AI Ethics #23: Repeating society's classic mistakes in AI ethics, contesting ML benchmarks, cognitive science of fake news, insurance for the gig economy, and more ...
TikTok's algorithm and filter bubbles, internet without "like" counts, tools to combat disinformation, facial recognition in border control and more from the world of AI ethics!
Welcome to the twenty-third edition of our weekly newsletter that will help you navigate the fast changing world of AI Ethics! Every week we dive into research papers that caught our eye, sharing a summary of those with you and presenting our thoughts on how it links with other work in the research landscape. We also share brief thoughts on interesting articles and developments in the field. More about us on: https://montrealethics.ai/about/
If someone has forwarded this to you and you want to get one delivered to you every week, you can subscribe to receive this newsletter by clicking below:
Summary of the content this week:
In research summaries this week, we look at facial recognition technology, contesting machine learning benchmarks, algorithmic biases looked at from the lens of implicit biases of social technology, the cognitive science of fake news, and the risk shifts of a gig economy and a normative case for insurance schemes.
In article summaries this week, we look at the efficacy of facial recognition in border control scenarios, new tools from Microsoft to combat disinformation, the internal workings of the TikTok algorithm, how technology companies can advance data science for social good, what building a fair AI really entails, and what the internet would look like without “like” counts.
In featured work from our staff this week, Abhishek and Victoria published a piece for the MIT Technology Review on how we might be repeating some of society’s classic mistakes in the domain of AI ethics, our report on publication norms for responsible AI prepared for Partnership on AI, a podcast discussion on the State of AI Ethics June 2020 report, and finally a call for submissions for a NeurIPS 2020 workshop being co-organized by Abhishek.
In upcoming events, we will be hosting a workshop on the key considerations in building responsible AI systems in partnership with the NSCAI. Scroll to the bottom of the email for more information.
MAIEI Learning Community:
Interested in working together with thinkers from across the world to develop interdisciplinary solutions in addressing some of the biggest ethical challenges of AI? Join our learning community; it’s a modular combination of reading groups + collaborating on papers. Fill out this form to receive an invite!
AI Ethics Concept of the week: ‘Classification’
Classification is a machine learning technique that teaches AI to categorize information. It’s used in spam detection and content recommendation. Humans do it too, like when we sort recycling.
Learn about the relevance of classification to AI ethics and more in our AI Ethics Living dictionary. 👇
Explore the Living Dictionary!
Consulting on AI Ethics by the research team at the Montreal AI Ethics Institute
In this day and age, organizations using AI are expected to do more than just create captivating technologies that solve tough social problems. Rather, in today’s market, the make or break feature is whether organizations using AI espouse concepts that have existed since time immemorial, namely, principles of morality and ethics.
The Montreal AI Ethics Institute wants to help you ‘make’ your AI organization. We will work with you to analyze your organization and/or product offerings for moral and ethical weaknesses and make sure your business model is air tight. By undergoing a thorough, multidisciplinary review of your AI tool, we will provide ethical, social, and technical feedback on your work and research, which will allow you to proactively address your own blindspots and maximize your potential before ever undergoing a third party ethics review.
To find out more, please take a look at this page.
Research:
Let's look at some highlights of research papers that caught our attention at MAIEI:
Snapshot Series: Facial Recognition Technology by Centre for Data Ethics and Innovation
Offering an interactive and well-worded over-arching summary of facial recognition technology (FRT), this paper presents the current situation within the UK. It makes important distinctions between different FRT systems, explains the presence of bias and offers topical case studies of those trying to implement FRT. The paper lists the benefits and risks in one of its 6 sections, as well as explaining how the technology actually works. Both fascinating and engaging, the paper earns a spot as some much needed background reading for any FRT debate.
To delve deeper, read our full summary here.
Bring the People Back In: Contesting Benchmark Machine Learning by Emily Denton, Alex Hanna, Razvan Amironesi, Andrew Smart, Hilary Nicole, Morgan Klaus Scheuerman
The biases present in machine learning datasets, which revealed themselves to favour white, cisgender, male and Western subjects, have received a considerable amount of scholarly attention. Denton et al. argue that the scientific community has failed to consider the histories, values, and norms that construct and pervade such datasets. The authors intend to create a research program, what they termed the genealogy of machine learning, that works to understand how and why such datasets are created. By turning our attention to data collection, and specifically the labour involved in dataset creation, we can “bring the people back in” the machine learning process. For Denton et al., understanding the labour embedded in the dataset will push researchers to critically reflect on the type and origin of the data they are using and thereby contest some of its applications.
To delve deeper, read our full summary here.
Algorithmic Bias: On the Implicit Biases of Social Technology by Gabbrielle M Johnson
The paper presents a comparative analysis of biases as they arise in humans and machines with an interesting set of examples to boot. Specifically, taking a lens of cognitive biases in humans as a way of better understanding how biases arise in machines and how they might be combatted is essential as AI-enabled systems become more widely deployed. What is particularly interesting about the paper is also how the author takes a simple k-nearest neighbor (kNN) approach to showcase how biases arise in practice in algorithmic systems. Also, tackling the hard problem of proxy variables is done through the use of illustrative examples that eschew the overused example of zip codes as a proxy for race. Taking multiple different iterations on the same running example helps to elucidate how biases can crop up in novel ways even when we have made genuine efforts to remove sensitive and protected attributes and made other attempts to prevent biases from seeping into the dataset. Finally, the paper concludes with a call to action for people to closely examine both human and machine biases in conjunction to create approaches that can more holistically address the issues of harm for people who are disproportionately impacted by these systems.
To delve deeper, read our full summary here.
What we are thinking:
Op-eds and other work from our research staff that explore some of the most pertinent issues in the field of AI ethics:
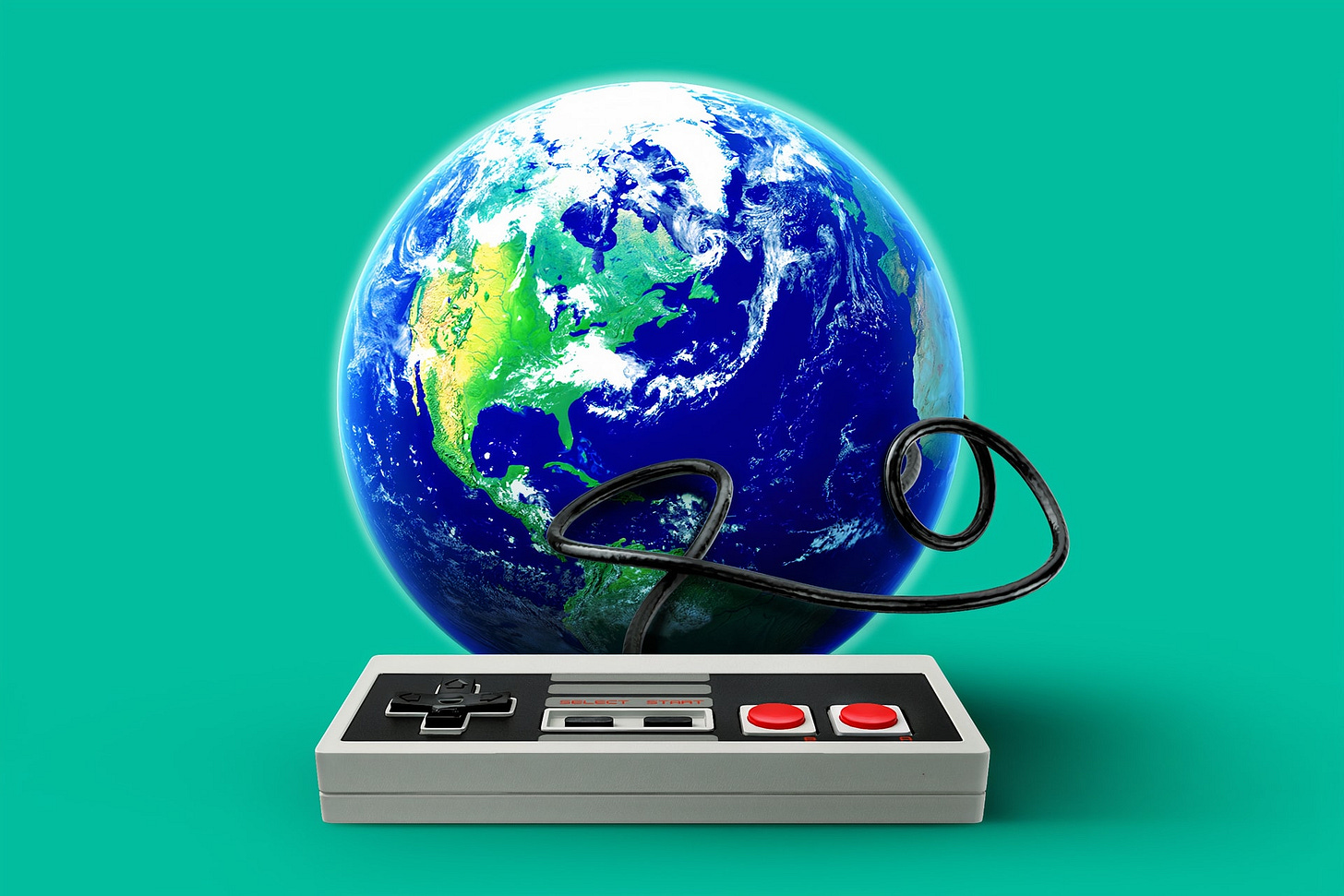
Photo by Morning Brew on Unsplash
The Unnoticed Cognitive Bias Secretly Shaping the AI Agenda by Camylle Lanteigne, AI Ethics Researcher and Research Manager at MAIEI and Ethics Analyst, Algora Lab
This explainer was written in response to colleagues’ requests to know more about temporal bias, especially as it relates to AI ethics.It begins with a refresher on cognitive biases, then dives into: how humans understand time, time preferences, present-day preference, confidence changes, planning fallacies, and hindsight bias.
To delve deeper, read the full article here.
From our learning communities:
Research that we covered in the learning communities at MAIEI this week, summarized for ease of reading:
The cognitive science of fake news by Levy, N. L., & Ross, R. M.
How many people are sincerely fooled by fake news? A moment’s reflection reminds us that we often express attitudes towards propositions that resemble belief, but which aren’t quite the same, and instead signal approval, encouragement, or aspiration, or even mockery. The mainstream account of the psychology of fake news, such as it is given the infancy of this area of study, explains the high level of self-reported belief in fake news as the result of partisan motivated reasoning. This paper, however, challenges both the viability of accepting self-reports at face-value, and raises several challenges to the motivated reasoning explanation.
The paper focuses on three core questions:
To what extent do we really believe fake news?
What explains this belief?
How can we mitigate harms?
To delve deeper, read the full summary here.
Risk Shifts in the Gig Economy: The Normative Case for an Insurance Scheme against the Effects of Precarious Work by Bieber, Friedemann & Jakob Moggia
Authors Bieber and Moggia examine the issue of the gig economy from a political philosophy perspective. It is the notion of “risk shifting” that is central to their analysis, and which is relatively unexplored in the discipline with respect to labour research. The gig economy refers to the phenomenon of hiring workers for concrete and temporary tasks: the supply of labour therefore depends on the demand. The central thesis of the article is that the authors are rather critical of the deleterious effects of the gig economy on workers, as the risk is shifted to workers and becomes a personal one.
They propose a policy framework to policymakers, the “Principle of Inverse Coverage (PIC)”, that would allow them to reduce the risks and compensate for the disadvantages caused to workers and, by extension, to society, without entirely prohibiting the way in which the gig economy operates, which is not entirely detrimental, but whose harmful sides are never entirely erased. This policy would stabilize the working conditions of the gig workers and allow them to project themselves into the future, by giving them back this agility. Compared to the “UBI”, the “PIC” does not generalize the risks of the firms to the whole population but compensates all the same for the risks to which the workers are exposed.
To delve deeper, read the full summary here.
Articles:
Let’s look at highlights of some recent articles that we found interesting at MAIEI:
Border Patrol Used Facial Recognition to Scan More Than 16 Million Fliers — and Caught 7 Imposters (OneZero)
Facial Recognition Technology (FRT) continues to be featured prominently in discussions on AI ethics, specifically around the issues of bias and privacy. Not much of a surprise in this article that talks about a report published by the Government Accountability Office (GAO) in the US on the use of FRT in border crossings. Specifically, the report found that in scanning 16 million passengers only 7 were found to be imposters. While there might be gains to be had in terms of enabling the border patrol to operate in a leaner and more efficient way, such small gains when compared to the potential bias and privacy pitfalls raise concerns.
There was limited information available to passengers with respect to when they were being subjected to automated FRT. Additionally, the opt-out process placed a higher burden on the passengers and the choice was often framed as a negative for the passengers thus discouraging them from opting out. They were told that they would experience delays and additional security checks. Also, when passengers did choose to opt-out, often there was a shortage of staff to process them manually and limited information on the expectations from the alternate process. While the use of AI brings a lot of potential, it is important to consider who bears the brunt of the negative consequences and if the actors who face those burdens have adequate recourse in case things go wrong.
New Steps to Combat Disinformation (Microsoft Blog)
By now readers of this newsletter know that mis-, dis-, and malinformation are problems that are plaguing our current information system online and offline. The impacts are severe but there are few technical and policy solutions that have been shown to be effective. This announcement unveils both technical tools and educational efforts to enhance our defenses when it comes to combating problematic information. On the technical side, Microsoft (disclaimer: Our founder works at Microsoft) has partnered with a number of organizations to create a video authenticator that detects deepfakes by analyzing the boundary between the deepfake elements and grayscale elements and subtle fading that would be imperceptible to a human. The system was trained on the Deep Fake Detection Challenge dataset (we covered that in edition #3) and the Face Forensics++ dataset.
Another technical tool created by the team helps users identify whether the content that they are watching is authentic or not. It adds digital hashes and certificates to the content that travel along with the content as metadata allowing creators of the content to stamp their productions. On the other hand, there is an accompanying consumption tool that can help users verify those hashes and certificates to assert whether the content is authentic or not, and to provide details on the creators of the content.
Finally, given that detection and evasion are an inherently adversarial dynamic, technical solutions alone are not enough. To that end, the team has also partnered with organizations to release media literacy tools that will help to educate the public on signs they should look out for, current capabilities, and other critical thinking skills that will be essential in this fight against problematic information. An implementation of NewsGuard has also been expanded to increase the efforts of rating news and media sources along nine journalistic integrity criteria, essentially creating nutrition labels and red/green marks that can help consumers discern the veracity and authenticity of content coming from those sources.
TikTok reveals details of how its algorithm works (Axios)
While this tool might still fall under the realm of being “cool”, a lot of people outside the target demographic of this tool have been paying attention to it ever since the announcement from POTUS on requiring the parent company to sell the tool to a US-based entity if it were to continue operating in the US, ostensibly one of its most profitable markets; Oracle has since won the deal for TikTok’s US operations. The reason for the success of the platform has been its underlying platform algorithm which is optimized for engagement (as is the case with all other platforms too) taking into account factors such as the expressed interests that evolve over time, the user location, type of device, etc. They use machine learning to cluster users into groups that might have similar preferences but also supplement that with some deterministic rules to prevent showing repeated content that might bore the user.
But, as is the case with all other platforms too, there is a massive implication in terms of creating filter bubbles that can negatively affect the quality of the information ecosystem. Specifically, leading into the election in the US, this poses significant challenges for problematic information to spread on the platform. The policy team at TikTok takes that seriously and mentioned how they have been in contact with lawmakers to ensure that they don’t run into issues and how they might prevent the spread of problematic information on the platform. Ultimately, from an international policy standpoint, whether or not it’s possible will depend on the interpretation that the Chinese government has made on the fine print of the relationship between Oracle and TikTok, notably if this famed algorithm that is supposed to be behind the success of the platform would be allowed to be transferred outside of China due to the new rules imposed by the Chinese government.
How Tech Companies Can Advance Data Science for Social Good (Stanford Social Innovation Review)
Reminiscent of the some of the thoughts put forward by our founder, Abhishek Gupta, in a piece for the SSIR almost 2 years ago on AI as Force for Good, this piece identifies some of the ways that the landscape has changed since and catalogues some of the initiatives in this space that are helping to equip non-profits with the technology and skills to apply AI to their work. Specifically, there is a great deal of enthusiasm on the part of technology companies to aid with this but the article urges them to ask critical questions such as what might be some of the incentives and disincentives for an organization to utilize AI, what skills gaps exist, and what gaps can funders help to fill.
Based on some of the conversations by the author, 4 key takeaways that emerged from this were the following:
Focus on what it takes in the preprocessing stages of AI to make projects successful. Specifically, an emphasis on the work that is required to clean and prepare data is often invisible in the final result and equipping data scientists so that they can carry this out more effectively is crucial.
Helping the organizations build internal capacity so that they can carry out the work rather than having to continuously rely on external partners for skills and technology.
Supplementing that through skills transfer by having experienced data scientists work with the local teams is a great way to share knowledge and build internal capacity. Those who have a proven track record in being good communicators and teachers are great candidates for this.
Finally, providing insights such that the use of data is done in an ethical and responsible manner is also important, especially as a lot of these organizations work in areas that have significant impacts on human lives.
What Does Building a Fair AI Really Entail? (Harvard Business Review)
The article presents an oft-ignored perspective towards building AI-enabled systems that are fair, namely, the organizational scaffolding that is required to create AI fairness beyond just technical measures. In essence, it is not enough to have technical solutions that achieve fairness according to some definition as specified in academic literature. When rubber meets the road, there are a lot of other factors that go into the making of what is perceived as a fair AI system.
In terms of adoption of these systems, both by employees and users of the product, their perception of the degree of fairness of the organization plays an equal importance role. The more fair the organization is deemed to be, the more willing the users and employees are to accept the system. One recommendation made by the author in the article is to treat fairness in these systems as a cooperative act where you have a human devil’s advocate to validate and check for the fairness of the system. Demonstrated through prior research, it is evident that humans are more likely to spot biases in other people than themselves and utilizing that trick here can lead to fairer systems.
The second recommendation made by the author is to look at the tradeoffs between utility and humanity of the system. This requires articulating the values that are important to the organization and squaring them with the technical goals as specified for the system. Finally, being transparent in communication, in treating the larger community with due respect also play an important role in the perception of the organization being fair and ultimately amplifying the impact of their work in building fair AI systems.
Would the Internet Be Healthier Without 'Like' Counts? (Wired)
Mental health problems due to addiction to internet usage is something that is discussed in waves under varying circumstances. This article dives into the intricacies of the efforts made by different platforms in terms of reorienting their services (potentially) towards a metric-free experience. The argument goes that this radicalizes the consumption patterns for the users who pay more attention to the metrics rather than the content. On the creators end, it creates obsessive behaviour patterns which skew them towards putting out content that is apparently optimized for garnering higher counts of these metrics on the platforms.
Even before these talks began, artists and activists have put tools that have sparked a movement called demetrication, including extensions in your browsers to help you do that. One of the other positive impacts of this is to discourage the purchase of bot accounts that help to artificially boost counts and depress the sales and prevalence of shady companies on platforms who try to trick users into buying low quality products or services. Experiments on Instagram, Facebook, YouTube, and Twitter have shown mixed results thus far and a lot of keen watchers of the space have argued that this might not eventually come to be, especially in the case where one might experience lower rates of engagement on the platform since that would hurt the bottomline in a highly lucrative and competitive domain.
From elsewhere on the web:
Things from our network and more that we found interesting and worth your time.
AI Ethics groups are repeating one of society’s classic mistakes by Abhishek Gupta and Victoria Heath for the MIT Technology Review
"The problem: AI systems have repeatedly been shown to cause problems that disproportionately affect marginalized groups while benefiting a privileged few. The global AI ethics efforts underway today—of which there are dozens—aim to help everyone benefit from this technology, and to prevent it from causing harm. These groups are well-intentioned and are doing worthwhile work.
However… Without more diverse geographic representation, they’ll produce a global vision for AI ethics that reflects the perspectives of people in only a few regions of the world, particularly North America and northwestern Europe. If unaddressed, they risk developing standards that are, at best, meaningless and ineffective across all the world’s regions. At worst, these flawed standards will lead to more AI systems and tools that perpetuate existing biases and are insensitive to local cultures."
To delve deeper, read the full article here.
The history of science and technology shows that seemingly innocuous developments in scientific theories and research have enabled real-world applications with significant negative consequences for humanity. In order to ensure that the science and technology of AI is developed in a humane manner, we must develop research publication norms that are informed by our growing understanding of AI's potential threats and use cases. Unfortunately, it's difficult to create a set of publication norms for responsible AI because the field of AI is currently fragmented in terms of how this technology is researched, developed, funded, etc. To examine this challenge and find solutions, the Montreal AI Ethics Institute (MAIEI) collaborated with the Partnership on AI in May 2020 to host two public consultation meetups. These meetups examined potential publication norms for responsible AI, with the goal of creating a clear set of recommendations and ways forward for publishers.
In its submission, MAIEI provides six initial recommendations, these include: 1) create tools to navigate publication decisions, 2) offer a page number extension, 3) develop a network of peers, 4) require broad impact statements, 5) require the publication of expected results, and 6) revamp the peer-review process. After considering potential concerns regarding these recommendations, including constraining innovation and creating a "black market" for AI research, MAIEI outlines three ways forward for publishers, these include: 1) state clearly and consistently the need for established norms, 2) coordinate and build trust as a community, and 3) change the approach.
To delve deeper, read the full report here.
Our founder, Abhishek Gupta, is co-organizing the following workshop that is now accepting submissions:
The ML-Retrospectives, Surveys & Meta-Analyses @ NeurIPS 2020 Workshop is about reflecting on machine learning research. This workshop is a new edition of the previous Retrospectives Workshops at NeurIPS’19 and ICML’20 respectively. While earlier the focus of the workshop was primarily on Retrospectives, this time the focus is on surveys & meta-analyses. The enormous scale of research in AI has led to a myriad of publications. Surveys & Meta-Analyses meet the need of taking a step back and looking at a sub-field as a whole to evaluate actual progress. However, we will also accept retrospectives.
In conjunction with NeurIPS, the workshop will be held virtually. Please see our schedule for details.
To delve deeper, take a look at the workshop website here.
The research team from the Montreal AI Ethics Institute spoke about the State of AI Ethics Report June 2020 on the AI Asia Pacific Institute podcast covering some of the research and development that we believe to be the most important when it comes to the domain of AI ethics.
From the archives:
Here’s an article from our blogs that we think is worth another look:
Report on the Santa Clara Principles for Content Moderation by the Montreal AI Ethics Institute team
In April 2020, the Electronic Frontier Foundation (EFF) publicly called for comments on expanding and improving the Santa Clara Principles on Transparency and Accountability (SCP), originally published in May 2018. The Montreal AI Ethics Institute (MAIEI) responded to this call by drafting a set of recommendations based on insights and analysis by the MAIEI staff and supplemented by workshop contributions from the AI Ethics community convened during two online public consultation meetups.
To delve deeper, read the full article here.
Guest contributions:
If you’ve got an informed opinion on the impact of AI on society, consider writing a guest post for our community — just send your pitch to support@montrealethics.ai. You can pitch us an idea before you write, or a completed draft.
Why We Need to Audit Government AI by Alayna Kennedy, Public Sector Consultant and AI Ethics Researcher at IBM
Artificial Intelligence (AI) technology has exploded in popularity over the last 10 years, with each wave of technical breakthroughs ushering more and more speculation about the potential impacts of AI on our society, businesses, and governments. First, the Big Data revolution promised to forever change the way we understood analytics, then Deep Learning promised human-level AI performance, and today AI offers huge business returns to investors. AI has long been a buzzword in businesses across the world, but for many government agencies and larger organizations, earlier applications of commercial AI proved to be overhyped and underwhelming. Only now are large-scale organizations, including governments, beginning to implement AI technology at scale, as the technology has moved from the research lab to the office.
Each of the waves of AI development has been accompanied by a suite of ethical concerns and mitigation strategies. Between 2016 and 2019, 74 sets of ethical principles or guidelines for AI were published, focusing on high-level guidance like “creating transparent AI.” These high-level principles rarely provided concrete guidance, and often weren’t necessary, since most large organizations and government agencies were not yet using AI at scale. In recent years, the AI Ethics community has moved past high-level frameworks and begun to focus on statistical bias mitigation. A plethora of toolkits, including IBM’s AIF360, Microsoft’s Fairlearn, and FairML, have emerged to combat bias in datasets and in AI models.
To delve deeper, read the full article here.
Events:
As a part of our public competence building efforts, we host events frequently spanning different subjects as it relates to building responsible AI systems, you can see a complete list here: https://montrealethics.ai/meetup
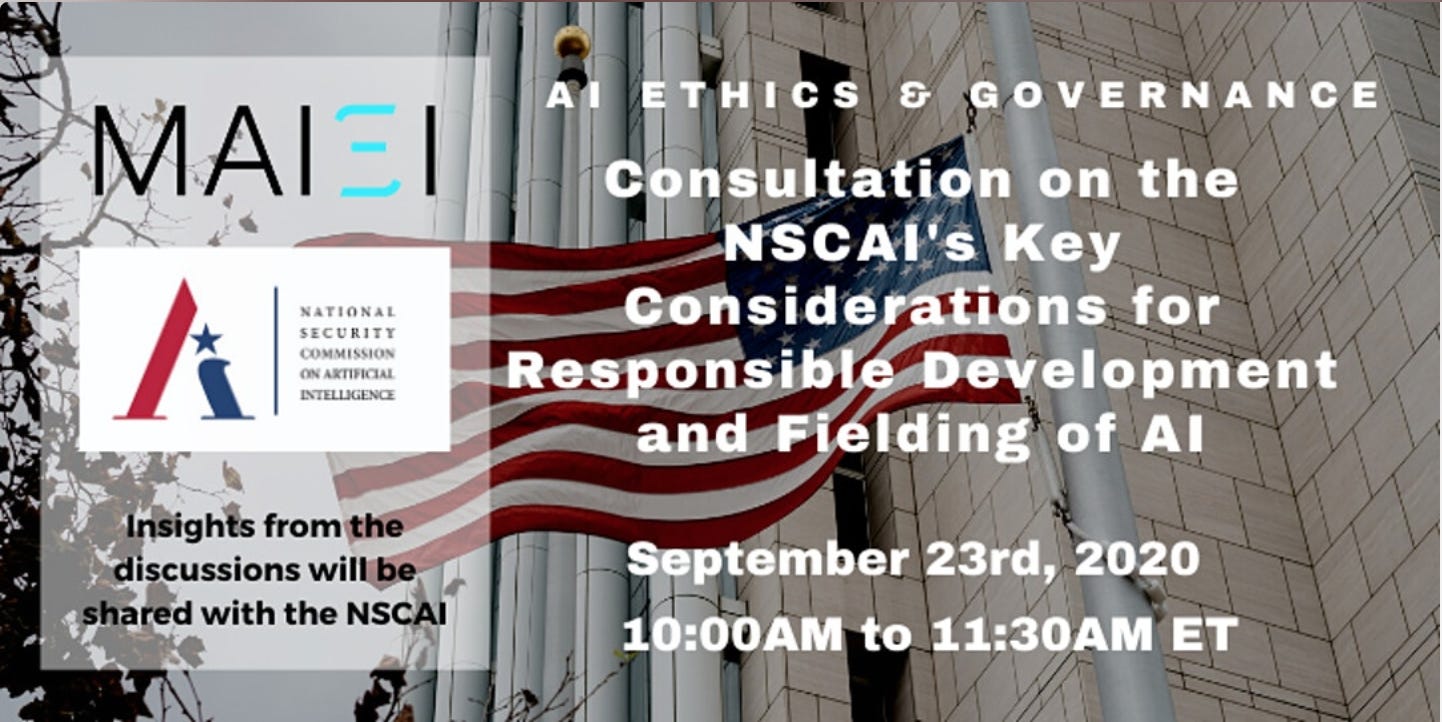
MAIEI Consultation: NSCAI's Key Considerations for Responsible AI
September 23, 10 AM - 11:30 AM ET (Online)
You can find all the details on the event page, please make sure to register as we have limited spots (because of the online hosting solution).
Signing off for this week, we look forward to it again in a week! If you enjoyed this and know someone else that can benefit from this newsletter, please share it with them!
If you have feedback for this newsletter or think there is an interesting piece of research, development or event that we missed, please feel free to email us at support@montrealethics.ai