
AI Ethics #18: Living Dictionary, red-teaming AI systems, computing in social change, energy latency attacks, future of work with software automation and more ...
Ethical practices blueprints, tech ethics guidelines with workers in mind, memes and the pandemic, voting misinformation, proving war crimes in courts using AI, and more from the world of AI Ethics!
Welcome to the eighteenth edition of our weekly newsletter that will help you navigate the fast changing world of AI Ethics! Every week we dive into research papers that caught our eye, sharing a summary of those with you and presenting our thoughts on how it links with other work in the research landscape. We also share brief thoughts on interesting articles and developments in the field. More about us on: https://montrealethics.ai/about/
If someone has forwarded this to you and you want to get one delivered to you every week, you can subscribe to receive this newsletter by clicking below:
Summary of the content this week:
In research summaries this week, we look at AI in context and the labor of integrating new technologies, roles for computing in social change, decision points in AI governance, energy latency attacks on neural networks, software automation and the future of work, troubling trends in machine learning scholarship, and algorithms deciding the future of legal decisions.
In article summaries this week, steps for drafting an ethical practices blueprint, memes, the pandemic, and new tactics in information warfare, rewriting ethics guides with workers in mind, using AI to prove war crimes in court, hacking and red-teaming your own AI systems, and voting misinformation flourishing on Facebook.
MAIEI Community Initiatives:
Our learning communities and the Co-Create program continue to receive an overwhelming response! Thank you everyone!
We operate on the open learning concept where we have a collaborative syllabus on each of the focus areas and meet every two weeks to learn from our peers. You can fill out this form to receive an invite!
MAIEI Living Dictionary:
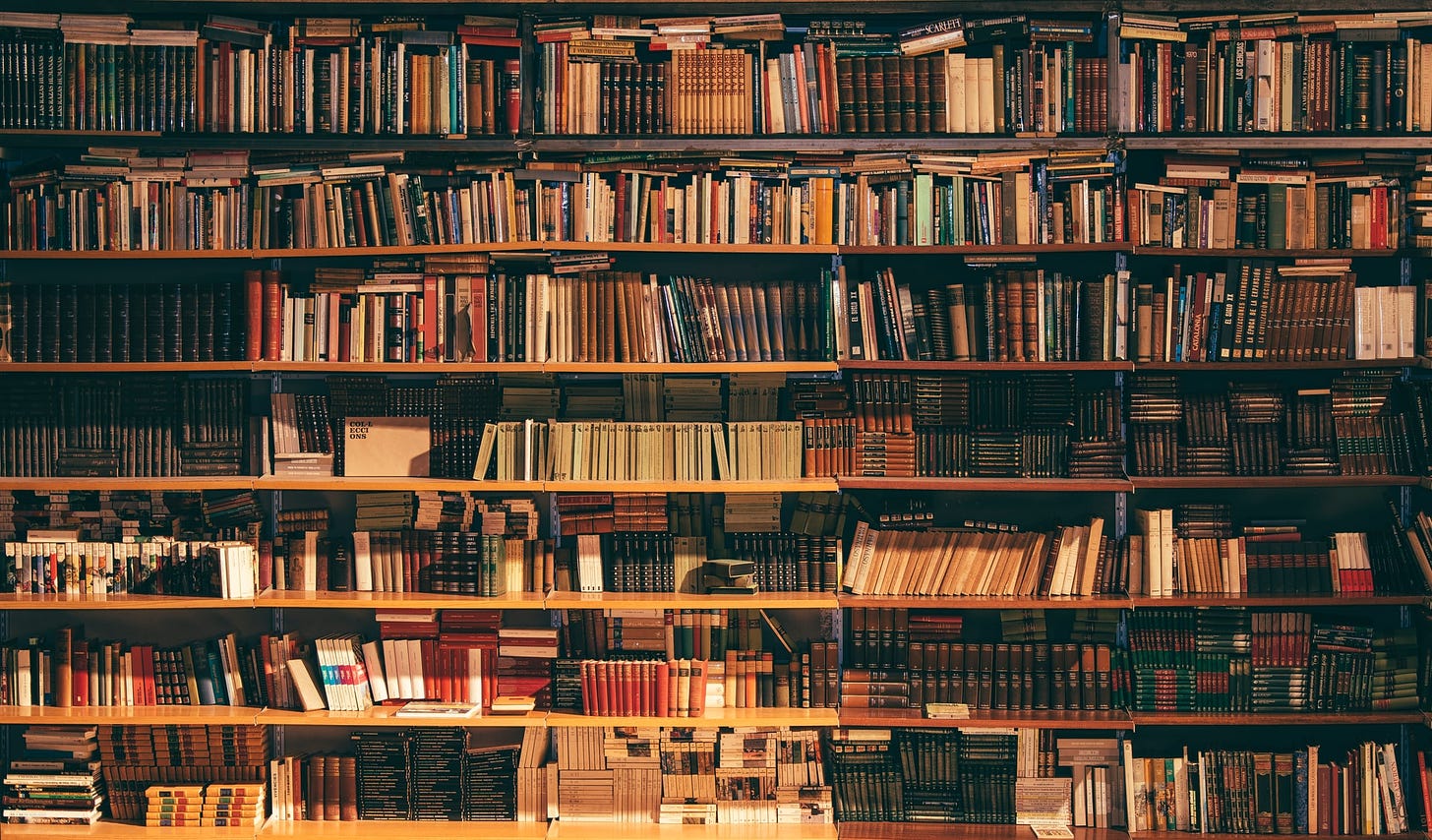
Photo by Alfons Morales on Unsplash
The Living Dictionary was designed by the Montreal AI Ethics Institute to inspire and empower you to engage more deeply in the field of AI Ethics. With technical computer science and social science terms explained in plain language, the Living Dictionary aims to make the field of AI ethics more accessible, no prior knowledge necessary! We hope that the Living Dictionary will encourage you to join us in shaping the trajectory of ethical, safe and inclusive AI development.
Explore the AI Ethics Living Dictionary!
MAIEI Serendipity Space:
The first session was a great success and we encourage you to sign up for the next one!
This will be a 30 minutes session from 12:15 pm ET to 12:45 pm ET so bring your lunch (or tea/coffee)! Register here to get started!
Research:
Let's look at some highlights of research papers that caught our attention at MAIEI:
AI in Context: The Labor of Integrating New Technologies by Alexandra Mateescu and Madeleine Clare Elish
The rise of automation has created fears over the future of human labour, with claims by economists that more than 47% of American jobs will be rendered obsolete in 2030. The authors, contrary to these predictions, posit that AI systems will reconfigure work practices rather than replace workers. Through using the case study of family-owned farms and retail grocery technologies, they unveil new evidence and a framework to examine and predict the near-term impacts of automated and AI systems. Their findings reveal the recurrent tendency to obscure the human labour needed to integrate these technologies, with their adoption often requiring new skills, new routines and changes to the physical infrastructure. These unacknowledged and often uncompensated forms of labour will put precarious workers at greater financial risk than the firms and managers who control the design or use of AI technologies.
To delve deeper, read our full summary here.
Roles for Computing in Social Change by Rediet Abebe, Salon Barocas, Jon Kleinberg, Karen Levy, Manish Raghavan, and David G. Robinson
This paper highlights the increasing dissonance between computational and policy approaches to addressing social change. Specifically, it calls out how computational approaches are viewed as an exacerbating element to the social ills in society. But, the authors point out how computing might be utilized to focus and direct policymaking to better address social challenges. Specifically, they point to the use of technology as a medium of formalization of social challenges. This methodology brings forth the benefits of making explicit the inputs, outputs, and rules of the system which can create opportunities for intervention. It also has the benefit of translating high-level advocacy work into more concrete, on-the-ground action.
Computational approaches can also serve as a method for rebuttal: empowering stakeholders to question and contest design and development choices. They present an opportunity to shed new light on existing social issues, thus attracting more resources to redressal mechanisms. From a practitioner’s standpoint, computational approaches provide diagnostic abilities that are useful in producing metrics and outputs that showcase the extent of social problems. While such computational methods don’t absolve practitioners of their responsibilities, it provides them and other stakeholders requisite information in acting on levers that bring about change in the most efficacious manner possible.
To delve deeper, read our full summary here.
Decision Points in AI Governance by Jessica Cussins Newman
Newman embarks on the lonely and brave journey of investigating how to put AI governmental principles into action. To do this, 3 case studies are considered, ranging from ethics committees, publication norms and intergovernmental agreement. While all 3 of those aspects have their benefits, none of them are perfect, and Newman eloquently explains why. The challenges presented are numerous, but the way forward is visible, and that way is called practicality.
To delve deeper, read our full summary here.
Sponge Examples: Energy-Latency Attacks on Neural Networks by Shumailov, I., Zhao, Y., Bates, D., Papernot, N., Mullins, R., & Anderson, R
Energy use can also be used for nefarious purposes through sponge examples: attacks made on an ML model to drastically increase its energy consumption during inference. Sponge examples can make an ML model’s carbon emissions skyrocket, but they can also cause more immediate harm. Increased energy consumption can significantly decrease the availability of the model, increase latency and ultimately delay operations. More concretely, autonomous vehicles undergoing a sponge attack may be unable to perform operations fast enough due to this delay, causing a vehicle to fail to break in time, leading to a collision.To defend against an adversary exploiting a sponge example, the authors suggest 1) a cut-off threshold, where the total amount of energy consumed for one inference cannot be higher than a predetermined threshold, and 2) to address delays in real-time performance which could have deadly consequences in mission-critical situations, these systems must be designed to function properly even in worst-case performance scenarios and have a fail-safe mechanism.
To delve deeper, read our full summary here.
From our learning communities:
Research that we covered in the learning communities at MAIEI this week, summarized for ease of reading:
Working Algorithms: Software Automation and the Future of Work by Benjamin Shestakofsky
Fears surrounding automation and human labour have consumed economists, with the advance of “smart machines” threatening to trigger mass unemployment. Contrary to this perspective, there is the argument that new forms of labour and human-machine complementarity will contribute to the endurance of human labour. Through a 19-month participation-observation study at AllDone, a software startup in San Francisco, Shestakofsky gained first-hand insight into the relations between workers and technologies throughout three stages of corporate development. He documented how new complementarities continued to emerge between humans and software systems as the startup grew. Rather than solely relying on economic abstractions, daily observations can help identify to what extent software systems can operate autonomously and where they continue to require human assistance. Through the author’s micro-study, we can trace how companies, particularly startups, will need to adapt and be dynamic in the face of automation. Shestakofsky’s work can also point to larger macro-trends in the domestic and global division of labour.
To delve deeper, read our full summary here.
Articles:
Let’s look at highlights of some recent articles that we found interesting at MAIEI:
Four steps for drafting an ethical data practices blueprint (TechCrunch)
Prompting the need for having an ethical data science blueprint, the article starts by mentioning how a healthcare provider that relied on AI ended up prioritizing white patients over black patients. The article advocates for four different steps to building responsible AI within the organization. The first step is the integration of ethical AI practices within the existing work in the organization around the assessment of privacy risks and legal compliance. Leveraging existing bodies reduces friction in adoption. The second step is maintaining an appropriate level of transparency, which can be decided by a C-suite executive who takes on this role. They can analyze the ethics against business requirements and then articulate them to product managers who are responsible for implementing them in practice.
The importance of a robust and clear blueprint is that it leads to consistency in implementation rather than delegating decisions to individual data scientists. From a technical standpoint, since there are numerous definitions for fairness, a body of experts must be consulted to select those that are most appropriate for the problem at hand.
Finally, education and training on data ethics will equip and empower front-line workers in making the right decisions in their everyday work and help them align with the ethical blueprint for the organization.
Memes, the pandemic and the new tactics of information warfare (C4ISRNET)
We have covered the subject of disinformation many times in this newsletter and our learning communities at the Montreal AI Ethics Institute. The pandemic has brought on new dimensions to this problem by equipping malicious actors with more tools that they can wield in their information operations. Researchers point out that adversaries don't even need to create new content, but merely amplify existing divisive content in a host country to achieve their aims. It has the benefit of leveraging contextual and cultural sensitivity that outsiders would never understand, thus making the material much more effective. Secondly, using what is called memetic warfare, adversaries use compact, visually-appealing content that has a quick, emotional punch to convey their message to their target audiences. Since memes are typically not signed by their creators, they evoke an emotional response, and are susceptible to being shared very widely and quickly.
State-backed adversaries also use this tactic to inflict harm and derail genuine efforts made by a nation towards building a positive society. They often play both sides of the debate, sowing confusion and eroding the trust that the public has in their governments. Finally, sometimes bureaucracy can become an internal enemy by slowing down responses to the spread of disinformation. By the time the disinformation gets debunked, the damage has taken place, and adversaries have succeeded in their goals.
An Ethics Guide for Tech Gets Rewritten With Workers in Mind (Wired)
Ethics needs to be discussed as early in the lifecycle of product development as possible. Akin to cybersecurity, the cost of doing so is orders of magnitudes less, and the efficacy much higher when following this pattern. So why is it that this doesn't happen often? This article talks about a new toolkit from the Omidyar Network that builds on their prior work. Specifically, this iteration focuses on mechanisms that empower developers and those who are on the ground, creating products and services to feel confident in bringing up these issues.
"The kit includes a field guide for navigating eight risk zones: surveillance, disinformation, exclusion, algorithmic bias, addiction, data control, bad actors, and outsize power." Absolutely in line with the work that we do at the Montreal AI Ethics Institute, we believe that there is a dire need for handy formulations that we can frictionlessly integrate into the design, development, and deployment phases of AI. While the unofficial mantra of Silicon Valley is to "move fast and break things," such tools might help to slow down this process and offer opportunities for reflection that can help incorporate ethics, safety, and inclusion in the systems that we build.
Human rights activists want to use AI to help prove war crimes in court (MIT Tech Review)
Just as is the case with content moderation that hurts the human agents who have to review it and are subsequently traumatized by it, analyzing footage from conflict zones to identify potential human rights violations poses similar challenges. Machine learning-enabled solutions that can automatically parse footage and identify different violations can spare humans from having to look at this kind of footage. However, and more importantly, these techniques have the benefit of being able to process orders of magnitude more content, which can be tremendously useful in making more substantive cases in international courts against authoritative regimes and other violators of human rights.
Given that instances of some kinds of prohibited weapons and techniques are rare, to aid the machine learning process, researchers are utilizing synthetic data crafted from limited information sets to supplement the process. What would have taken years for teams of humans working around the clock to analyze all the accumulated footage, the machine learning system can do in a few days. It makes the case made by humanitarian organizations that much more robust since they can demonstrate a systematic abuse of human rights by pulling out several examples through the automated analyses of the accumulated footage. It might just usher in a new era where more malicious actors are held liable for their war crimes, and help bring justice to those who don't have the resources to express their problems.
Facebook’s ‘Red Team’ Hacks Its Own AI Programs (Wired)
We are increasingly relying on content moderation by automated means because of the rise in the amount of content produced and the detrimental mental health effects faced by human content moderators. Given the current limitations of AI systems, we have settled into an adversarial setting between content moderation systems and malicious actors who try to slip content by the moderation system.
Facebook found that as they tried to curb nudity in their platform families, content creators came up with creative ways to elude censorship. When the team created new fixes to address those "attacks", they found that the creators came up with other novel ways of evading filters.
The CTO of Facebook mentioned that as AI systems become more integral to their production systems, it has become even more critical to ensure that they are robust against such circumvention attempts. In the same vein, to understand deepfakes in the wild and how to limit their spread, they launched the DeepFake Detection Challenge, which was an attempt at surfacing techniques that might be effective in countering the rising ubiquity of these tools. Building on the work of some academic researchers, it is crucial to develop tooling similar to the ones used in cybersecurity that can be integrated into existing developer workflows to enhance the robustness of AI systems. Research at the Montreal AI Ethics Institute by Abhishek Gupta and Erick Galinkin proposes new mechanisms to foster ubiquity in adoption.
“Outright Lies”: Voting Misinformation Flourishes on Facebook (ProPublica)
Voting is one of the fundamental pillars of democracy, which is why Facebook includes voting-related misinformation to be a category of its own whereby content that misrepresents voting violates their community standards and is liable to be taken down. With a major election coming up this year in the US, researchers have found that the platform is rife with misinformation. Many posts that violate their community standards are left unattended without any action. The platform has kicked the can down the road in terms of harsher clampdowns on misinformation on its platform. Some of the posts blended opinions and factual errors, outright lies about voting, or misleading claims about the reliability of different voting methods. While they are committed to providing accurate information about voting, they also commit to free speech rights, and both of these might conflict.
The platform has made the concession that they are considering banning political ads from the platform, but research has found that misinformation spreads more through posts and political ads largely contain factually correct information, thus limiting the impact of the ban on political ads in addressing the underlying issue. When misinformation spreads and even those who are skeptical of it see it proliferating the platform, there is a sense of disenfranchisement among those who are explicitly targeted, often people of color. Sowing distrust in the communities is an effective way to depress voter turnout and skew the results of the election. Slow action by contracted fact-checkers also gives enough spotlight to misinformation that it achieves its goals well before the content gets taken down.
From the archives:
Here’s an article from our blogs that we think is worth another look:
Troubling Trends in Machine Learning Scholarship by Zachary Lipton and Jacob Steinhardt
With the explosion of people working in the domain of ML and the prevalence of the use of preprint servers like arXiv, there are some troubling trends that have been identified by the authors of this paper when it comes to scholarship in ML. Specifically, the authors draw attention to some common issues that have become exacerbated in the field due to a thinning experienced reviewer pool who are increasingly burdened with larger numbers of papers to review and might need to default to checklist type of patterns in evaluating papers. A misalignment of incentives when it comes to communicating and expressing the results of the papers and the subject matter associated with them in terms that might draw the attention of investors and other entities who are not finely attuned to pick up flaws in scholarship. Lastly, a complacency in the face of progress whereby weak arguments are seen as acceptable in the face of strong quantitative and empirical evidence also makes this problem tough to handle.
To delve deeper, read the full article here.
Guest contributions:
Algorithms Deciding the Future of Legal Decisions by Brooke Criswell
Artificial intelligence (AI) is everywhere and in every industry. Technological advances can enhance people’s everyday lives and produce some amazing outcomes at rapid speed. However, AI also has the potential to be biased and harm individuals, depending on the algorithms’ usage and design. Many industries including the judicial system are now incorporating AI into their decision making. The claim is that using machines takes the biases that humans have, out of the equation, and so the decisions have to be objective. However, it has been shown time and time again that it is not true (O’Neil, 2017). This paper explores how artificial intelligence is being used in the court room for predicting criminal behavior, length of sentences, and determining who is likely to recommit a crime. Data scientists are also being hired within the judicial system to manage these machines; however, media psychologists are better fit and need to be involved in the process. Data scientists are not trained in human cognition and human behavior. In fact, before algorithmic techniques, the risk was assessed clinically by psychologists.
To delve deeper, read the full article here.
If you’ve got an informed opinion on the impact of AI on society, consider writing a guest post for our community — just send your pitch to support@montrealethics.ai. You can pitch us an idea before you write, or a completed draft.
Events:
As a part of our public competence building efforts, we host events frequently spanning different subjects as it relates to building responsible AI systems, you can see a complete list here: https://montrealethics.ai/meetup
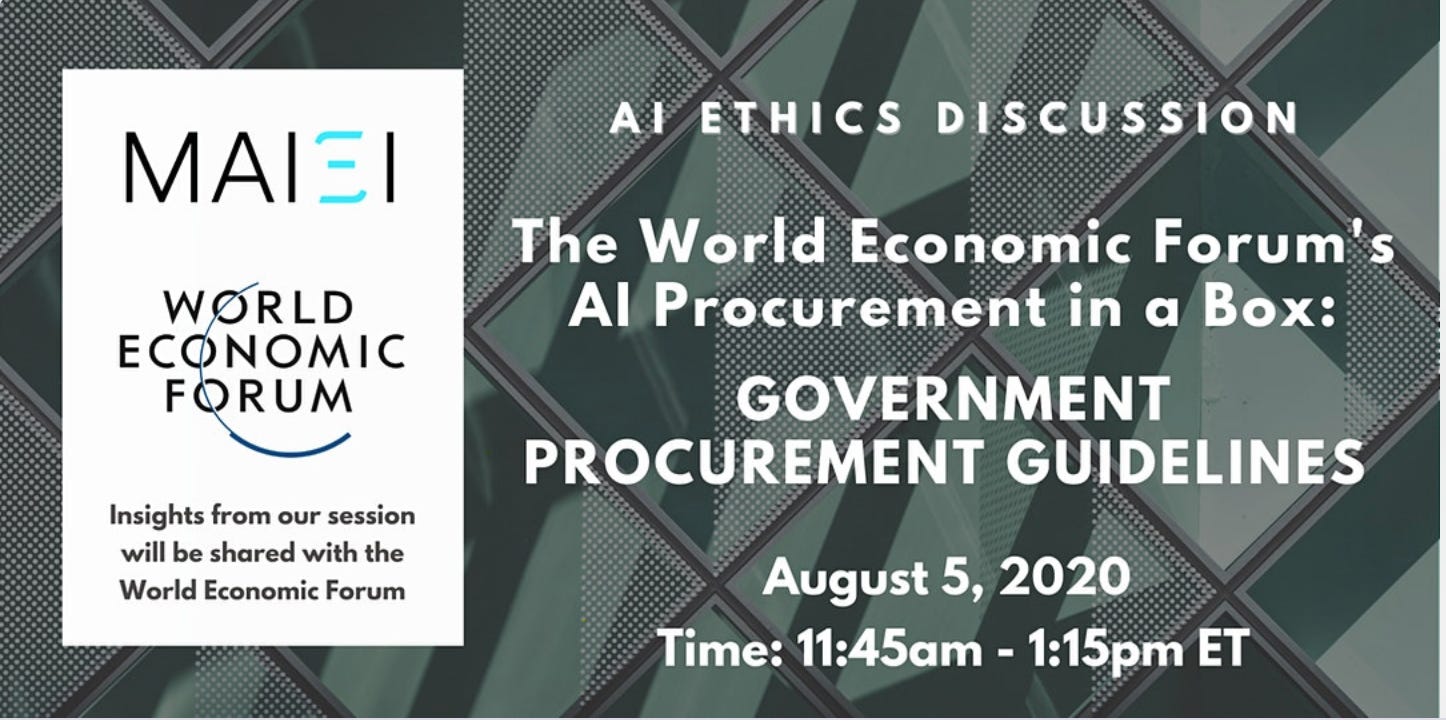
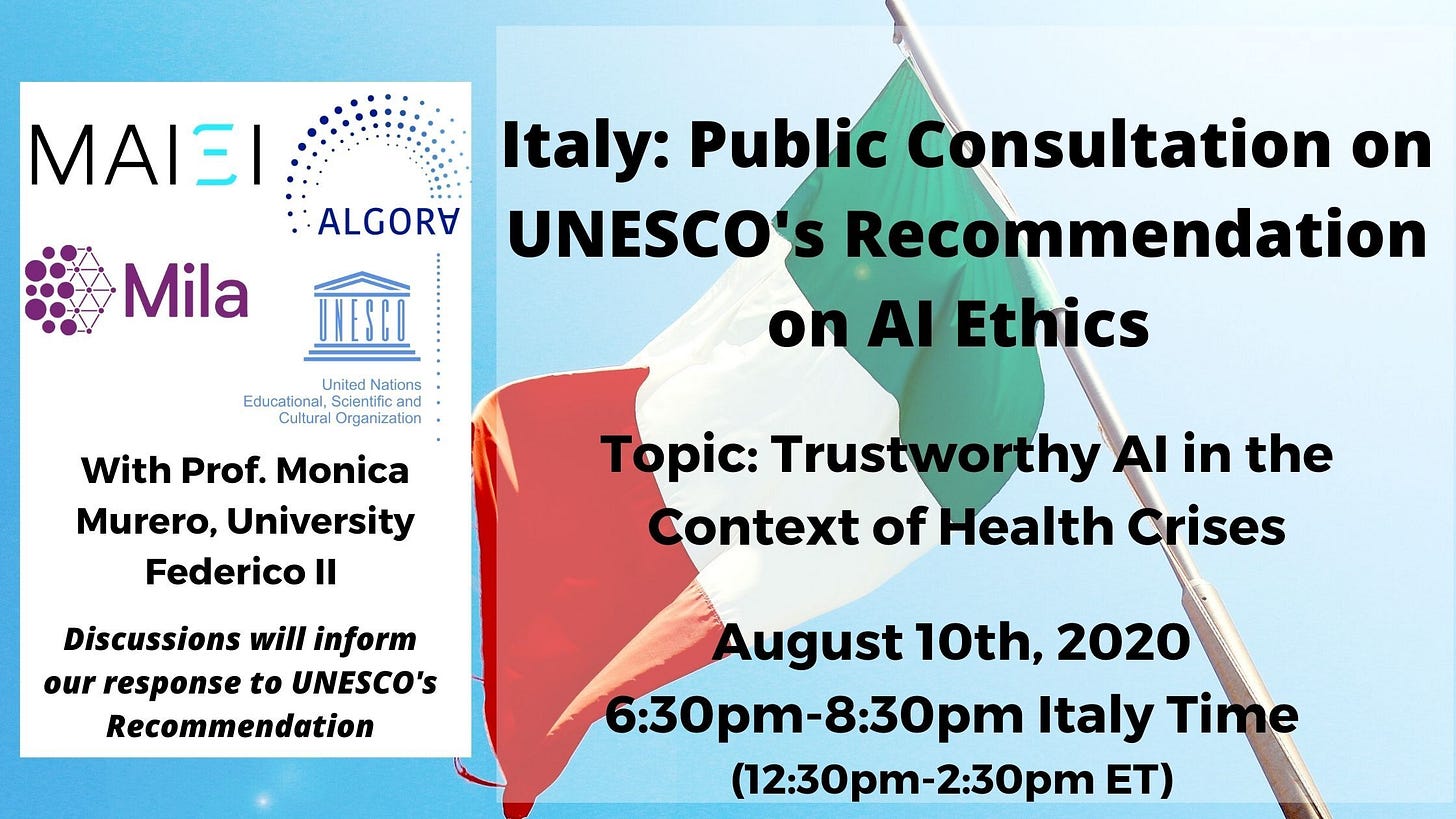
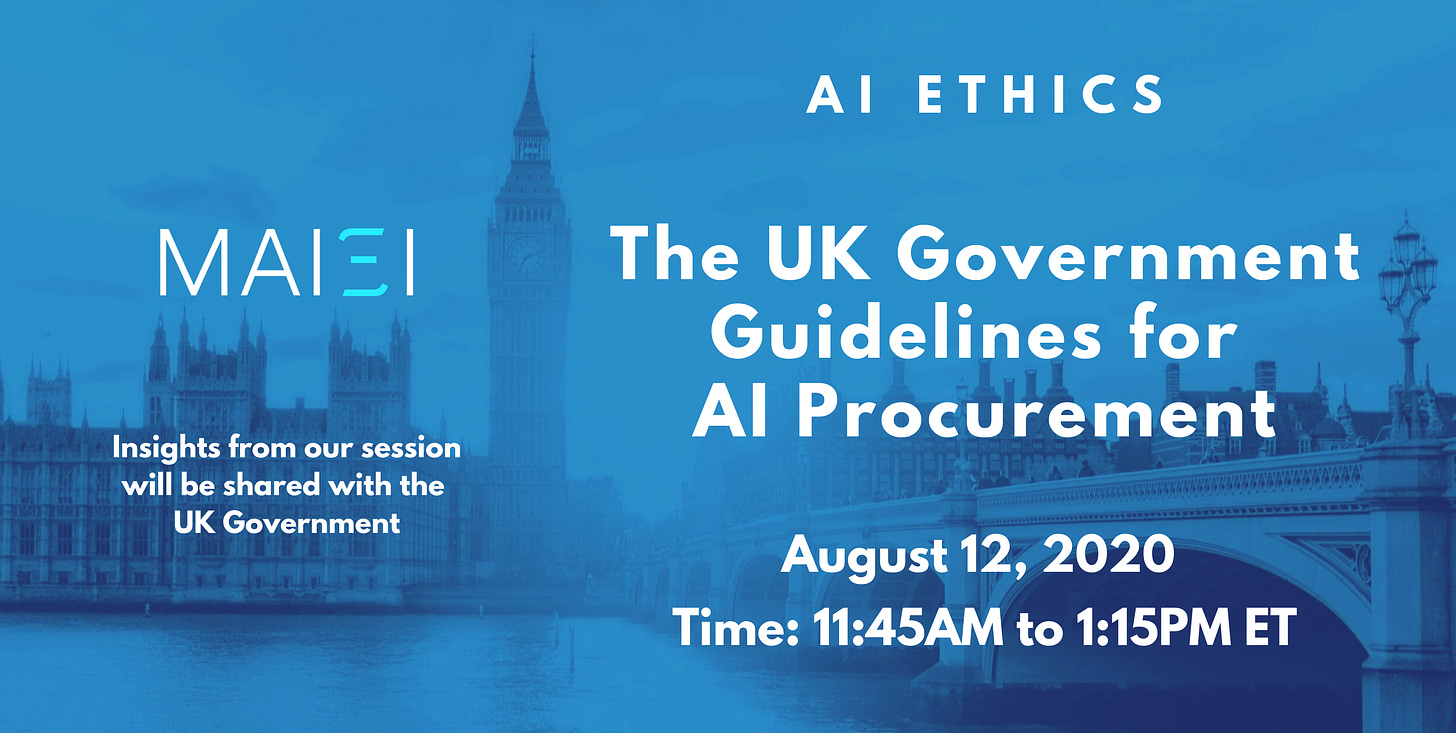
AI Ethics: The World Economic Forum's AI Procurement in a Box
August 5, 11:45 AM - 1:15 PM ET (Online)
Italy: Public Consultation on UNESCO's Recommendation on AI Ethics
August 10, 6:30 PM - 8:30 PM (Italy time) (Online)
AI Ethics: UK Government Guidelines for AI Procurement
August 12, 11:45 AM - 1:15 PM ET (Online)
AI Ethics Framework for the US Intelligence Community
August 26, 11:45 AM - 1:15 PM ET (Online)
You can find all the details on the event page, please make sure to register as we have limited spots (because of the online hosting solution).
From elsewhere on the web:
Things from our network and more that we found interesting and worth your time.
White Paper On Artificial Intelligence: An Opinion by the Montreal AI Ethics Institute by Muriam Fancy
The Montreal AI Ethics Institute (“MAIEI”) prepared a series of recommendations based on the findings from the European Commission Whitepaper on AI. The findings were supported by a series of workshops on May 27th and June 3rd, 2020, and participation from members within the AI ethics community. This paper aims to summarize the findings of MAIEI’s report on the Whitepaper.
To delve deeper, read the full article here.
Trust me!: How to use trust-by-design to build resilient tech in times of crisis by Gabrielle Paris Gagnon, Esq., and Vanessa Henri, Esq., Fasken, and Abhishek Gupta, Montreal AI Ethics Institute
Nations across the world have started to deploy their own contact-and proximity tracing apps that claim to be able to balance the privacy and security of users’ data while helping to combat the spread of COVID-19, but do users trust them? The efficacy of such applications depends, among other things, on high adoption and consistent use rates, but this will be made difficult if users do not trust the tracing apps. Trust is a defining factor in the adoption of emerging technologies, and tracing apps are not an exception. In this article, we argue that trust-based design is critical to the development of technologies and use of data during crisis such as the COVID-19 pandemic. Trust helps to maintain social cohesion by hindering misinformation and allowing for a collective response.
To delve deeper, read the full article here.
Signing off for this week, we look forward to it again in a week! If you enjoyed this and know someone else that can benefit from this newsletter, please share it with them!
If you have feedback for this newsletter or think there is an interesting piece of research, development or event that we missed, please feel free to email us at support@montrealethics.ai