AI Ethics #20: Machines complementing humans, GDPR and CCPA, UBI and the value of data, cookies and journalism, and more ...
Harnessing adversarial examples, scary AI-generated text, picking locks with audio technology, personal data is a national security issue, virtues not principles, and more from the world of AI ethics.
Welcome to the twentieth edition of our weekly newsletter that will help you navigate the fast changing world of AI Ethics! Every week we dive into research papers that caught our eye, sharing a summary of those with you and presenting our thoughts on how it links with other work in the research landscape. We also share brief thoughts on interesting articles and developments in the field. More about us on: https://montrealethics.ai/about/
If someone has forwarded this to you and you want to get one delivered to you every week, you can subscribe to receive this newsletter by clicking below:
Summary of the content this week:
In research summaries this week, we cover fairness in clustering with multiple sensitive attributes, building governance in online communities, learning to complement humans, comparing GDPR and CCPA, changing one’s mind about AI, UBI, and the value of data, and explaining and harnessing adversarial examples.
In article summaries this week, if killing cookies can save journalism, how open data could be a more effective way of regulating the power of Big Tech rather than breaking them up, why AI-generated text is the scariest deepfake of them all, using audio technology to pick locks, why personal data is a national security issue, and why Wikipedia decided to stop calling Fox News a reliable source.
In op-eds this week, we talk about virtues not principles as a way of building responsible AI systems.
In upcoming events, we have a session being hosted to collect feedback on the Responsible AI principles from the Intelligence Community of the US Government and a session on Facial Recognition Technologies. Scroll to the bottom of the email for more information.
MAIEI Community Initiatives:
Our learning communities and the Co-Create program continue to receive an overwhelming response! Thank you everyone!
We operate on the open learning concept where we have a collaborative syllabus on each of the focus areas and meet every two weeks to learn from our peers. You can fill out this form to receive an invite!
AI Ethics Concept of the week: ‘AI Consciousness’
Can AI can be made aware of its own decisions? If it can, does that imply moral responsibility? The Trolley Problem is described as an illustrative example of this concept.
Learn about the relevance of AI consciousness to AI ethics and more in our AI Ethics Living dictionary. 👇
Explore the Living Dictionary!
MAIEI Serendipity Space:
The first session was a great success and we encourage you to sign up for the next one!
This will be a 30 minutes session from 12:15 pm ET to 12:45 pm ET so bring your lunch (or tea/coffee)! Register here to get started!
Research:
Let's look at some highlights of research papers that caught our attention at MAIEI:
Fairness in Clustering with Multiple Sensitive Attributes by Savitha Sam Abraham, Deepak P., Sowmya S Sundaram
With the expansion and volume of readily available data, scientists have set in place AI techniques that can quickly categorize individuals based on shared characteristics. A common task in unsupervised machine learning, known as clustering, is to identify similarities in raw data and group these data points into clusters. As is frequently the case with decision-making algorithms, notions of fairness are put into the forefront when discriminatory patterns and homogenous groupings start to appear.
For data scientists and statisticians, the challenge is to develop fair clustering techniques that can protect the sensitive attributes of a given population, with each of these sensitive attributes in a cluster proportionately reflecting that of the overall dataset. Until recently, it appeared mathematically improbable to achieve statistical parity when balancing more than one sensitive attribute. The authors offer an innovative statistical method, called Fair K-Means, that can account for multiple multi-valued or numeric sensitive attributes. Fair K-Means can bridge the gap between previously believed incompatible notions of fairness.
To delve deeper, read our full summary here.
PolicyKit: Building Governance in Online Communities by Amy X. Zhang, Grant Hugh, Michael S. Bernstein
Online communities have various forms of governance, which generally include a permission-based model. Although these governance models do work in theory, these models caused the admin/moderator to burn out, lack legitimacy of the platform, and the governance model itself cannot evolve. The need for alternative governance models for online communities is necessary. Different types of governance models were tested on other platforms such as LambdaMOO – which shifted from a dictatorship (governed by “wizards”) to a petition model that involved voting and wizards implemented the outcome of the votes.
Wikipedia and its openness for multiple contributors also faced a series of conflicts such as processing petitions and voting to solve disputes. But again, it was left to the admins to address these issues, a very manual and labor-intensive process. This report aims to present “PolicyKit” as a software platform that allows and empowers online communities to “concisely author” governance procedures on their home platforms. The analysis of PolicyKit is done based on being able to carry out actions and policies such as random jury deliberation, and a multistage caucus.
To delve deeper, read our full summary here.
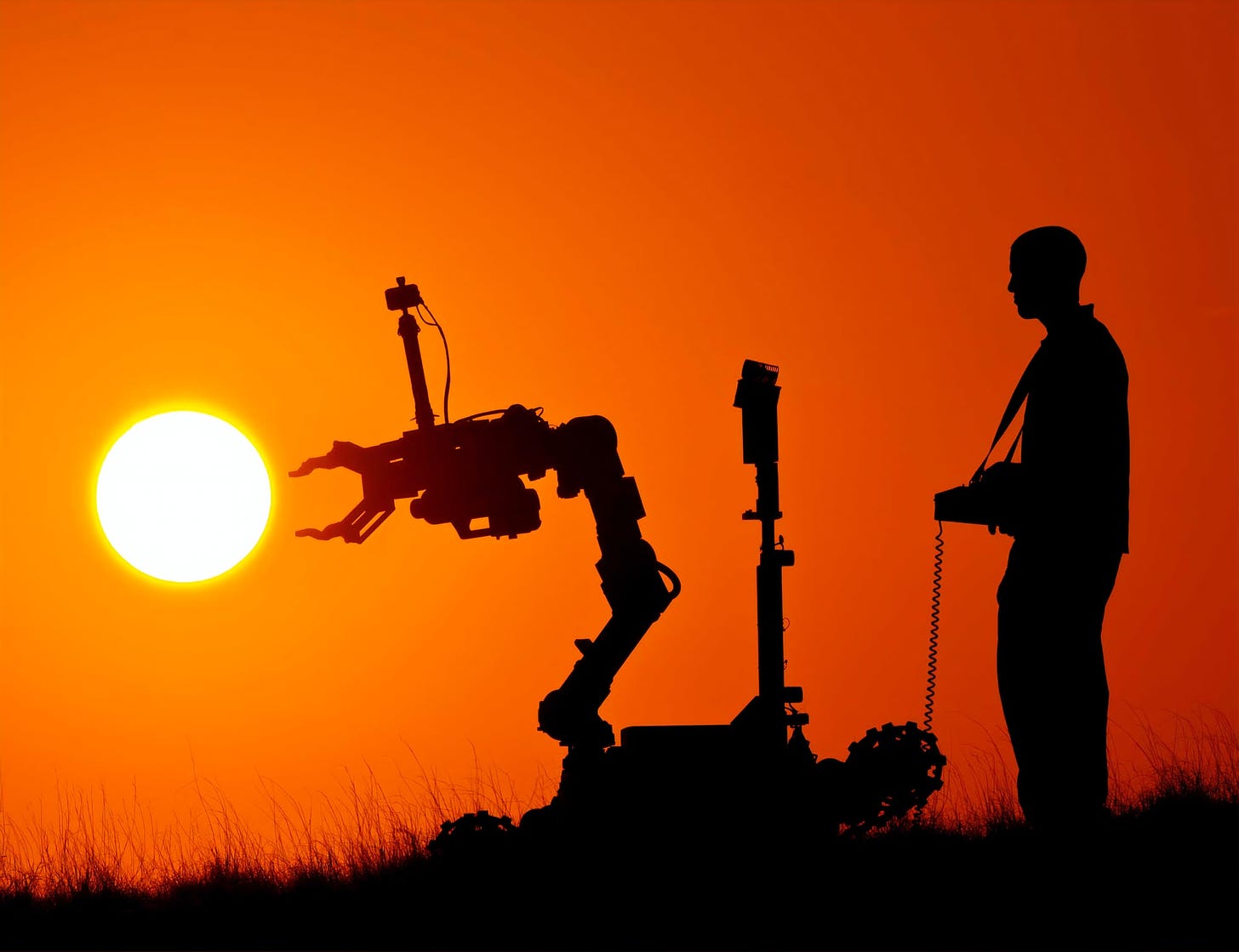
Photo by Science in HD on Unsplash
Learning to Complement Humans by Bryan Wilder, Eric Horvitz, and Ece Kamar
Human machine collaborations have shown to outperform pure human and pure machine systems time and again. This is motivated by the fact that we have complementary strengths which allow us to distribute pieces of tasks in a manner that allow us to complement and cover each other’s weaknesses. In this paper, the authors explain how this can be done better in a discriminative and decision-theoretic setting. They advocate for the use of joint training approaches that keeps the predictive task and the policy task of selecting when to query a human for support together rather than doing that separately.
Experimental results as shown in the paper highlight how this approach leads to results that are never worse and often quite better than other approaches taken in this domain of human-machine complementarity. Specifically, in areas where there are asymmetric costs in terms of the errors that are incurred by the system, the authors find that their approach significantly outperforms existing approaches. This is a welcome sign for the use of machine learning in contexts where high-stakes decisions are taken, for example in medicine where we want to reduce as much as possible on missing diagnosis. Ultimately, utilizing an approach such as this will allow us all to build more safe and robust systems that help us leverage our relative strengths when it comes to making predictions.
To delve deeper, read our full summary here.
What we are thinking:
Op-eds from our research staff that explore some of the most pertinent issues in the field of AI ethics:
Virtues Not Principles by Ryan Khurana
There has been a recent explosion of interest in the field of Responsible Artificial Intelligence (aka AI Ethics). It is well understood that AI is having (and will continue to have) significant social implications as the technology currently exists. Concerns over bias, fairness, privacy, and labour market impacts do not require the arrival of some mythical Artificial General Intelligence (AGI) to be socially destructive. Organisations from around the world are publishing frameworks for the ethical development and deployment of AI, corporations are affirming their commitment to social responsibility by funding responsible AI research and creating review boards, and a political interest in AI has led to state guidelines from numerous countries. Despite this, to many observers, little feels to have changed. Algorithms with suspected bias continue to be used, privacy continues to be a major concern, and accountability is near non-existent.
The failure of progress in responsible AI stems from a mistaken approach which prioritises good outcomes over good behaviour. It is uncontroversial to say that bias is bad and that privacy is good, but what this means in practice is more contentious. By attempting to simplify the work of achieving good outcomes to “frameworks” or “principles” the work being done in the field risks bearing little fruit. Our understanding of how AI systems can lead to problematic social outcomes is inherently reactive, in that we respond to problems that can be documented. The goal of responsible AI, however, is to be proactive by anticipating potential harms and mitigating their impact. Checklists on what ought to be done can never achieve the full range of potential risks that responsible AI seeks to address, and as a result are inherently limited. Proactive concern with socially beneficial outcomes requires not just work on frameworks for ethical use, but the cultivation of virtuous technologists and managers, who are motivated to take the concerns of responsible AI seriously.
To delve deeper, read the full article here.
From our learning communities:
Research that we covered in the learning communities at MAIEI this week, summarized for ease of reading:
Comparing Privacy Law GDPR Vs CCPA by:
DataGuidance: Alice Marini, Alexis Kateifides, Joel Bates
Future of Privacy Forum: Gabriela Zanfir-Fortuna, Michelle Bae, Stacey Gray, Gargi Sen
The paper is a summary of key similarities and distinctions between GDPR and CCPA. The paper analyses these similarities and distinctions in areas including scope, definitions, legal, rights and enforcement areas.
The scope is fairly inconsistent, definitions are fairly consistent, legal grounds are inconsistent, rights are fairly consistent in some cases and enforcement is inconsistent. These analyses are based on the regulations themselves.
Changing My Mind About AI, Universal Basic Income, and the Value of Data by Amy X. Zhang, Grant Hugh, Michael S. Bernstein
Artificial Intelligence may soon become powerful enough to change the landscape of work. When it does, will it devastate the job market and widen the wealth gap, or will it lay the foundation for a technological utopia where human labor is no longer required? A potential intersection between these seemingly opposed theories has developed into an increasingly popular idea in the past 5 years: the idea that human work may become obsolete, but that AI will generate such excess wealth that redistribution in the form of Universal Basic Income is possible. In the article “Changing my Mind about AI, Universal Basic Income, and the Value of Data”, author Vi Hart explores the attractive idea of UBI and AI – long prophesied by tech industry leaders – and weighs its practicality and pitfalls.
A marketplace radically transformed by AI will likely drive workers’ perceived worth down – and UBI may not reverse the harmful results. The utopian vision for AI and UBI, touted by the tech elite, deflects responsibility from corporations to pay for the data labor that is so valuable to them. The author proposes a solution that goes beyond UBI to establish “data dignity”: fair compensation for data labor in a balanced marketplace. Above all else, individuals must be recognized and valued for their data and the labor of producing it. They must be able to reason on the value of their contributions and make the conscious choice to contribute.
To delve deeper, read the full summary here.
Articles:
Let’s look at highlights of some recent articles that we found interesting at MAIEI:
Can Killing Cookies Save Journalism? (Wired)
Cookies help to track users and their behaviour across the internet and have long been heralded as the saving grace for publishers and advertisers as the mechanism that will help them both gain more value from the dollars that are allocated to marketing budgets. Yet, a recent experiment by a major publisher in the Netherlands (NPO) shows otherwise. NPO switched from microtargeting using cookies to non-tracked contextual targeting for ads and found that the revenues that they got actually jumped, even after factoring in for the recessionary effects of the pandemic raging at the moment.
Microtargeting is when users are bucketed into categories based on their demographics and other data that is supposed to allow advertisers to fine-tune who their ads are shown to. Contextual advertising on the other hand relies on the content on the web page and displays the ad based on that rather than using information about the user.
A few reasons have led to better performance of contextual advertising compared to microtargeting according to NPO. The move away from platforms like Google Ads that provide this service means that publishers get to keep a much larger chunk of the incoming revenues. Secondly, a more privacy-conscious audience is inclined to visit websites that don’t explicitly track them. And lastly, perhaps the strongest claim, whether or not someone clicks on an ad selling them pizza is more influenced by whether they are hungry and reading up recipes, than factors like their age group or where they live. This might just be the future of how journalism operates with a restoration of privacy of users while boosting revenues that will make digital journalism sustainable in the long run.
How open data could tame Big Tech’s power and avoid a breakup (The Conversation)
With the recent antitrust hearings where some of the biggest tech companies were put on the stand to justify their behaviour, this article proposes an interesting alternative to the traditional call for breaking up Big Tech. Specifically, it highlights how the problem of breaking up Big Tech is fraught with problems of assessing what constitutes Big Tech. In addition, given the virtuous data cycles and network effects of these platforms, the effects of which have been exacerbated by automation, only serves to repeat the cycle even if Big Tech is broken up into smaller pieces. The smaller pieces have the potential to again metastasize into something large with concentrated market power that would be hard to control. In other cases, the merger of certain smaller players might suddenly create an entity that has unfettered market power.
Instead, a focus on creating public data commons whereby data is made available openly for anyone to use and develop on while protecting the privacy of individuals and keeping the market operations information of firms out of the picture could be a way to distribute market power. While the article is scant in terms of details on how to address the privacy challenges and (in our opinion) mistakenly mentions anonymization as a sufficient methodology for protecting the privacy of individuals, it is nonetheless an interesting idea. Additionally, the article also proposes the creation of a market regulatory authority that could operate in a way similar to the regulation of financial data and how individuals and institutions trade securities for profits and the restrictions that are made in using insider information. Such ideas provide a fresh perspective on the current paradigm and are welcome additions to the debate surrounding the best way to act in the interest of the users.
AI-Generated Text Is the Scariest Deepfake of All (Wired)
In keeping with some of the assessments made by the team at the Montreal AI Ethics Institute, this article highlights why AI-generated text might be the deepfake that we must pay attention to in our fight against disinformation. Specifically, the pervasiveness of deepfake text across all channels and relative lightweight analysis done on it so far makes it quite dangerous. There are two kinds of media that needs to be moderated, one that is synthetic and one that is merely modified, that is, altered taking original content slightly to create something that is meant to deceive.
One of the first problems with text deepfakes is that we must now start to tune not only our content moderation tools to detect them, the first line of defense, but also become more discerning consumers of content. There has been press coverage talking about video and audio deepfakes which will hopefully sensitize consumers to watch out for them. But, there are very limited examples covered in popular media that talk about this. With the recent GPT-3 model, there have been some impressive demonstrations that have been put forward by those who were given beta access to the models. While video and audio deepfakes might be deployed at strategic moments to trigger particular outcomes, say compromising footage before an election, text poses larger problems in that it could be used gradually over time on public fora to sway opinions in the form of a “majority illusion” that shifts public opinion on a subject subtly and without checks.
Better media and digital literacy seem to be our best defenses rather than technological solutions that risk becoming outmoded in an arms race.
Picking Locks with Audio Technology (Communications of the ACM)
The article highlights some recent research work that demonstrates an attack on how to break physical locks using captured audio samples from the insertion of a key into a slot to unlock the door. The metallic clicks as the key goes into the lock can be used to compute the depth of the ridges on a key that would be required so that a duplicate key can be 3D-printed to give someone access to a secured space. The way pin-tumbler locks work is that the ridges on the key alter the height of the pins attached to springs inside the lock and once they are all in the right position it allows the tumbler to turn and unlocks the door. Typically for the most common kinds of these locks there are around 330,000 combinations possible for the patterns on the key. The research approach presented in the article narrows that down to the 3 most likely key patterns.
The research group that produced this work works on harvesting audio and other signals in the environment that are typically of little value and translate them into attacks on the security of systems. As an example, they mention how the gyroscope sensors on a smartwatch and tracking the rotatory movements can help unearth the combination of a safe lock that works through rotation. One of the things that presents a limitation to their current approach is in the quality of audio samples that they are able to capture. Additionally, there are also limitations in terms of interference emerging from other sounds around in the environment, for example, the jingling of other keys, traffic, and other sounds that are typically found in the real-world.
Some of the limitations can be overcome through the attack vectors that were identified in the paper, including the use of machine learning over repeated samples that can help to eliminate noise, and provide a higher degree of accuracy. Yet, it remains a pipe dream at the moment in terms of mounting realistic attacks but as the article mentions, it sounds like a fun idea to insert into a movie plot.
Why Personal Data Is a National Security Issue (Barrons)
The internet is abuzz with TikTok and what the implications of its potential acquisition sets in terms of a precedent for how software products and services work on a global scale, especially when they come into conflict with regulations and legislation, and perhaps even with political agendas. One of the highlights in the article is stressing the importance of interoperable data legislations since data from consumers in one country can be stored in another and if legislations are widely different, that might lead to all sorts of concerns.
From a national security perspective, in places where the government through instruments like lawful intercepts require that a company disclose records, the governments can combine lawfully obtained data with other large, public datasets, even when anonymized, to glean insights that would be beyond the capabilities of other actors in the ecosystem. This can often lead to knowing details about an individual but also at times about the nation as a whole and what its strategies might be in areas of strategic importance. Trust in data ecosystems requires that homegrown legislation is strengthened to protect the rights of people, at the same time ensuring that it is in sync with the operations in the rest of the world.
Why Wikipedia Decided to Stop Calling Fox a ‘Reliable’ Source (Wired)
For an encyclopedia that is built by community members, volunteers, and run by a non-profit organization, the impact that Wikipedia has had on the knowledge ecosystem of the world is immense despite strong criticism that it isn’t thorough enough. Given how many people visit the website to obtain information and how highly it ranks in search engine results, it is essential that information there is as accurate as possible. But this can be hard to achieve for topics related to politics, because the volunteer writers/editors don’t always reach a consensus.
Entries related to Karen Bass received a lot of attention in recent news cycles and editors were working in overdrive to ensure that the information included in her Wikipedia entry were accurate. In some places where Fox News was cited as a source, the editors took that information down citing that it wasn’t a reliable source on that subject. The community editors have decided that instead of doing post-hoc verification, they are branding sources as credible or not in different subject areas. For example, while Fox News is not considered by them to be a reliable source for politics related items, it continues to be maintained as a potential source in other areas. Such an approach helps to avoid bickering and confusion in the distributed editing workflow for the encyclopedia and helps to keep moving things forward.
From elsewhere on the web:
Things from our network and more that we found interesting and worth your time.
Our founder, Abhishek Gupta, recommended the State of AI Ethics June 2020 report as a featured must-read on the RE-WORK blog alongside many other experts from the domain of AI ethics.
Ideas for Improving the Field of Machine Learning: Summarizing Discussion from the NeurIPS 2019 Retrospectives Workshop by Shagun Sodhani, Mayoore S. Jaiswal, Lauren Baker, Koustuv Sinha, Carl Shneider, Peter Henderson, Joel Lehman, and Ryan Lowe
This report documents ideas for improving the field of machine learning, which arose from discussions at the ML Retrospectives workshop at NeurIPS 2019. The goal of the report is to disseminate these ideas more broadly, and in turn encourage continuing discussion about how the field could improve along these axes. We focus on topics that were most discussed at the workshop: incentives for encouraging alternate forms of scholarship, re-structuring the review process, participation from academia and industry, and how we might better train computer scientists as scientists. Videos from the workshop can be accessed at this https URL.
Free course on Practical Data Ethics! (ethics.fast.ai)
In this course, we will focus on topics that are both urgent and practical. In keeping with my teaching philosophy, we will begin with two active, real-world areas (disinformation and bias) to provide context and motivation, before stepping back in Lesson 3 to dig into foundations of data ethics and practical tools. From there we will move on to additional subject areas: privacy & surveillance, the role of the Silicon Valley ecosystem (including metrics, venture growth, & hypergrowth), and algorithmic colonialism. I realize this course still just covers a slice of what is a sprawling field, and I hope that it will be a helpful entry point for continued exploration.
Some notes:
⮕This is a FREE course with no specialized prerequisite knowledge needed
⮕Co-developed by the University of San Francisco’s Data Institute, Rachel Thomas (Director of USF Center for Applied Data Ethics), and fast.ai
⮕It was originally taught to a class of diverse working professionals
⮕There is a transcript search feature that allows jumping to specific parts of lectures
Check out the course at ethics.fast.ai
From the archives:
Here’s an article from our blogs that we think is worth another look:
Explaining and Harnessing Adversarial Examples by Ian J. Goodfellow, Jonathan Shlens and Christian Szegedy
A bemusing weakness of many supervised machine learning (ML) models, including neural networks (NNs), are adversarial examples (AEs). AEs are inputs generated by adding a small perturbation to a correctly-classified input, causing the model to misclassify the resulting AE with high confidence. Goodfellow et al. propose a linear explanation of AEs, in which the vulnerability of ML models to AEs is considered a by-product of their linear behaviour and high-dimensional feature space. In other words, small perturbations on an input can alter its classification because the change in NN activation (as result of the perturbation) scales with the size of the input vector.
Identifying ways to effectively handle AEs is of interest for problems like image classification, where the input consists of intensity data for many thousands of pixels. A method of generating AEs called “fast gradient sign method” badly fools a maxout network, leading to a 89.4% error rate on a perturbed MNIST test set. The authors propose an “adversarial training” scheme for NNs, in which an adversarial term is added to the loss function during training.
This dramatically improves the error rate of the same maxout network to 17.4% on AEs generated by the fast gradient sign method. The linear interpretation of adversarial examples suggests an approach to adversarial training which improves a model’s ability to classify AEs, and helps interpret properties of AE classification which the previously proposed nonlinearity and overfitting hypotheses do not explain.
To delve deeper, read the full article here.
Guest contributions:
If you’ve got an informed opinion on the impact of AI on society, consider writing a guest post for our community — just send your pitch to support@montrealethics.ai. You can pitch us an idea before you write, or a completed draft.
Events:
As a part of our public competence building efforts, we host events frequently spanning different subjects as it relates to building responsible AI systems, you can see a complete list here: https://montrealethics.ai/meetup
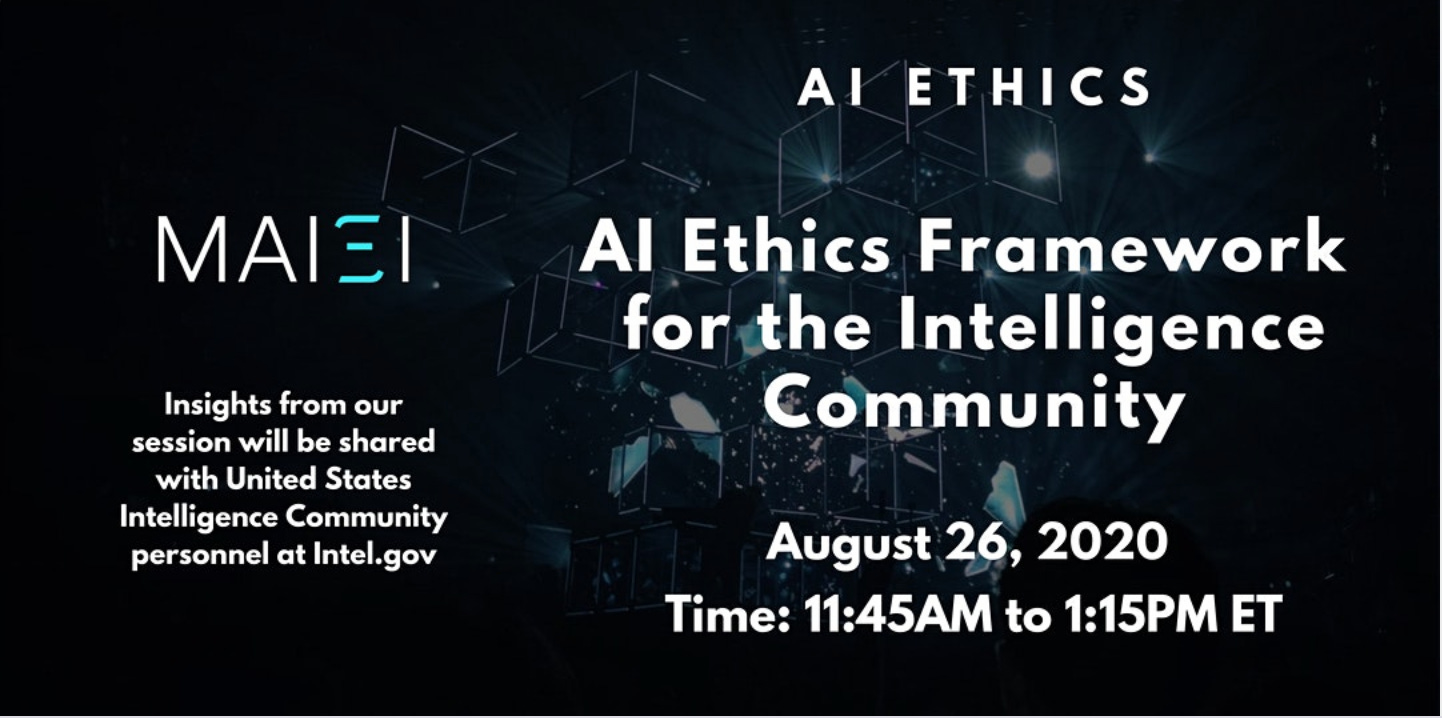
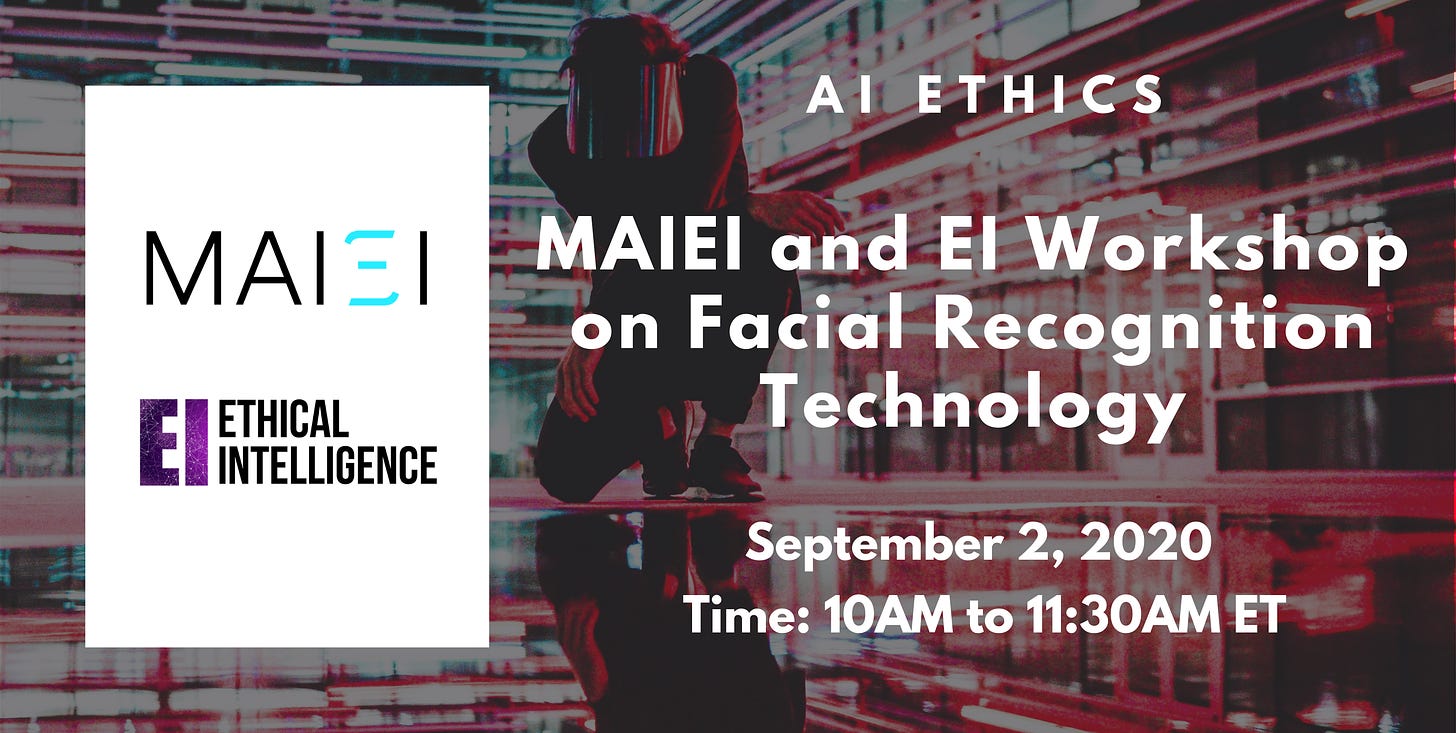
AI Ethics Framework for the US Intelligence Community
August 26, 11:45 AM - 1:15 PM ET (Online)
Facial Recognition Technology Workshop (AI Ethics)
September 2, 10 AM - 11:30 AM ET (Online)
You can find all the details on the event page, please make sure to register as we have limited spots (because of the online hosting solution).
Signing off for this week, we look forward to it again in a week! If you enjoyed this and know someone else that can benefit from this newsletter, please share it with them!
If you have feedback for this newsletter or think there is an interesting piece of research, development or event that we missed, please feel free to email us at support@montrealethics.ai