AI Ethics #9 : Defending ML systems, learning from Ubuntu, the "reputation age", boosting science against coronavirus misinformation, and more ...
Our ninth weekly edition covering research and news in the world of AI Ethics
Welcome to the ninth edition of our weekly newsletter that will help you navigate the fast changing world of AI Ethics! Every week we dive into research papers that caught our eye, sharing a summary of those with you and presenting our thoughts on how it links with other work in the research landscape. We also share brief thoughts on interesting articles and developments in the field. More about us on: https://montrealethics.ai/about/
If someone has forwarded this to you and you want to get one delivered to you every week, you can subscribe to receive this newsletter by clicking below
Our session on Publication Norms for Responsible AI was packed with insights from community members that joined us from many countries from around the world, we went into the depths of the questions trying to find answers to some of the thorniest challenges when it comes to publishing about high-stakes research.
“ … one important aspect in upholding ‘responsible AI’ is the consideration of when and how to publish novel research in a way that maximizes benefits while mitigating potential harms.” - Partnership on AI.
We’re working with Partnership on AI for this events and look forward to your participation! You can get your tickets from here. Even if you missed the first session, don’t worry as you’ll receive the notes from it in preparation for the session this coming week.
In research summaries this week, we dive into the idea of what the gaps in industry are today when it comes to defending against ML specific attacks and what we can learn from traditional cybersecurity. We also discuss a paper on how to capture diversity better in outcomes of algorithmic systems by leveraging subjective inputs from individuals themselves.
In article summaries, we talk about some techniques that will help boost accurate scientific information on the coronavirus in the face of misinformation, the role of reputation in today’s information age, how AI systems can be insured against malicious adversaries, the limits of AI in fighting the coronavirus, how AI could lend a helping hand to journalism, and what we can learn from Ubuntu to build more inclusive AI systems.
Our learning communities have received an overwhelming response! Thank you everyone!
We operate on the open learning concept where we have a collaborative syllabus on each of the focus areas and meet every two weeks to learn from our peers. We are starting with 5 communities focused on: disinformation, privacy, labor impacts of AI, machine learning security and complex systems theory. You can fill out this form to receive an invite!
Hoping you stay safe and healthy and looking forward to seeing you at our upcoming public consultation sessions (virtually!) and our learning communities! Enjoy this week’s content!
Research:
Let's look at some highlights of research papers that caught our attention at MAIEI:
Learning to Diversify from Human Judgments: Research Directions and Open Challenges by Denton et al.
Current algorithmic techniques frame the notion of diversity in the sense of using the presence of sensitive attributes in the result set as a measurement for whether there is sufficient representation. Yet, such an approach often ends up stripping these sensitive attributes, often gender and race from their deep social, culture and context specific meanings and bucket them into discrete categories that are rigid, uni-dimensional, and determined algorithmically in the process of clustering.
The paper presents a research direction using the concept of determinantal point process (DPP) as a mechanism for capturing diversity in a more subjective and individualized manner by taking in the feelings of the individuals on whether they think they are well represented in the result set or not. It tends to cluster together the things that the individual feels represents them well and further away from others that don’t in an embedding space. Relying on individual’s perceptions to tailor these representations moves applications a step forward in a direction where representation is adequately captured. The authors do identify challenges associated with this approach namely the reliable sourcing of this information in a large-scale manner, especially as it relates to the limitations of how crowdsourcing platforms are structured today but still gives the research community some food for thought in how to capture diversity better.
To delve deeper, read our full summary here.
Adversarial Machine Learning - Industry Perspectives by Kumar et al.
An emerging area of concern for companies that are seeing heavy deployments of ML systems in the industry is cybersecurity. There are many emergent risks that are a departure from traditional cybersecurity practice that need to be addressed when applying insights to the field of ML. The authors of this study surveyed two key personas: ML engineers who were responsible for the development and deployment of ML systems and security first incident responders. While a lot of them recognized the concerns raised by the authors, they didn’t have clarity in the mechanisms and techniques that they could deploy to secure the system against some of these potential threats. Most concerned themselves with data poisoning attacks on the systems and paid less attention to other areas such as model inversion, adversarial examples in the physical domains, and supply chain related vulnerabilities. Privacy breaches and intellectual property related thefts were at the top of the minds again because of their primacy in wider discussions thus focusing attention on a smaller subset of potential attacks which leaves some of the attack surface open.
The authors make a strong case for borrowing some of the best practices from the field of traditional cybersecurity like utilizing shared vulnerability databases, a common standard for scoring risks, and better secure coding practices as associated with the popular ML development frameworks. They also observed a certain degree of relegation of responsibility when it came to using ML as a service from cloud providers where downstream applications developers didn’t realize that some of the cybersecurity measures fell on their shoulders in terms of managing the risks. While there remain many challenges in successfully replicating the state of cybersecurity and improving on it from traditional software infrastructure, given that ML is the new software and there is increasing adoption, the authors call on the community to start paying serious attention to the concerns raised and building up capabilities to manage and combat the risks posed by cybersecurity threats that are geared towards the ML aspects of the larger software industry.
To delve deeper, read our full summary here.
Articles:
Let’s look at highlights of some recent articles that we found interesting at MAIEI:
Going viral: how to boost the spread of coronavirus science on social media (Nature)
The WHO has mentioned the infodemic as being one of the causes that is exacerbating the pandemic as people follow differing advice on what to do. Communication by authorities has been persistent but at times ineffective and this article dives into how one could enhance the visibility of credible information by governments, health authorities and scientists so that the negative impacts of the infodemic can be curbed. But, spewing scientific facts from a soapbox alone isn’t enough — one is competing with all the other pieces of information and entertainment for attention and that needs to be taken into account. One of the key findings is that starting a dialogue helps more than just sending a one-way communiqué. Good science communication relies on the pillars of storytelling, cutting through the jargon and making the knowledge accessible.
While online platforms are structured such that polarization is encouraged through the algorithmic underpinnings of the system, we should not only engage when there is something that we disagree with, instead taking the time to amplify good science is equally important. Using platform-appropriate messaging, tailoring content to the audience and not squabbling over petty details, especially when they don’t make a significant impact on the overall content helps to push out good science signals in the ocean of information pollution.
Clickbait-style headlines do a great job of hooking in people but when leading people into making a certain assumption and then debunking it, you stand the risk of spreading misinformation if someone doesn’t read the whole thing, so in trying to make headlines engaging, it is important to consider what might be some unintended consequences if someone didn’t read past the subtitle. Science isn’t just about the findings, the process only gets completed when we have effective communication to the larger audience of the results, and now more than ever, we need accurate information to overpower the pool of misinformation out there.
Say goodbye to the information age: it’s all about reputation now (Aeon)
There isn’t a dearth of information available online, one can find confirmatory evidence to almost any viewpoint since the creation and dissemination of information has been democratized by the proliferation of the internet and ease of use of mass-media platforms. So in the deluge of information, what is the key currency that helps us sift through all the noise and identify the signal? This article lays out a well articulated argument for how reputation and being able to assess it is going to be a key skill that people will need to have in order to effectively navigate the information ecosystem effectively. We increasingly rely on other people’s judgement of content (akin to how MAIEI analyzes the ecosystem of AI ethics and presents you with a selection), coupled with algorithmically-mediated distribution channels, we are paradoxically disempowered by more information and paralyzed into inaction and confusion without a reputable source to curate and guide us.
There are many conspiracy theories, famous among them that we never visited the Moon, Flat Earth and more recently that 5G is causing the spread of the coronavirus. As rational readers, we tend to dismiss this as misinformation yet we don’t really spend time to analyze the evidence that these people present to support their claims. To a certain extent, our belief that we did land on the Moon depends on our trust in NASA and other news agencies that covered this event yet we don’t venture to examine the evidence first-hand. More so, with highly specialized knowledge becoming the norm, we don’t have the right tools and skills to even be able to analyze the evidence and come to meaningful conclusions. So, we must rely on those who provide us with this information. Instead of analyzing the veracity of a piece of information, the focus of a mature digital citizen needs to be on being able to analyze the reputation pathway of that information, evaluate the agendas of the people that are disseminating the information and critically analyze the intentions of the authorities of the sources.
How we rank different pieces of information arriving to us via our social networks need to be appraised for this reputation and source tracing, in a sense a second-order epistemology is what we need to prepare people for. In the words of Hayek, “civilization rests on the fact that we all benefit from the knowledge that we do not possess.” Our cyber-world can become civilized by evaluating this knowledge that we don’t possess critically when mis/disinformation can spread just as easily as accurate information.
The Case for AI Insurance (Harvard Business Review)
In the age of adversarial machine learning (MAIEI has a learning community on machine learning security if you’d like to learn more about this area) there are enormous concerns with protecting software infrastructure as ML opens up a new attack surface and new vectors which are seldom explored. From the perspective of insurance, there are gaps in terms of what cyber-insurance covers today, most of it being limited to the leakage of private data. There are two kinds of attacks that are possible on ML systems: intentional and unintentional. Intentional attacks are those that are executed by malicious agents who attempt to steal the models, infer private data or get the AI system to behave in a way that favors their end goals. For example, when Tumblr decided to not host pornographic content, creators bypassed that by using green screens and pictures of owls to fool the automated content moderation system. Unintended attacks can happen when the goals of the system are misaligned with what the creators of the system actually intended, for example, the problem of specification gaming, something that Abhishek Gupta discussed here in this Fortune article.
In interviewing several officers in different Fortune 500 companies, the authors found that there are 3 key problems in this domain at the moment: the defenses provided by the technical community have limited efficacy, existing copyright, product liability, and anti-hacking laws are insufficient to capture AI failure modes. Lastly, given that this happens at a software level, cyber-insurance might seem to be the way to go, yet current offerings only cover a patchwork of the problems.
Business interruptions and privacy leaks are covered today under cyber-insurance but other problems like bodily harm, brand damage, and property damage are for the most part not covered. In the case of model recreation, as was the case with the OpenAI GPT-2 model, prior to it being released, it was replicated by external researchers - this might be covered under cyber-insurance because of the leak of private information. Researchers have also managed to steal information from facial recognition databases using sample images and names which might also be covered under existing policies.
But, in the case with Uber where there was bodily harm because of the self-driving vehicle that wasn’t able to detect the pedestrian accurately or similar harms that might arise if conditions are foggy, snowy, dull lighting, or any other out-of-distribution scenarios, these are not adequately covered under existing insurance terms. Brand damage that might arise from poisoning attacks like the case with the Tay chatbot or confounding anti-virus systems as was the case with an attack mounted against the Cylance system, cyber-insurance falls woefully short in being able to cover these scenarios. In a hypothetical situation as presented in a Google paper on RL agents where a cleaning robot sticks a wet mop into an electric socket, material damage that occurs from that might also be considered out of scope in cyber-insurance policies.
Traditional software attacks are known unknowns but adversarial ML attacks are unknown unknowns and hence harder to guard against. Current pricing reflects this uncertainty, but as the AI insurance market matures and there is a deeper understanding for what the risks are and how companies can mitigate the downsides, the pricing should become more reflective of the actual risks. The authors also offer some recommendations on how to prepare the organization for these risks - for example by appointing an officer that works closely with the CISO and chief data protection officer, performing table-top exercises to gain an understanding of potential places where the system might fail and evaluating the system for risks and gaps following guidelines as put forth in the EU Trustworthy AI guidelines.
Artificial Intelligence Won't Save Us From Coronavirus (Wired)
The push has been to apply AI to any new problem that we face, hoping that the solution will magically emerge from the application of the technique as if it is a dark art. Yet, the more seasoned scientists have seen these waves come and go and in the past, a blind trust in this technology led to AI winters. Taking a look at some of the canaries in the coal mine, the author cautions that there might be a way to judge whether AI will be helpful with the pandemic situation. Specifically, looking at whether domain experts, like leading epidemiologists endorse its use and are involved in the process of developing and utilizing these tools will give an indication as to whether they will be successful or not. Data about the pandemic depends on context and without domain expertise, one has to make a lot of assumptions which might be unfounded. All models have to make assumptions to simplify reality, but if those assumptions are rooted in domain expertise from the field then the model can mimic reality much better.
Without context, AI models assume that the truth can be gleaned solely from the data, which though it can lead to surprising and hidden insights, at times requires humans to evaluate the interpretations to make meaning from them and apply them to solve real-world problems. This was demonstrated with the case where it was claimed that Ai had helped to predict the start of the outbreak, yet the anomaly required the analysis from a human before arriving at that conclusion.
Claims of extremely high accuracy rates will give hardened data scientists reason for caution, especially when moving from lab to real-world settings as there is a lot more messiness with real-world data and often you encounter out-of-distribution data which hinders the ability of the model to make accurate predictions. For CT scans, even if they are sped up tremendously by the use of AI, doctors point out that there are other associated procedures such as the cleaning and filtration and recycling of air in the room before the next patient can be passed through the machine which can dwindle the gains from the use of an unexplainable AI system analyzing the scans. Concerns with the use of automated temperature scanning using thermal cameras also suffers from similar concerns where there are other confounding factors like the ambient temperature, humidity, etc. which can limit the accuracy of such a system. Ultimately, while AI can provide tremendous benefits, we mustn’t blindly be driven by its allure to magically solve the toughest challenges that we face.
How artificial intelligence can save journalism (The Conversation)
While the previous summary gives us some caution when thinking about how AI might be applied to different industries, there is a potential for AI to automate repetitive tasks and free up scarce resources towards more value-added tasks. With a declining business model and tough revenue situations, newsrooms and journalism at large are facing an existential crisis. Cutting costs while still keeping up high standards of reporting will require innovation on the part of newsrooms to adapt emerging technologies like AI. For example, routine tasks like reporting on sports scores from games and giving updates on company earnings calls is already something that is being done by AI systems in several newsrooms around the world. This frees up time for journalists to spend their efforts on things like long-form journalism, data-driven and investigative journalism, analysis and feature pieces which require human depth and creativity. Machine translation also offers a handy tool making the work of journalists accessible to a wider audience without them having to invest in a lot of resources to do the translations themselves. This also brings up the possibility of smaller and resource-constrained media rooms to use their limited resources for doing in-depth pieces while reaching a wider audience by relying on automation.
Transcription of audio interviews so that reporters can work on fact-checking and other associated pieces also helps bring stories to fruition faster, which can be a boon in the rapidly changing environment. In the case of evolving situations like the pandemic, there is also the possibility of using AI to parse through large reams of data to find anomalies and alert the journalist of potential areas to cover. Complementing human skills is the right way to adopt AI rather than thinking of it as the tool that replaces human labor.
Q&A: Sabelo Mhlambi on what AI can learn from Ubuntu ethics (People + AI Research)
Offering an interesting take on how to shape the development and deployment of AI technologies, Mhlambi utilizes the philosophy of Ubuntu as a guiding light in how to build AI systems that better empower people and communities. The current Western view that dominates how AI systems are constructed today and how they optimize for efficiency is something that lends itself quite naturally to inequitable outcomes and reinforcing power asymmetries and other imbalances in society. Embracing the Ubuntu mindset which puts people and communities first stands in contrast to this way of thinking. It gives us an alternative conception of personhood and has the potential to surface some different results. While being thousands of years old, the concept has been seen in practice over and over again, for example, in South Africa, after the end of the apartheid, the Truth and Reconciliation program forgave and integrated offenders back into society rather than embark on a Kantian or retributive path to justice. This restorative mindset to justice helped the country heal more quickly because the philosophy of Ubuntu advocates that all people are interconnected and healing only happens when everyone is able to move together in a harmonious manner.
This was also seen in the aftermath of the Rwanda genocide, where oppressors were reintegrated back into society often living next to the people that they had hurt; Ubuntu believes that no one is beyond redemption and everyone deserves the right to have their dignity restored. Bringing people together through community is important, restorative justice is a mechanism that makes the community stronger in the long run. Current AI formulation seeks to find some ground truth but thinking of this in the way of Ubuntu means that we try to find meaning and purpose for these systems through the values and beliefs that are held by the community. Ubuntu has a core focus on equity and empowerment for all and thus the process of development is slow but valuing people above material efficiency is more preferable than speeding through without thinking of the consequences that it might have on people. Living up to Ubuntu means offering people the choice for what they want and need, rooting out power imbalances and envisioning the companies as a part of the communities for which they are building products and services which makes them accountable and committed to the community in empowering them.
From the archives:
Here’s an article from our blogs that we think is worth another look:
Probing Networked Agency: Where is the Locus of Moral Responsibility? by Audrey Balogh (Philosophy, McGill University)
This paper problematizes the case for autonomous robots as loci of moral responsibility in circuits of networked agency, namely by troubling an analogy drawn between canine and machine in John P. Sullins’ paper ‘When Is a Robot a Moral Agent?”. It will also explore the pragmatic implications of affording these machines a morally responsible designation in contexts of law and policy.
Guest contributions:
AI Economist: Reinforcement Learning is the Future for Equitable Economic Policy by Richard Socher and Stephan Zheng
Long before pandemic-related lockdowns, economic inequality has been one of the most significant issues affecting humanity. A report from the United Nations in January 2020 found that inequality is rising in most of the developed world. With so much to lose or gain, it’s no surprise that bias can influence policymaking… often to the detriment those who need the most help. This underscores the potential that AI can have for good, and why it’s important to develop tools and solutions that are simulation- and data-driven to yield more equitable policies.
The new AI Economist model from Salesforce Research is designed to address this kind of equality by identifying an optimal tax policy. By using a two-level reinforcement learning (RL) framework, training both (1) AI agents and (2) tax policies, it simulates and helps identify dynamic tax policies that best accomplish a given objective. This RL framework is model-free in that it uses zero prior world knowledge or modeling assumptions, and learns from observable data alone.
Richard Socher and Stephan Zheng penned a piece for MAIEI, you can read it here.
If you’ve got an informed opinion on the impact of AI on society, consider writing a guest post for our community — just send your pitch to support@montrealethics.ai. You can pitch us an idea before you write, or a completed draft.
Events:
As a part of our public competence building efforts, we host events frequently spanning different subjects as it relates to building responsible AI systems, you can see a complete list here: https://montrealethics.ai/meetup
We’ve got 3 events lined up, one each week on the following topics, for events where we have a second edition, we’ll be utilizing insights from the first session to dive deeper, so we encourage you to participate in both (though you can just participate in either, we welcome fresh insights too!)
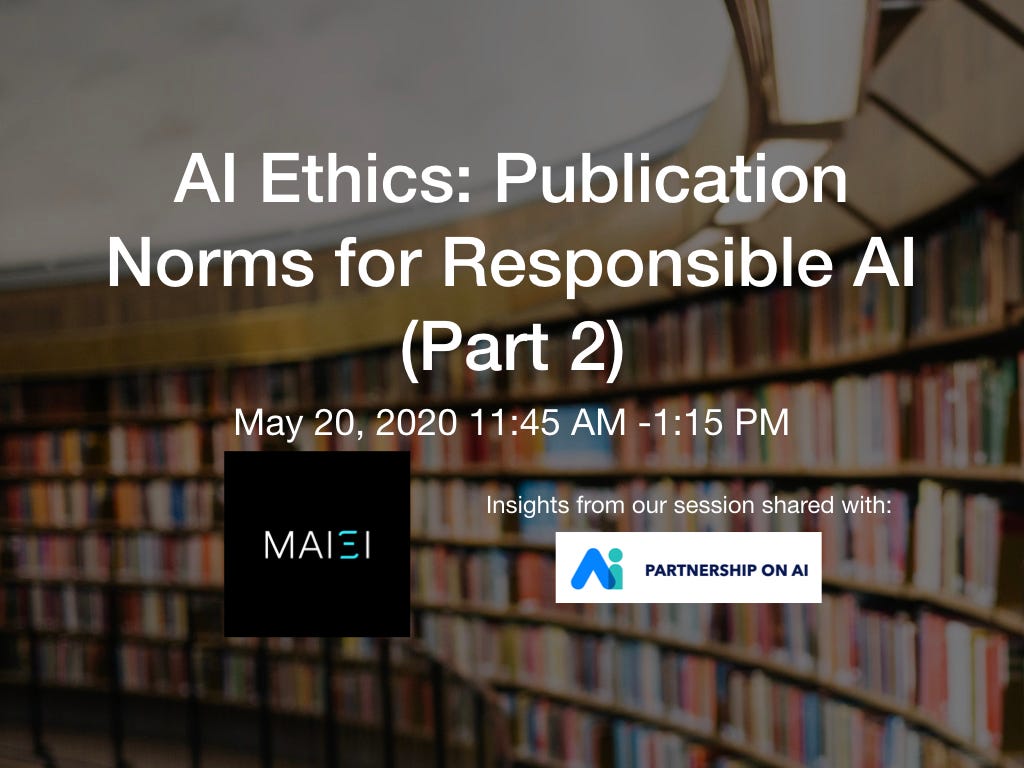
AI Ethics: Publication Norms for Responsible AI (Part 2) with Partnership on AI
May 20, 2020 11:45 AM -1:15 PM Online
AI Ethics: Public Consultation on European Commission AI Whitepaper (Part 1)
May 27, 2020 11:45 AM -1:15 PM Online
AI Ethics: Public Consultation on European Commission AI Whitepaper (Part 2)
June 3, 2020 11:45 AM -1:15 PM Online
You can find all the details on the event page, please make sure to register as we have limited spots (because of the online hosting solution).
From elsewhere on the web:
Things from our network and more that we found interesting and worth your time.
A.I. engineers should spend time training not just algorithms, but also the humans who use them by Jeremy Kahn from Fortune
Our founder Abhishek Gupta was featured by Jeremy Kahn in Fortune where he detailed his views on AI safety concerns in RL systems, the “token human” problem, and automation surprise among other points to pay attention to when developing and deploying AI systems. Especially in situations where these systems are going to be used in critical scenarios, humans operating in tandem with these systems and utilizing them as decision inputs need to gain a deeper understanding of the inherent probabilistic nature of the predictions from these systems and make decisions that take it into consideration rather than blindly trusting recommendations from an AI system because they have been accurate in 99% of the scenarios.
Signing off for this week, we look forward to it again in a week! If you enjoyed this and know someone else that can benefit from this newsletter, please share it with them!
If you have feedback for this newsletter or think there is an interesting piece of research, development or event that we missed, please feel free to email us at support@montrealethics.ai
If someone has forwarded this to you and you like what you read, you can subscribe to receive this weekly newsletter by clicking below