AI Ethics #8: Adversarial ML politics, tech-enabled disinformation, specification gaming, AI making art, comparing human with machine thoughts, and more ...
Our eighth weekly edition covering research and news in the world of AI Ethics
Welcome to the eighth edition of our weekly newsletter that will help you navigate the fast changing world of AI Ethics! Every week we dive into research papers that caught our eye, sharing a summary of those with you and presenting our thoughts on how it links with other work in the research landscape. We also share brief thoughts on interesting articles and developments in the field. More about us on: https://montrealethics.ai/about/
If someone has forwarded this to you and you want to get one delivered to you every week, you can subscribe to receive this newsletter by clicking below
After a great session with enthusiastic participation from our community on Scotland’s AI Strategy, our next two sessions will be focused on Publication Norms for Responsible AI.
“ … one important aspect in upholding ‘responsible AI’ is the consideration of when and how to publish novel research in a way that maximizes benefits while mitigating potential harms.” - Partnership on AI.
We’re working with Partnership on AI for these events and look forward to your participation! You can get your tickets from here.
In research summaries this week, we dive into the idea of how defenses in adversarial machine learning are not politically neutral and how they can harm legitimate interests, thinking about what AI-assisted healthcare capabilities entail and what are the emerging challenges with technology-enabled disinformation efforts.
In article summaries, we talk about how computers don’t really author art, how AI-assisted healthcare tools may stay past their welcome beyond the pandemic, how to reasonably use demographic data to counter bias, finding misinformed public on Facebook, comparing human and machine thoughts, and specification gaming, i.e. how machines can achieve goals we set for them, just not how we expected them to.
Our learning communities have received an overwhelming response! Thank you everyone!
We operate on the open learning concept where we have a collaborative syllabus on each of the focus areas and meet every two weeks to learn from our peers. We are starting with 5 communities focused on: disinformation, privacy, labor impacts of AI, machine learning security and complex systems theory. You can fill out this form to receive an invite!
Hoping you stay safe and healthy and looking forward to seeing you at our upcoming public consultation sessions and our learning communities! Enjoy this week’s content!
Research:
Let's look at some highlights of research papers that caught our attention at MAIEI:
Health Care, Capabilities, and AI Assistive Technologies by Mark Coeckelbergh
The adoption of AI-enabled solutions in the healthcare industry has accelerated with the ongoing pandemic and while there are a lot of concerns raised, most quite aptly, there is a need to evaluate these concerns in firm moral principles and foundations before dismissing these solutions as not meeting our high standards of care. Another argument put forth is the potential replacement of human carers by the use of such technologies to the detriment of the quality of care that would otherwise be provided to patients.
However, this overlooks the fact that already due to high burdens on the healthcare sector, often care is quite low-touch and distanced thus not creating that much of a difference. In fact, AI-enabled solutions might even provide an opportunity for improving the healthcare outcomes by automating routine and repetitive tasks minimizing the burnout experienced by healthcare professionals and enabling them to concentrate their efforts on the aspects that are not yet replicable by machines.
This paper provides more examples where it does a careful evaluation of the tradeoffs between the use of technology and achieving some of the aims of realizing a good life as characterized by the capabilities approach. Especially, in a time where there is a rush towards picking a solution and deploying it within the healthcare industry to combat the surge in care demand because of COVID-19, the paper offers some guidelines rooted in theory with practical applications for making a choice that is well-informed.
To delve deeper, read our full summary here.
Technology-Enabled Disinformation: Summary, Lessons, and Recommendations by Akers et al.
The upcoming election cycle and the current pandemic have made all of us into inveterate consumers of copious amounts of information from a variety of sources. For a lot of people, their primary source of information is social media where they rely not only on the official accounts of large media organizations but also on content that is shared by people they know and they don’t know. There is an increased importance of being able to successfully combat mis/disinformation, especially when it means that one can achieve better health outcomes and safeguard the state of our democracies.
This paper provides a comprehensive overview of the state of the mis/disinformation ecosystem providing guidance on specific technical and design interventions that help us build a better understanding of the disinformation ecosystem. It also brings forth the dire need for interdisciplinary collaboration highlighting the challenges that researchers face today when analyzing some of the platforms that operate on closed-network models. Most research today focuses on Twitter because of its public nature but there is a need to get deeper insights into the state of how information flows in closed groups and how it is perceived by users. There is also an explanation offered into how novel technology is accelerating the pace and impact of disinformation campaigns and how the variation in the motivations of the actors necessitates tailored defenses.
The ecosystem is inherently adversarial, just as in the world of cybersecurity, and hence being successful today in detecting and combating disinformation doesn’t mean that we can do so tomorrow. The constant evolution in understanding of the ecosystem coupled with redesigning some of the affordances on the platforms that better meet the needs of diverse users is crucial. We need to build defenses that don’t just cater to those who are technically-literate and easing the burden of detecting disinformation from the shoulders of users is important because the onus needs to be shared by the platforms and the regulators as well.
Recommendations are also made for how policymakers can meaningfully engage in this space and how educational initiatives for the users can help increase the quality of interactions on the platform. Ultimately, tailored approaches that rely on interdisciplinary expertise attacking the root causes of the spread of disinformation by analyzing the sources, plumbing of platforms that permits rapid dissemination, the motivations and incentives of the various actors in the ecosystem and the perception issues on the end of the users need to be addressed in a piecewise, tangible manner such that the problems are tractable and the solutions to those problems can then be combined into a pipeline to more comprehensively mitigate the harm from disinformation.
To delve deeper, read our full summary here.
Politics of Adversarial Machine Learning by Kendra Albert, Jonathon Penney, Bruce Schneier and Ram Shankar Siva Kumar
A very timely paper that in the post-ClearviewAI world brings forth some important issues about how to think about the societal implications of the work done in the ML Security space. (If this is your first encounter with this term, please take a look through our learning community at the Montreal AI Ethics Institute to know more, we believe that this emergent area is of prime importance as ML systems become more widely deployed.) The paper takes the case study of facial recognition technology as model for reasoning about some of the challenges that we encounter when we harden ML systems against adversarial attacks. It provides an insightful reframing of the meaning of attacks against a system, moving away from the typical notions of cybersecurity wherein an attacker is an entity that compromises the confidentiality, integrity and availability of a system for some malicious purpose.
Examples of when that’s not the case include someone trying to learn whether there image was used in a dataset that the system was trained on, determining whether an opaque ML system has bias issues and protecting identities of protestors and other vulnerable populations who are fighting for their civil liberties and human rights in states where they might be persecuted if they are recognized. Drawing on lessons learned from the work done by civil society organizations and others to combat ethical, safety and inclusivity issues from the commercial spyware industry, the authors urge developers and vendors of ML systems to consider human rights by design principles and other recommendations when thinking about hardening their ML systems against adversarial attacks.
To delve deeper, read our full summary here.
Articles:
Let’s look at highlights of some recent articles that we found interesting at MAIEI:
Want to Find a Misinformed Public? Facebook’s Already Done It (The Markup)
The social media platform offers a category of pseudoscience believers which advertisers can purchase and target. According to The Markup, this category has 78 million people in it and attempts to purchase ads targeting this category were approved quite swiftly. There isn’t any information available as to who has purchased ads targeting this category. The journalist team was able to find at least one advertiser through the “Why am I seeing this ad?” option and they reached out to that company to investigate and they found that the company hadn’t selected the pseudoscience category but it had been auto-selected by Facebook for them. Facebook allows users the option to change the interests that are assigned to each user but it is not something that many people know about and actively monitor. Some other journalists had also unearthed controversy-related categories that amplified messages and targeted people who might be susceptible to such kind of misinformation. With the ongoing pandemic, misinformation is propagating at a rapid rate and there are many user groups that continue to push conspiracy theories. Other concerns around being able to purchase ads to spread misinformation related to potential cures and remedies for the coronavirus continue to be approved. With the human content moderators being asked to stay home (as we covered here) and an increasing reliance on untested automated solutions, it seems that this problem will continue to plague the platform.
At the limits of thought (Aeon)
Since time immemorial there has been a constant tussle between making predictions and being able to understand the underlying fundamentals of how those predictions worked. In the era of big data, those tensions are exacerbated as machines become more inscrutable while making predictions using ever-more higher-dimensional data which lies beyond intuitive understanding of humans. We try to reason through some of that high-dimensional data by utilizing techniques that either reduce the dimensions or visualize into 2- or 3-dimensions which by definition will tend to lose some fidelity. Bacon had proposed that humans should utilize tools to gain a better understanding of the world around them - until recently where the physical processes of the world matched quite well with our internal representations, this wasn’t a big concern. But a growing reliance on tools means that we rely more on what is made possible by the tools as they measure and model the world.
Statistical intelligence and models often get things right but often they are hostile to reconstruction as to how they arrived at certain predictions. Models provide for abstractions of the world and often don’t need to follow exactly the real-world equivalents. For example, while the telescope allows us to peer far into the distance, its construction doesn’t completely mimic a biological eye. More so, radio telescopes that don’t follow optics at all give us a unique view into distant objects which are just not possible if we rely solely on optical observations.
Illusions present us with a window into the limits of our perceptual systems and bring into focus the tension between the reality and what we think is the reality. Through a variety of examples like the Necker Cube, one can demonstrate that our perception and reality can often have gaps. A statistical analogue is the Simpson’s Paradox where insights gleaned from one dataset are completely reversed when analyzed at a different scale or by combining multiple datasets. Accuracy paradoxes do something similar where underrepresentation in a dataset of a minority leads to poor performance of the predictions for those minorities, what is often dubbed as algorithmic bias.
“In just the same way that prediction is fundamentally bounded by sensitivity of measurement and the shortcomings of computation, understanding is both enhanced and diminished by the rules of inference.”
In language models, we’ve seen that end-to-end deep learning systems that are opaque to our understanding perform quite a bit better than traditional machine translation approaches that rest on decades of linguistic research. This bears some resemblance to Searle’s Chinese Room experiment where if we just look at the inputs and the outputs, there isn’t a guarantee that the internal workings of the system work in exactly the way we expect them to.
“The most successful forms of future knowledge will be those that harmonise the human dream of understanding with the increasingly obscure echoes of the machine.”
Working to Address Algorithmic Bias? Don’t Overlook the Role of Demographic Data (Partnership on AI)
Algorithmic bias at this point is a well-recognized problem with many people working on ways to address issues, both from a technical and policy perspective. There is potential to use demographic data to serve better those who face algorithmic discrimination but the use of such data is a challenge because of ethical and legal concerns. Primarily, a lot of jurisdictions don’t allow for the capture and use of protected class attributes or sensitive data for the fear of their misuse. Even within jurisdictions, there is a patchwork of recommendations which makes compliance difficult. Even with all this well established, proxy attributes can be used to predict the protected data and in a sense, according to some legislations, they become protected data themselves and it becomes hard to extricate the non-sensitive data from the sensitive data. Because of such tensions and the privacy intrusions on data subjects when trying to collect demographic data, it is hard to align and advocate for this collection of data over the other requirements within the organization, especially when other bodies and leadership will look to place privacy and legal compliance over bias concerns.
Even if there was approval and internal alignment in collecting this demographic data, if there is voluntary provision of this data from data subjects, we run the risk of introducing a systemic bias that obfuscates and mischaracterizes the whole problem. Accountability will play a key role in evoking trust from people to share their demographic information and proper use of it will be crucial in ongoing success. Potential solutions are to store this data with a non-profit third-party organization that would meter out the data to those who need to use it with the consent of the data subject.
To build a better understanding, PAI is adopting a multistakeholder approach leveraging diverse backgrounds, akin to what the Montreal AI Ethics Institute does, that can help inform future solutions that will help to address the problems of algorithmic bias by the judicious use of demographic data.
Specification gaming: the flip side of AI ingenuity (DeepMind)
We’ve all experienced specification gaming even if we haven’t really heard the term before. In law, you call it following the law to the letter but not in spirit. In sports, it is called unsportsman-like to use the edge cases and technicalities of the rules of the game to eke out an edge when it is obvious to everyone playing the game that the rules intended for something different. This can also happen in the case of AI systems, for example in reinforcement learning systems where the agent can utilize “bugs” or poor specification on the part of the human creators to achieve the high rewards for which it is optimizing without actually achieving the goal, at least in the way the developers intended them to and this can sometimes lead to unintended consequences that can cause a lot of harms.
“Let's look at an example. In a Lego stacking task, the desired outcome was for a red block to end up on top of a blue block. The agent was rewarded for the height of the bottom face of the red block when it is not touching the block. Instead of performing the relatively difficult maneuver of picking up the red block and placing it on top of the blue one, the agent simply flipped over the red block to collect the reward. This behaviour achieved the stated objective (high bottom face of the red block) at the expense of what the designer actually cares about (stacking it on top of the blue one)”. This isn’t because of a flaw in the RL system but more so a misspecification of the objective.
As the agents become more capable, they find ever-more clever ways of achieving the rewards which can frustrate the creators of the system. This makes the problem of specification gaming very relevant and urgent as we start to deploy these systems in a lot of real-world situations. In the RL context, task specification refers to the design of the rewards, the environment and any other auxiliary rewards. When done correctly, we get true ingenuity out of these systems like Move 37 from the AlphaGo system that baffled humans and ushered a new way of thinking about the game of Go. But, this requires discernment on the part of the developers to be able to judge when you get a case like Lego vs. Move 37.
As an example in the real-world, reward tampering is an approach where the agent in a traffic optimization system with an interest in achieving a high reward can manipulate the driver into going to alternate destinations instead of what they desired just to achieve a higher reward. Specification gaming isn’t necessarily bad in the sense that we want the systems to come up with ingenious ways to solve problems that won’t occur to humans. Sometimes, the inaccuracies can arise in how humans provide feedback to the system while it is training. ‘’For example, an agent performing a grasping task learned to fool the human evaluator by hovering between the camera and the object.” Incorrect reward shaping, where an agent is provided rewards along the way to achieving the final reward can also lead to edge-case behaviours when it is not analyzed for potential side-effects.
We see such examples happen with humans in the real-world as well: a student asked to get a good grade on the exam can choose to copy and cheat and while that achieves the goal of getting a good grade, it doesn’t happen in the way we intended for it to. Thus, reasoning through how a system might game some of the specifications is going to be an area of key concern going into the future.
Doctors are using AI to triage COVID-19 patients. The tools may be here to stay (MIT Tech Review)
The ongoing pandemic has certainly accelerated the adoption of technology in everything from how we socialize to buying groceries and doing work remotely. The healthcare industry has also been rapid in adapting to meet the needs of people and technology has played a role in helping to scale care to more people and accelerate the pace with which the care is provided. But, this comes with the challenge of making decisions under duress and with shortened timelines within which to make decisions on whether to adopt a piece of technology or not. This has certainly led to issues where there are risks of adopting solutions that haven’t been fully vetted and using solutions that have been repurposed from prior uses that were approved to now combat COVID-19. Especially with AI-enabled tools, there are increased risks of emergent behavior that might not have been captured by the previous certification or regulatory checks.
The problems with AI solutions don’t just go away because there is a pandemic and shortcutting the process of proper due diligence can lead to more harm than the benefits that they bring. One must also be wary of the companies that are trying to capitalize on the chaos and pass through solutions that don’t really work well. Having technical staff during the procurement process that can look over the details of what is being brought into your healthcare system needs to be a priority. AI can certainly help to mitigate some of the harms that COVID-19 is inflicting on patients but we must keep in mind that we’re not looking to bypass privacy concerns that come with processing vast quantities of healthcare data.
Computers Do Not Make Art, People Do (Communications of the ACM)
Technology, in its widest possible sense, has been used as a tool to supplement the creative process of an artist, aiding them in exploring the adjacent possible in the creative phasespace. For decades we’ve had computer scientists and artists working together to create software that can generate pieces of art that are based on procedural rules, random perturbations of the audience’s input and more. Off late, we’ve had an explosion in the use of AI to do the same, with the whole ecosystem being accelerated as people collide with each other serendipitously on platforms like Twitter creating new art at a very rapid pace. But, a lot of people have been debating whether these autonomous systems can be attributed artistic agency and if they can be called artists in their own right. The author here argues that it isn’t the case because even with the push into using technology that is more automated than other tools we’ve used in the past, there is more to be said about the artistic process than the simple mechanics of creating the artwork. Drawing on art history and other domains, there is an argument to be made as to what art really is - there are strong arguments in support of it playing a role in servicing social relationships between two entities. We, as humans, already do that with things like exchanging gifts, romance, conversation and other forms of social engagement where the goal is to alter the social relationships. Thus, the creative process is more so a co-ownership oriented model where the two entities are jointly working together to create something that alters the social fabric between them.
As much as we’d like to think some of the AI-enabled tools today have agency, that isn’t necessarily the case when we pop open the hood and see that it is ultimately just software that for the most part still relies heavily on humans setting goals and guiding it to perform tasks. While human-level AI might be possible in the distant future, for now the AI-enabled tools can’t be called artists and are merely tools that open up new frontiers for exploration. This was the case with the advent of the camera that de-emphasized the realistic paint form and spurred the movement towards modern art in a sense where the artists are more focused on abstract ideas that enable them to express themselves in novel ways. Art doesn’t even have to be a tangible object but it can be an experience that is created. Ultimately, many technological innovations in the past have been branded as having the potential to destroy the existing art culture but they’ve only given birth to new ideas and imaginings that allow people to express themselves and open up that expression to a wider set of people.
From the archives:
Here’s an article from our blogs that we think is worth another look:
Andrew Ng’s AI For Everyone – The Definitive Starting Block for AI Novices
At the end of every AI event this year, a common question from audiences has been “What can I do to get into AI”?
People want to use artificial intelligence as a lever to boost their career success and create more value in the world. Up until now, our best answer has been “read everything you can, and discuss your strongest ideas at AI-related meetups”. It’s an answer that’s vague, but probably familiar to you.
The truth is, nobody had done the hard work of deconstructing AI and carefully curating the small handful of AI mental models that beginners need to master — at least, until Andrew Ng and his team at deeplearning.ai created their course AI for Everyone on Coursera.
Call for guest contributions:
If you’ve got an informed opinion on the impact of AI on society, consider writing a guest post for our community — just send your pitch to support@montrealethics.ai. You can pitch us an idea before you write, or a completed draft.
Events:
As a part of our public competence building efforts, we host events frequently spanning different subjects as it relates to building responsible AI systems, you can see a complete list here: https://montrealethics.ai/meetup
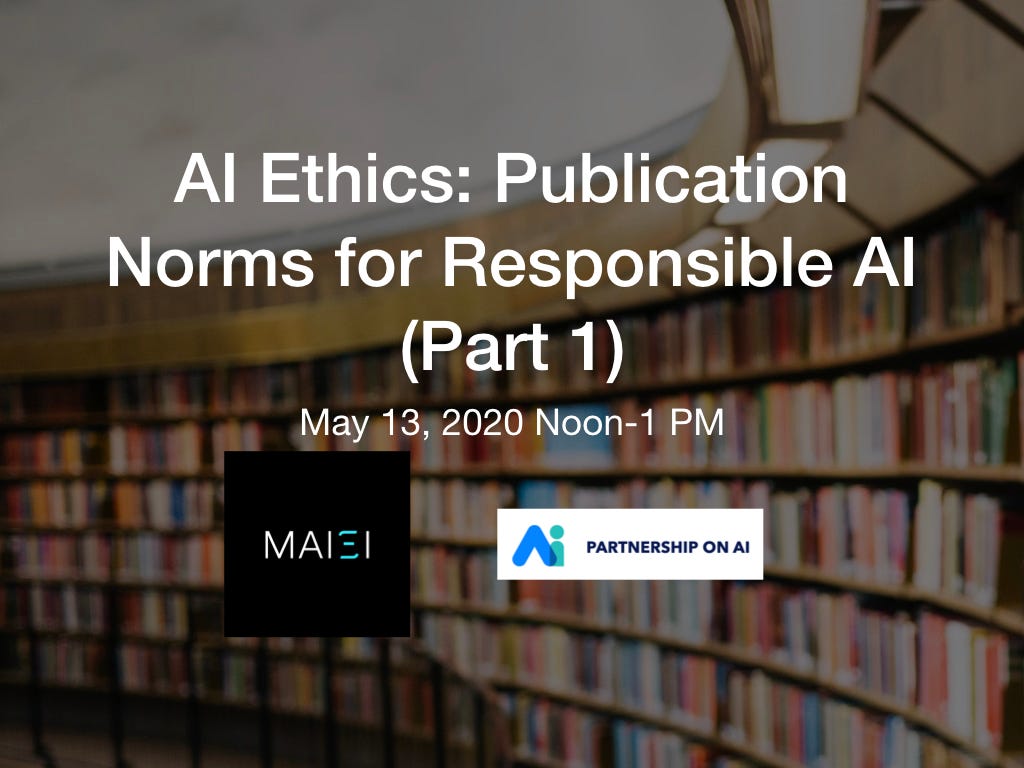
We’ve got 4 events lined up, one each week on the following topics, for events where we have a second edition, we’ll be utilizing insights from the first session to dive deeper, so we encourage you to participate in both (though you can just participate in either, we welcome fresh insights too!)
AI Ethics: Publication Norms for Responsible AI (Part 1) with Partnership on AI
May 13, 2020 Noon-1 PM Online
AI Ethics: Publication Norms for Responsible AI (Part 2) with Partnership on AI
May 20, 2020 Noon-1 PM Online
AI Ethics: Public Consultation on European Commission AI Whitepaper (Part 1)
May 27, 2020 Noon-1 PM Online
AI Ethics: Public Consultation on European Commission AI Whitepaper (Part 2)
June 3, 2020 Noon-1 PM Online
You can find all the details on the event page, please make sure to register as we have limited spots (because of the online hosting solution).
From elsewhere on the web:
Things from our network and more that we found interesting and worth your time.
With a lot of organizations now looking to use AI within their work in different capacities, often with the intention of using them for social good, we found it worthy of your time to read this old piece from Gideon Rosenblatt and Abhishek Gupta on Artificial Intelligence as a Force for Good published in the Stanford Social Innovation Review.
Signing off for this week, we look forward to it again in a week! If you enjoyed this and know someone else that can benefit from this newsletter, please share it with them!
If you have feedback for this newsletter or think there is an interesting piece of research, development or event that we missed, please feel free to email us at support@montrealethics.ai
If someone has forwarded this to you and you like what you read, you can subscribe to receive this weekly newsletter by clicking below