AI Ethics #13 : The State of AI Ethics quarterly report and more ...
Our thirteenth weekly edition covering research and news in the world of AI Ethics
Welcome to the thirteenth edition of our weekly newsletter that will help you navigate the fast changing world of AI Ethics! Every week we dive into research papers that caught our eye, sharing a summary of those with you and presenting our thoughts on how it links with other work in the research landscape. We also share brief thoughts on interesting articles and developments in the field. More about us on: https://montrealethics.ai/about/
If someone has forwarded this to you and you want to get one delivered to you every week, you can subscribe to receive this newsletter by clicking below
State of AI Ethics June 2020:
We are interrupting our normal programming to bring to you this special report on the State of AI Ethics for this past quarter!
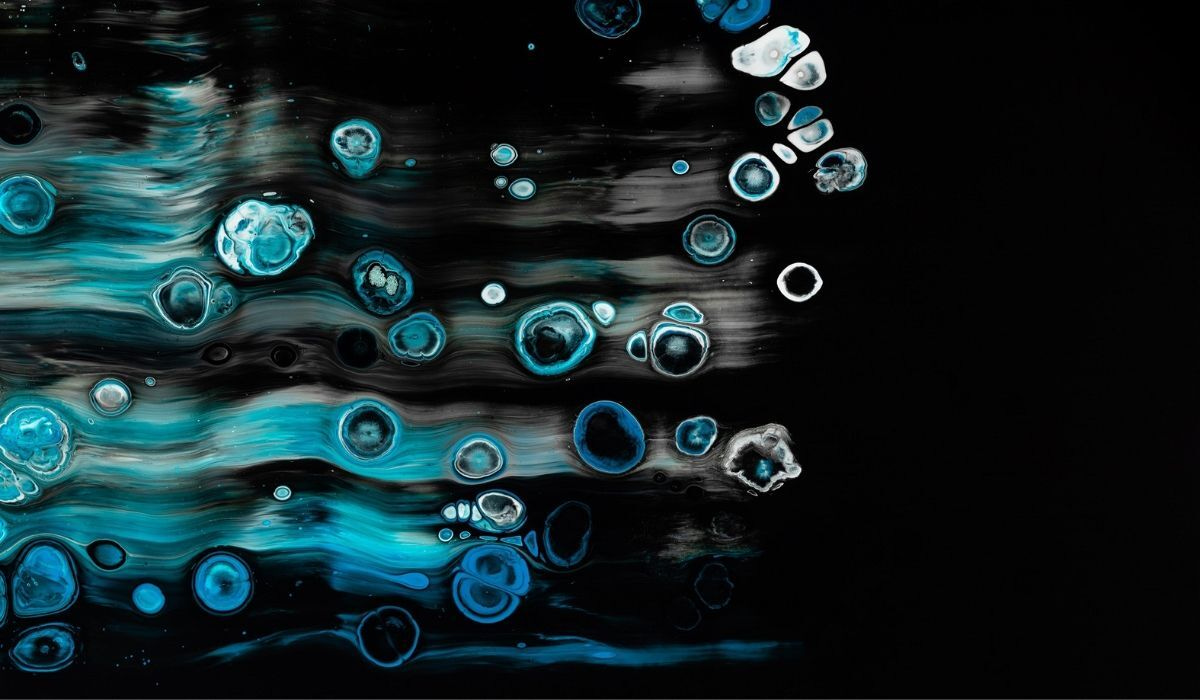
These past few months have been especially challenging, and the deployment of technology in ways hitherto untested at an unrivalled pace has left the internet and technology watchers aghast. Artificial intelligence has become the byword for technological progress and is being used in everything from helping us combat the COVID-19 pandemic to nudging our attention in different directions as we all spend increasingly larger amounts of time online.
It has never been more important that we keep a sharp eye out on the
development of this field and how it is shaping our society and interactions with each other. With this inaugural edition of the State of AI Ethics we hope to bring forward the most important developments that caught our attention at the Montreal AI Ethics Institute this past quarter. Our goal is to help you navigate this ever-evolving field swiftly and allow you and your organization to make informed decisions.
This pulse-check for the state of discourse, research, and development is geared towards researchers and practitioners alike who are making decisions on behalf of their organizations in considering the societal impacts of AI-enabled solutions.
We cover a wide set of areas in this report spanning Agency and Responsibility, Security and Risk, Disinformation, Jobs and Labor, the Future of AI Ethics, and more. Our staff has worked tirelessly over the past quarter surfacing signal from the noise so that you are equipped with the right tools and knowledge to confidently tread this complex yet consequential domain.
We hope you will enjoy this report and if you find it useful, please do share it with your colleagues and networks!
We have launched some new initiatives at MAIEI that are aimed at increasing scientific diversity, if you are not already a part of our Slack community this is a great time to join in!
In article summaries, we take a look at how no one reads privacy policies and what can be done about that, how Facebook groups can end up creating echo chambers, what can be done to improve IoT security, how humans working behind the AI curtain make faux automation, responsibility allocation when automated systems fail, and how complex workflows in science lead to a reproducibility crisis.
Our learning communities continue to receive an overwhelming response! Thank you everyone!
We operate on the open learning concept where we have a collaborative syllabus on each of the focus areas and meet every two weeks to learn from our peers. You can fill out this form to receive an invite!
Hoping you stay safe and healthy and looking forward to seeing you at our upcoming public consultation sessions (virtually!) and our learning communities! Enjoy this week’s content!
Articles:
Let’s look at highlights of some recent articles that we found interesting at MAIEI:
Nobody reads privacy policies. This senator wants lawmakers to stop pretending we do. (Washington Post)
Given that there isn’t a consumer privacy law that applies across the board, the proposal highlighted in this article mentions how there is a strong need for recognizing that consent based models which try to imbue agency onto users actually place undue burden on them. Specifically, it is well documented that the average person would require many days worth of time to parse through and comprehend meaningfully the various privacy policies as they relate to the different products and services that they use in their everyday life. In this case, people tend to just click through the “I accept” button without giving it much thought as to how their data is used and who has access to it. Informed consent doesn’t mean much, even when thinking about progressive disclosure models that purport to build up an understanding on the part of the user. The key problem lies in the allocation of burden and this proposed bill rightly shifts the burden away from the consumer onto the platform companies that tend to benefit enormously from the users’ private data, compared to the miniscule benefits that are offered in return to the users in terms of free service.
This shift also creates an impetus for companies to explore novel business models that don’t solely rely on extractive practices of selling users’ personal data for targeted advertising. Sen. Brown points out that the inspiration for this came from the Equifax data breach where the American public was caught off guard in that they didn’t even know what Equifax was, yet their personal information as held by Equifax had been compromised.
While the bill proposes to prohibit the use of data to generate targeted ads, contextual ads, such as the ones from Google in their search products would pass muster under the proposed requirements. Ultimately, as a researcher opines in the article, we must take incremental steps in privacy law in this context rather than aiming for something perfect which might not be something that will get passed due to differing views in the law-making bodies on this subject.
Facebook Groups Are Destroying America (Wired)
Facebook groups are a great place to meet like-minded people, but in this case birds of a feather flocking together might be a bad idea, especially as it relates to the spreading of disinformation. We have covered this topic extensively in the past, one of the things that we appreciated about this article was how closed groups that congregate around special topics can become sources for amplifying disinformation. A concern that we’ve been studying at the Montreal AI Ethics Institute as it relates to disinformation is how these closed groups tend to become a hindrance for researchers who are trying to study the effects that they have on spreading disinformation. Just as is the case with P2P messaging apps where researchers don’t have access to the larger network effects on how disinformation is propagating through the network, closed groups also hamper the ability of researchers to study more deeply that these closed groups have on Facebook. One of the suggestions made by the researchers quoted in the article asks for Facebook to change the privacy settings of the group to public once it exceeds a certain size so that researchers are better able to study them.
By working through some of the groups as members, the researchers were able to see how bad actors created Sybil-styled personas that tailored the messaging to the group and helped to propagate disinformation through gullible members of that group who believe the information from the group to be coming from a trusted and safe space because of the closed nature of the group. Another suggestion made by the researchers is to have the platforms provide more transparency on the ownership and management of the groups so that users are able to see patterns when there might be bad actors who are using several groups to coordinate and amplify their disinformation campaigns. While Facebook has taken steps to stop providing other suggested groups related to conspiracy and other topics, they still show up in the Discover tab. Researchers suggest that an approach to mitigate this would be to bar them from appearing at all so that users would have to search from them explicitly which would limit the amplification of these disinformation campaigns.
IoT Security Is a Mess. Privacy 'Nutrition' Labels Could Help (Wired)
From DEFCON 2017, in one of the talks that Abhishek attended, someone mentioned that you can’t spell “idiot” without “iot”, what the panelists and speakers were referring to were the rampant security and privacy challenges that plague IoT systems, especially due to their connected nature and their limited processing capabilities which hampers their ability to have extensive security mechanisms in place. Analogous to other work in the machine learning domain, for example, the dataset nutrition labels, the researchers quoted in this article created a new set of privacy nutrition labels that elucidate the security posture of the IoT system, detailing things like the length of support from the manufacturer, the updates schedule, what data is collected, how is it used, etc.
One of the things that immediately caught our attention was the focus on making the labels accessible to the everyday consumer and supplementing it with functionality that makes these labels machine-readable and interoperable. This is very strongly aligned with the work at the Montreal AI Ethics Institute in the domain of machine learning security being done by the research staff Erick Galinkin and Abhishek Gupta. As pointed out in the article, one of the benefits of this approach is that consumers will be able to search for this machine-readable information in a manner that allows them to make informed purchasing decisions along the privacy and security features of the product rather than just other technical considerations.
One of the other considerations is to standardize the bill of materials used in a software product so that there is transparency in terms of the open-source and other libraries that underpin the system so that consumers and external researchers can have clarity on potential security and privacy threats as it relates to the system by looking at these labels. While we are far away from widespread adoption, something that is evident is the need to create something that is intelligible to users that empowers them to make informed decisions.
The Humans Working Behind the AI Curtain (Harvard Business Review)
Just as in the Wizard of Oz and Dorothy peeking behind the curtain to discover strings being pulled and shattering an illusion, a lot of AI companies who are trying to latch onto the hype surrounding AI, as covered before in our newsletters, utilize low-cost human workers who are asked to step into smoothen out the rough edges of AI systems. This labor is often in unregulated environments where there is limited training and guidance provided to the workers. Especially when they are called upon to moderate disturbing online content, review posts that have been flagged for violating community guidelines, and many other tasks that help to keep the internet from being overwhelmed with spam and undesirable content.
One of the discouraging things about the labor that backs this faux automation is that they are rarely compensated in a fair manner and they are hidden in the shadows so that companies can maintain the aura that they have well-functioning automation in place, when it is more akin to a human-backed fly-by-the-wire system that helps to smoothen out the problems that occur when the AI system doesn’t function as intended or is non-existent to begin with. As AI is rolled out more widely, the author points out that this is the paradox of automation’s last mile, giving a nod to the last-mile problem faced by delivery companies where the last-mile to deliver the product from the source to destination is the most challenging and often requires resorting to highly labor-intensive and manual processes.
Given the severe lack in training, work environment regulations, protocols for regulating content, there is a high risk of uneven enforcement of the policies of different platforms which harms the experience of users. This harm often tends to fall disproportionately on those who are already marginalized further stripping them of their ability to express themselves freely online. We need to make a strong call for the companies to be transparent about their labor practices so that consumers are able to effectively evaluate them for unfair practices and use their attention and dollars to steer the market towards better social outcomes.
Who Is Responsible When Autonomous Systems Fail? (Center for International Governance Innovation)
In the infamous case of the Uber self-driving vehicle accident in 2018 that brought forth a dark cloud over testing of autonomous vehicles (AVs) on public roads for a while, it was interesting to note that they were back on the streets a few months later with almost no reprimands. The safety drive in the AV though still continues to grapple with legal action. The allocation of responsibility in this context has been uneven, whereby the manufacturers of the vehicle, the developer of the software for the AV, and the state of Arizona that allowed for testing to take place held no responsibility in the accident.
Often the human-in-the-loop is pushed forth as a safety mechanism that is going to be the failsafe in case the automation doesn’t work as intended, yet one has to carefully analyze the position of the human in that loop, especially as it relates to whether they are empowered or disempowered to take action. We can end up wrongly estimating the capabilities of both the humans and the machines such that the gap that is meant to be covered in case of failures in automation are in fact left even more widely exposed. Specifically, there is an argument to be made that automation can help to fill over the smaller errors but leave room for even larger errors to occur, especially as the humans who are supposed to be in the loop atrophy in skills and become tokenized.
The author points out the concept of the moral crumple zone, drawing from the examples in the aviation industry where there is an increasing amount of automation but when it comes to allocating blame for things going wrong, that is put squarely on the shoulders of the pilots / the nearest human around with little to no scrutiny on the automated systems themselves. Any of these systems always operate in a complicated environment where they have a variety of feedback loops with the humans that are a part of the system and disregarding those loops and the interactions between the humans and the machines takes a very narrow look at the problems. The term crumple zone comes from vehicle parts that are meant to absorb the bulk of the damage and impact during an accident to protect the human. In the case of highly complex and automated systems, the humans become the scapegoat or crumple zone for taking on the moral liabilities when it comes to the failure of these systems.
The author also points out the concept of the irony of automation whereby automation doesn’t fully eliminate human errors, it just creates opportunities for new kinds of errors. This also brings to light the handoff problem whereby we still don’t have effective ways to transfer control from a machine to a human quickly and meaningfully in the case of failures on the part of the automated systems. As a closing argument, it is important to consider, as the author points out, that we don’t doom these essential workers who form the human infrastructure to give us the illusion of automation running smoothly to become sacrificial workers without adequate protections. Governance in AI needs to address these challenges as a priority before more people end up in a situation where they take on a disproportionate burden of the fallout from the failure of these systems.
Complex data workflows contribute to reproducibility crisis in science, Stanford scientists say (Stanford)
The field of machine learning is no stranger to complex data workflows. It relies on crunching large amounts of data that are taken from their raw form and carefully processed and transformed by engineers and data scientists to arrive into a format and shape that is amenable to being processed by machine learning algorithms. Reproducibility has been a huge concern off late in the field and this experiment mentioned in the article highlights this in the context of life sciences where research teams from across the world were provided with the same dataset, the same hypotheses to test, and they came up with differing results on 5 out of the 9 hypotheses.
Diving deeper into the issues that gave rise to these discrepancies, the investigators behind the study surfaced that there was a lack of consistency in how the data was preprocessed, what libraries were used to do this transformation, what activations and thresholds were used in analyzing the fMRI data among other differences which ultimately led to differences in the final results. One of core tenets for good science revolves around being able to take the data, methods, and other information and reproduce the results independently. Barring which we end up just relying on the word of the scientists who originally conducted the experiment for the validity of the results.
While this challenge has plagued medicine, psychology, and other fields, especially in the domain of AI where there are a lot of resources being invested and we have a new generation of researchers utilizing fora like arXiv to grab the latest research and build from it, we must be cautious, accountable, and transparent in the conduction of our experiments and research so that we do right by the research community around us.
From the archives:
Here’s an article from our blogs that we think is worth another look:
Apps Gone Rogue: Maintaining Personal Privacy in an Epidemic
With a rising number of cases worldwide of COVID-19 and extensive measures being taken across the world to minimize the spread and mitigate economic harm and continuity to our way of life. Yet, some measures are creeping up on invading the privacy of people and creating real possible harms in how the collected data is used and managed. The paper by Raskar et al. presents some of the contact tracing solutions that are being used in places around the world and their associated risks. They also share information on an open-source solution called Private Kit : Safe Paths that is a privacy preserving way of doing contact tracing. While there are very clear benefits in containing the spread of the epidemic, the privacy and other social harms arising as a consequence of the use of such technology need to be weighed and judged in line with the culture and values of the society in which it is being used. It is also important to ensure the inclusivity of the solutions so developed because often those with minimal access to technology are the most vulnerable to the negative impacts of the epidemic. Ultimately, it is critical to weigh the tradeoffs in deploying contact tracing technology compared to the intended and unintended harms that can arise with its use.
To delve deeper, read the full article here.
Guest contributions:
If you’ve got an informed opinion on the impact of AI on society, consider writing a guest post for our community — just send your pitch to support@montrealethics.ai. You can pitch us an idea before you write, or a completed draft.
Machine learning retrospectives, surveys, and analyses:
The ICML Retrospectives Workshop is about reflecting on machine learning research. This workshop builds upon the NeurIPS 2019 Retrospectives Workshop and encourage the exploration of a new kind of scientific publication, called retrospectives.
The ultimate goal of retrospectives is to make research more human.
That means getting researchers to write papers like how they’d talk to a friend. It means making research transparent and accessible for everyone. And it means creating an environment in the machine learning community where it’s okay to make mistakes. After all, in the scientific endeavor, we’re all on the same team.
For more information on how to submit, please follow this link!
Events:
As a part of our public competence building efforts, we host events frequently spanning different subjects as it relates to building responsible AI systems, you can see a complete list here: https://montrealethics.ai/meetup
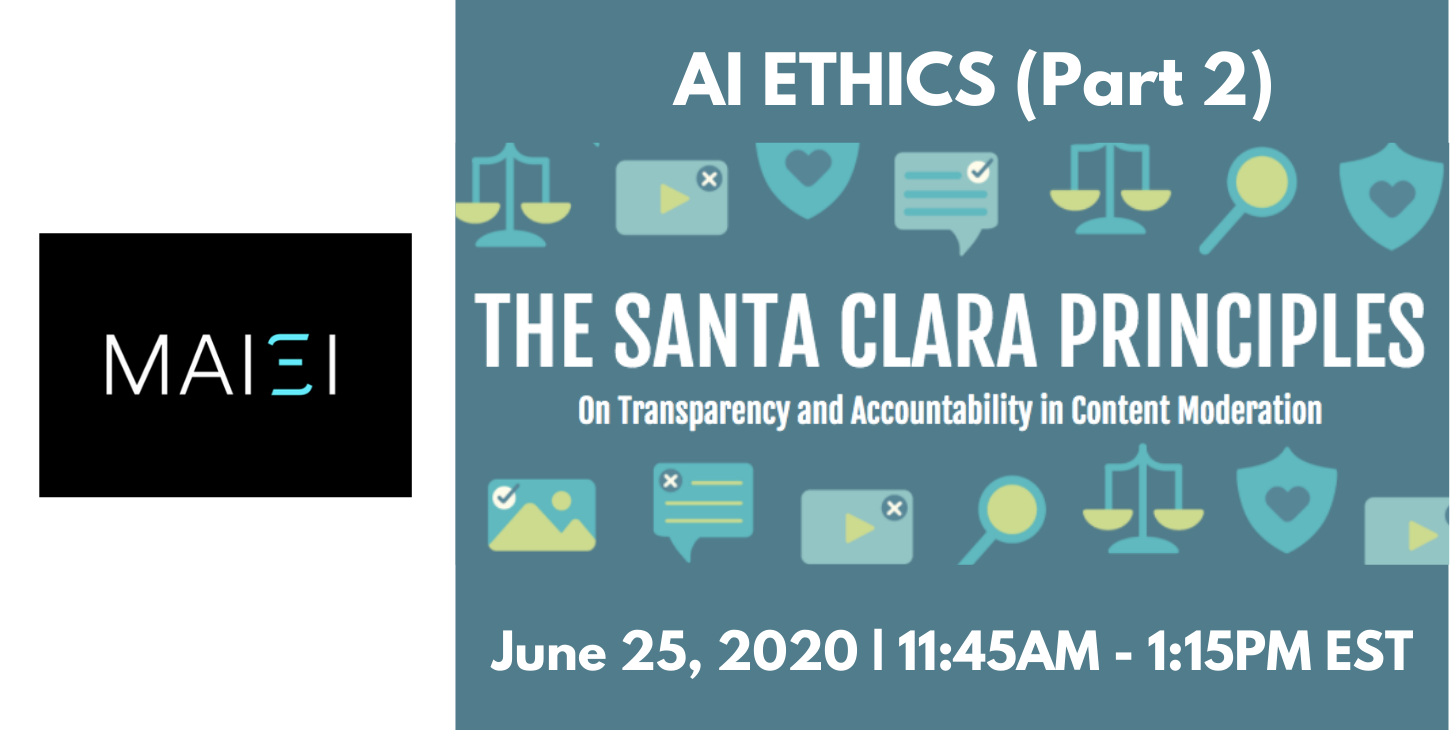
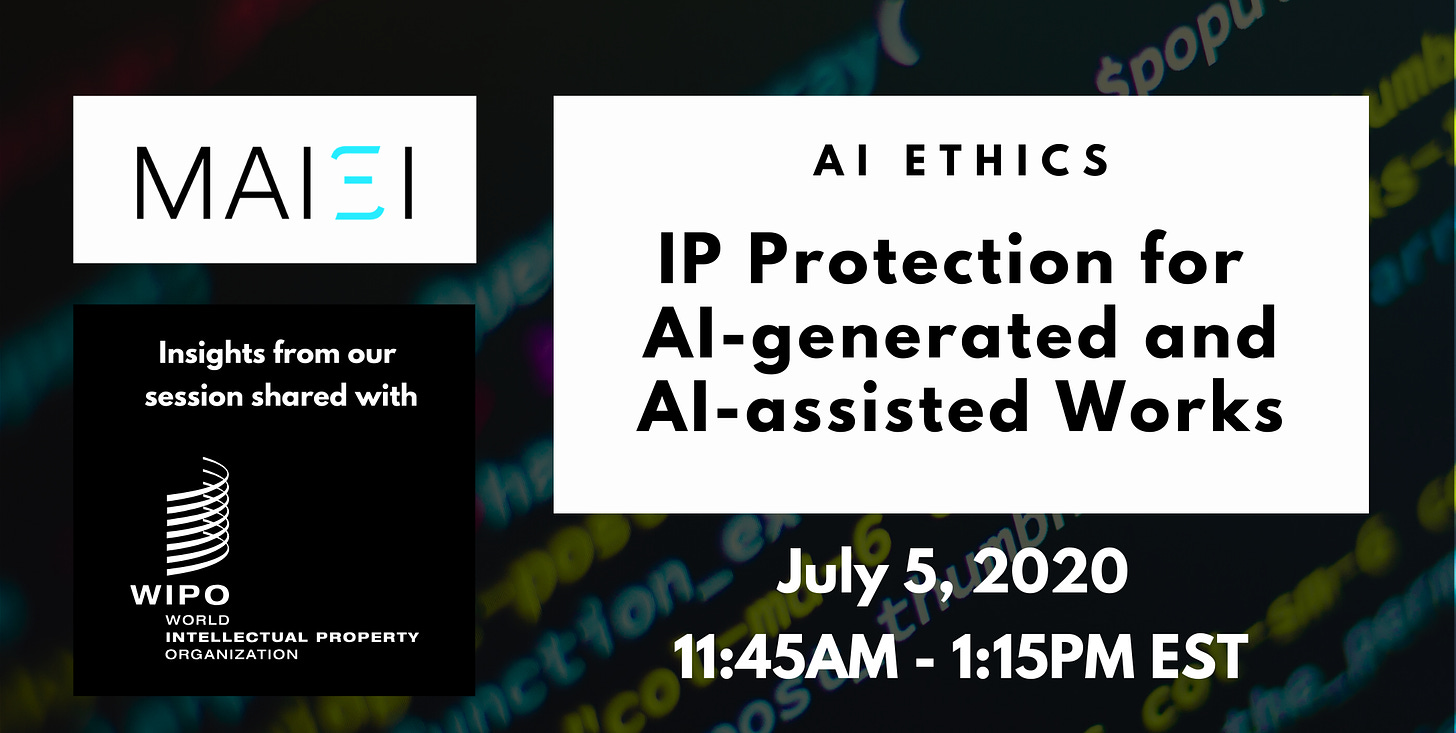
We’ve got 2 events lined up, one each week on the following topics, for events where we have a second edition, we’ll be utilizing insights from the first session to dive deeper, so we encourage you to participate in both (though you can just participate in either, we welcome fresh insights too!)
AI Ethics: Santa Clara Principles for Content Moderation (Part 2)
June 25, 11:45 AM - 1:15 PM ET (Online)
AI Ethics: IP Protection for AI-generated and AI-assisted works
July 5, 11:45 AM - 1:15 PM ET (Online)
You can find all the details on the event page, please make sure to register as we have limited spots (because of the online hosting solution).
From elsewhere on the web:
Things from our network and more that we found interesting and worth your time.
Response to Mila’s Proposal for a Contact Tracing App
“COVI” is the name of a recent contact tracing app developed by Mila and was proposed to help combat COVID-19 in Canada. The app was designed to inform each individual of their relative risk of being infected with the virus, which Mila claimed would empower citizens to make informed decisions about their movement and allow for a data-driven approach to public health policy; all the while ensuring data is safeguarded from governments, companies, and individuals.
This article will provide a critical response to Mila’s COVI White Paper. Specifically, this article will discuss: the extent to which diversity has been considered in the design of the app, assumptions surrounding users’ interaction with the app and the app’s utility, as well as unanswered questions surrounding transparency, accountability, and security.
We see this as an opportunity to supplement the excellent risk analysis done by the COVI team to surface insights that can be applied to other contact- and proximity-tracing apps that are being developed and deployed across the world. Our hope is that, through a meaningful dialogue, we can ultimately help organizations develop better solutions that respect the fundamental rights and values of the communities these solutions are meant to serve.
To delve deeper, please read the entire report here.
BANFF FORUM // Public Health Data, Technology, and Privacy
Our founder Abhishek Gupta will be speaking at the Banff Forum Virtual Speaker Series! Most Canadians leave a trail of data exhaust behind them in their daily online activity, yet there are real privacy concerns to consider when adopting digital tracing technology. Is the data to be gained useful enough to warrant the loss of privacy? Is economic recovery more pressing than continuing to flatten the curve?
Join us as we consider this complex issue through the lens of responsive public policy, ethical technology development, and public health impact.
Canadian Society for Ecological Economics
Our staff researcher Camylle Lanteigne is presenting some of her research work on SECure: A Social and Environmental Certificate for AI Systems at the Canadian Society for Ecological Economics which was also recently featured by VentureBeat in the article Researchers propose framework to measure AI’s social and environmental impact
Signing off for this week, we look forward to it again in a week! If you enjoyed this and know someone else that can benefit from this newsletter, please share it with them!
If you have feedback for this newsletter or think there is an interesting piece of research, development or event that we missed, please feel free to email us at support@montrealethics.ai
If someone has forwarded this to you and you like what you read, you can subscribe to receive this weekly newsletter by clicking below