journalists and policymakers who use these to make decisions that are ill-informed.
Finally, the authors do provide recommendations on how this can be improved by sharing that authors should consider why they get certain results, what they mean more so focusing on just how they got to the results. Trying to avoid these anti-patterns in scholarship and practicing diligence and critical assessment prior to submitting work to be considered for publishing will also help counter the negative impacts. For those that are reviewing such work, the guidance is to cut through the jargon, unnecessary use of math, anthropomorphization to exaggerate results, and critically asking the question why the authors arrived at results and evaluating arguments for strength and cohesion rather than just looking at empirical findings that compete in producing better SOTA results will also help to alleviate the problem.
Given that the field has seen a resurgence in publication and that the impacts from this are widespread, the authors of this paper call on the community to aspire to higher quality of scholarship and do their part in minimizing the unintended consequences that occur when you have poor quality of scholarship circulating.
To delve deeper, read our full summary here.
Social Biases in NLP Models as Barriers for Persons with Disabilities by Hutchinson et al.
When studying for biases in NLP models, there is not enough of a focus on the impacts that phrases related to disabilities has on the more popular models and how it skews and biases downstream tasks especially when using popular models like BERT and using tools like Jigsaw to do toxicity analysis of phrases. This paper presents an analysis of how toxicity changes based on the use of recommended vs. non-recommended phrases when talking about disabilities and how results are impacted when using them in downstream contexts such as when writers are nudged to use certain phraseology that moves them away from expressing themselves fully reducing their dignity and autonomy. It also looks at the impacts that this has in online content moderation whereby there is a disproportionate impact on the communities because of the heavy bias in censoring content that has these phrases even when they might be used in constructive contexts such as communities discussing the conditions and engaging with other hate speech to debunk myths. Given that more and more content moderation is being turned over to automated tools, this has the potential to suppress the representation of people with disabilities in online fora where they discuss using such phrases thus also skewing the social attitudes and perception of the prevalence of these conditions as being less prevalent than they actually are. The authors point to a World Bank study that mentions that approximately 1 billion people around the world have some form of disability.
They also look at the biases that are captured in the BERT model where there is a negative association between the recommended phrases for disability and associations with things like homelessness, gun violence, and other socially negative terms leads to a slant that impacts and shapes the representations of these words that are captured in the models. Since such models are used widely in many downstream tasks, the impacts are amplified and present themselves in unexpected ways. The authors finally make some recommendations on how to counter some of these problems by involving communities more directly and learning how to be more representative and inclusive. Making disclosures about the places where the models are appropriate to use, where they shouldn’t be used, and the underlying datasets that were used to train the system can also help people make more informed decisions about when to use and when not to use these systems so that they don’t perpetuate harm on their users.
To delve deeper, read our full summary here.
From our learning communities:
Research that we covered in the learning communities at MAIEI this week, summarized for ease of reading:
Warning Signs: The Future of Privacy and Security in the Age of Machine Learning
Summary contributed by Victoria Heath, Communications Manager at Creative Commons
Authors of full paper: Sophie Stalla-Bourdillon, Brenda Leong, Patrick Hall, and Andrew Burt
Articles:
Let’s look at highlights of some recent articles that we found interesting at MAIEI:
AI advances to better detect hate speech (Facebook AI Research)
Detection and removal of hate speech is particularly problematic, something that has been exacerbated as human content moderators have been scarce in the pandemic related measures as we covered here. So are there advances in NLP that we could leverage to better automate this process? Recent work from Facebook AI Research shows some promise. Developing a deeper semantic understanding across more subtle and complex meanings and working across a variety of modalities like text, images and videos will help to more effectively combat the problem of hate speech online. Building a pre-trained universal representation of content for integrity problems and improving and utilizing post-level, self-supervised learning to improve whole entity understanding has been key in improving hate speech detection. While there are clear guidelines on hate speech, when it comes to practice there are numerous challenges that arise from multi-modal use, differences in cultures and context, differences in idioms, language, regions, and countries. This poses challenges even for human reviewers who struggle with identifying hate speech accurately.
A particularly interesting example shared in the article points out how text which might seem ambiguous when paired with an image to create a meme can take a whole new meaning which is often hard to detect using traditional automated tooling. There are active efforts from malicious entities who craft specific examples with the intention of evading detection which further complicates the problem. Then there is the counterspeech problem where a reply to hate speech that contains the same phrasing but is framed to counter the arguments presented can be falsely flagged to be brought down which can have free speech implications.
The relative scarcity of examples of hate speech in its various forms in relation to the larger non-hate speech content also poses a challenge for learning, especially when it comes to capturing linguistic and cultural nuances. The new method proposed utilizes focal loss which aims to minimize the impact of easy-to-classify examples on the learning process which is coupled with gradient blending which computes an optimal blend of modalities based on their overfitting patterns. The technique called XLM-R builds on BERT by using a new pretraining recipe called RoBERTa that allows training on orders of magnitude more data for longer periods of time. Additionally, NLP performance is improved by learning across languages using a single encoder that allows learning to be transferred across languages. Since this is a self-supervised method, they can train on large unlabeled datasets and have also found some universal language structures that allow vectors with similar meanings across languages to be closer together.
Algorithms associating appearance and criminality have a dark past (Aeon)
Facial recognition technology (FRT) continues to get mentions because of the variety of ways that it can be misused across different geographies and contexts. With the most recent case where FRT is used to determine criminality, it brings up an interesting discussion around why techniques that have no basis in science, those which have been debunked time and time again keep resurfacing and what we can do to better educate researchers on their moral responsibilities in pursuing such work. The author of this article gives some historical context for where phrenology started, pointing to the work of Francis Galton who used the “photographic composite method” to try and determine characteristics of one’s personality from a picture. Prior, measurements of skull size and other facial features wasn’t deemed as a moral issue and the removal of such techniques from discussion was done on the objection that claims around the localization of different brain functions was seen as antithetical to the unity of the soul according to Christianity.
The authors of the paper that is being discussed in the article saw only empirical concerns with the work that they put forth and didn’t see any of the moral shortcomings that were pointed out. Additionally, they justified the work as being only for scientific curiosity. They also failed to realize the various statistical biases introduced in the collection of data as to the disparate rates of arrests, and policing, the perception of different people by law enforcement, juries, and judges and historical stereotypes and biases that confound the data that is collected.Thus, the labeling itself is hardly value-neutral. More so, the authors of the study framed criminality as an innate characteristic rather than the social and other circumstances that lead to crime.
Especially when a project like this resurrects class structures and inequities, one must be extra cautious of doing such work on the grounds of “academic curiosity”. The author of this article thus articulates that researchers need to take their moral obligations seriously and consider the harm that their work can have on people. While simply branding this as phrenology isn’t enough, the author mentions that identifying and highlighting the concerns will lead to more productive conversations.
How “truth decay” is harming America’s coronavirus recovery (Vox)
A very clear way to describe the problem plaguing the US response to the coronavirus, the phenomenon of truth decay is not something new but has happened many times in the past when trust in key institutions deteriorated and led to a diffused response to the crisis at hand, extending the recovery period beyond what would be necessary if there was a unified response. In the US, the calls for reopening the economy, following guidance on using personal protective equipment, and other recommendations is falling along partisan lines. The key factor causing this is how the facts and data are being presented differently to different audiences. While this epidemic might have been the perfect opportunity for bringing people together, because it affects different segments of society differently, it hasn’t been what everyone expected it to be.
At the core is the rampant disagreement between different factions on facts and data. This is exacerbated by the blurring of facts and opinions. In places like newsrooms and TV shows, there is an intermingling of the two which makes it harder for everyday consumers to discern fact from opinion. The volume of opinion has gone up compared to facts and people’s declining trust in public health authorities and other institutions is also aggravating the problem. Put briefly, people are having trouble finding the truth and don’t know where to go looking for it.
This is also the worst time to be losing trust in experts; with a plethora of information available online, people are feeling unnecessarily empowered that they have the right information, comparable to that of experts. Coupled with a penchant for confirming their own beliefs, there is little incentive for people to fact-check and refer to multiple sources of information. When different agencies come out with different recommendations and there are policy changes in the face of new information, something that is expected given that this is an evolving situation, people’s trust in these organizations and experts erodes further as they see them as flip-flopping and not knowing what is right. Ultimately, effective communication along with a rebuilding of trust will be necessary if we’re to emerge from this crisis soon and restore some sense of normalcy.
Too Big a Word (Data & Society)
Ethics in the context of technology carries a lot of weight, especially because the people who are defining what it means will influence the kinds of interventions that will be implemented and the consequences that follow. Given that technology like AI is used in high-stakes situations, this becomes even more important and we need to ask questions about the people who take this role within technology organizations, how they take corporate and public values and turn them into tangible outcomes through rigorous processes, and what regulatory measures are required beyond these corporate and public values to ensure that ethics are followed in the design, development and deployment of these technologies.
Ethics owners, the broad term for people who are responsible for this within organizations have a vast panel of responsibilities including communication between the ethics review committees and product design teams, aligning the recommendations with the corporate and public values, making sure that legal compliance is met and communicating externally about the processes that are being adopted and their efficacy. Ethical is a polysemous word in that it can refer to process, outcomes, and values. The process refers to the internal procedures that are adopted by the firm to guide decision making on product/service design and development choices. The values aspect refers to the value set that is both adopted by the organization and those of the public within which the product/service might be deployed. This can include values such as transparency, equity, fairness, privacy, among others. The outcomes refer to desirable properties in the outputs from the system such as equalized odds across demographics and other fairness metrics.
In the best case, inside a technology company, there are robust and well-managed processes that are aligned with collaboratively-determined ethical outcomes that achieve the community’s and organization’s ethical values. From the outside, this takes on the meaning of finding mechanisms to hold the firms accountable for the decisions that they take. Further expanding on the polysemous meanings of ethics, it can be put into four categories for the discussion here: moral justice, corporate values, legal risk, and compliance. Corporate values set the context for the rest of the meanings and provide guidance when tradeoffs need to be made in product/service design. They also help to shape the internal culture which can have an impact on the degree of adherence to the values. Legal risk’s overlap with ethics is fairly new whereas compliance is mainly concerned with the minimization of exposure to being sued and public reputation harm.
Using some of the framing here, the accolades, critiques, and calls to action can be structured more effectively to evoke substantive responses rather than being diffused in the energies dedicated to these efforts.
Be a Data Custodian, Not a Data Owner (Harvard Business Review)
Framing the metaphor of “data is the new oil” in a different light, this article gives some practical tips on how organizations can reframe their thinking and relationship with customer data so that they take on the role of being a data custodian rather than owners of the personal data of their customers. This is put forth with the acknowledgement that customers’ personal data is something really valuable that brings business upsides but it needs to be handled with care in the sense that the organization should act as a custodian that is taking care of the data rather than exploiting it for value without consent and the best interests of the customer at heart. Privacy breaches that can compromise this data not only lead to fines under legislation like the GDPR, but also remind us that this is not just data but details of a real human being.
As a first step, creating a data accountability report that documents how many times personal data was accessed by various employees and departments will serve to highlight and provide incentives for them to change behaviour when they see that some others might be achieving their job functions without the need to access as much information. Secondly, celebrating those that can make do with minimal access will also encourage this behaviour change, all being done without judgement or blame but more so as an encouragement tool. Pairing employees that need to access personal data for various reasons will help to build accountability and discourage intentional misuse of data and potential accidents that can lead to leaks of personal data.
Lastly, an internal privacy committee composed of people across job functions and diverse life experiences that monitors organization-wide private data use and provides guidance on improving data use through practical recommendations is another step that will move the conversation of the organization from data entitlement to data custodianship. Ultimately, this will be a market advantage that will create more trust with customers and increase business bottom line going into the future.
Beware of these futuristic background checks (Vox)
An increase in demand for workers for various delivery services and other gig work has accelerated the adoption of vetting technology like those that are used to do background checks during the hiring process. But, a variety of glitches in the system such as sourcing out-of-date information to make inferences, a lack of redressal mechanisms to make corrections, among others has exposed the flaws in an overreliance on automated systems especially in places where important decisions need to be made that can have a significant impact on a person’s life such as employment. Checkr, the company that is profiled in this article claims to use AI to scan resumes, compare criminal records, analyze social media accounts, and examine facial expressions during the interview process. During a pandemic, when organizations are short-staffed and need to make rapid decisions, Checkr offers a way to streamline the process, but this comes at a cost. Two supposed benefits that they offer are that they are able to assess a match between the criminal record and the person being actually concerned, something that can especially be fraught with errors in cases where the person has a common name. Secondly, they are also able to correlate and resolve discrepancies in the different terms that may be used for crimes across different jurisdictions.
A person spoke about his experience with another company that did these AI-powered background checks utilizing his public social media information and bucketed some of his activity into categories that were too coarse and unrepresentative of his behaviour, especially when such automated judgements are made without a recourse to correct, this can negatively affect the prospects of being hired. Another point brought up in the article is that social media companies might themselves be unwilling to tolerate scraping of their users’ data to do this sort of vetting which against their terms of use for access to the APIs. Borrowing from the credit reporting world, the Fair Credit Reporting Act in the US offers some insights when it mentions that people need to be provided with a recourse to correct information that is used about them in making a decision and that due consent needs to be obtained prior to utilizing such tools to do a background check. Though it doesn’t ask for any guarantees of a favorable outcome post a re-evaluation, at least it does offer the individual a bit more agency and control over the process.
From the archives:
Here’s an article from our blogs that we think is worth another look:
Computers, Creativity and Copyright: Autonomous Robot’s Status, Authorship, and Outdated Copyright Laws by Tess Buckley (Philosophy & International Development, McGill University)
This paper will argue that a robot has a degree of autonomy. It will then be problematized that an autonomous robot is capable of originality in its creations. Autonomous robots could be considered creative and would therefore be contenders for copyrights to creations. The authorship of AI’s creations will be discussed in relation to the multiplayer model. The current framework of copyright laws does not accommodate non-human authorship. It will be argued that copyright laws must be adjusted to protect AI as creators.
Guest contributions:
What has been published about ethical and social science considerations regarding the pandemic outbreak response efforts? by Bogdana Rakova, Research Fellow at Partnership on AI, Data Scientist at Accenture
This summary introduces the results from preliminary analysis of the CORD-19 research dataset and aims to investigate the ethical and social science considerations regarding pandemic outbreak response efforts. In particular, we identify the research articles in the dataset which discuss the potential barriers and enablers for the uptake of public health measures for prevention and control.
If you’ve got an informed opinion on the impact of AI on society, consider writing a guest post for our community — just send your pitch to support@montrealethics.ai. You can pitch us an idea before you write, or a completed draft.
Events:
As a part of our public competence building efforts, we host events frequently spanning different subjects as it relates to building responsible AI systems, you can see a complete list here: https://montrealethics.ai/meetup
We’ve got 2 events lined up, one each week on the following topics, for events where we have a second edition, we’ll be utilizing insights from the first session to dive deeper, so we encourage you to participate in both (though you can just participate in either, we welcome fresh insights too!)
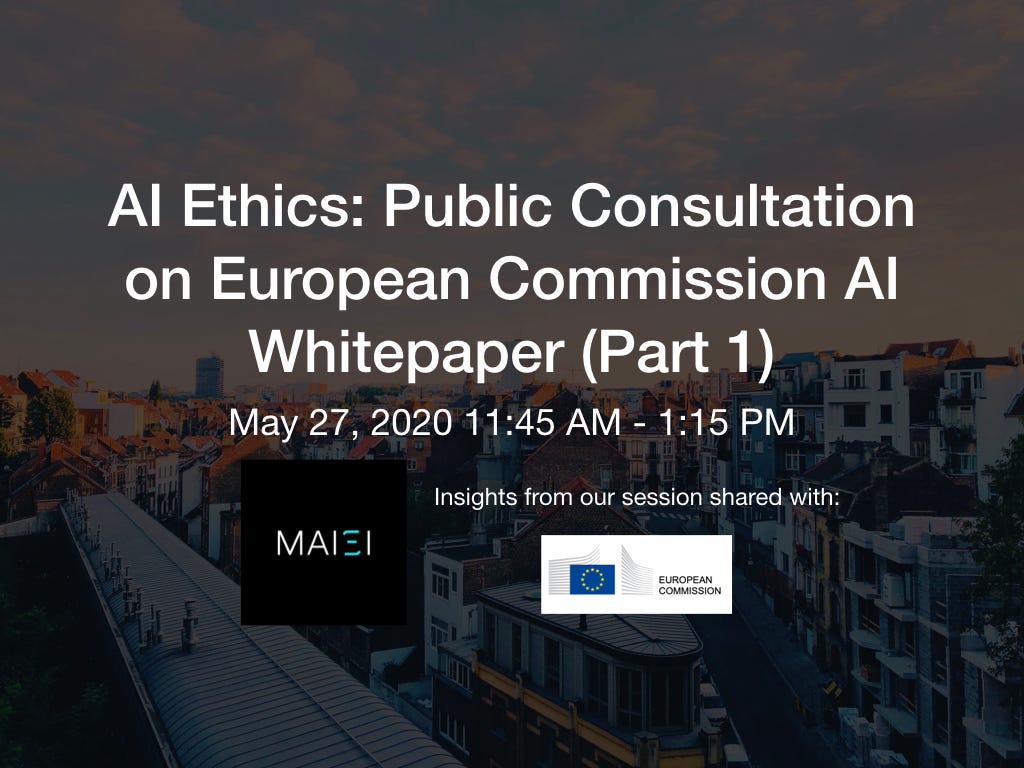
AI Ethics: Public Consultation on European Commission AI Whitepaper (Part 1)
May 27, 2020 11:45 AM -1:15 PM Online
AI Ethics: Public Consultation on European Commission AI Whitepaper (Part 2)
June 3, 2020 11:45 AM -1:15 PM Online
You can find all the details on the event page, please make sure to register as we have limited spots (because of the online hosting solution).
From elsewhere on the web:
Things from our network and more that we found interesting and worth your time.
We’ve highlighted the work from Jack Clark at OpenAI in the past and wanted to resurface that for our readers - take a look through his newsletter ImportAI where he dives deep into technical research that came out in the past week acting as a strong signal amidst all the noise in the field, you can subscribe here!
Signing off for this week, we look forward to it again in a week! If you enjoyed this and know someone else that can benefit from this newsletter, please share it with them!
If you have feedback for this newsletter or think there is an interesting piece of research, development or event that we missed, please feel free to email us at support@montrealethics.ai
If someone has forwarded this to you and you like what you read, you can subscribe to receive this weekly newsletter by clicking below