AI Ethics #12 : Citizen councils evaluating AI, lexicon of lies, future of work in Canada, AI governance, limitations of AI systems, success metrics in AI, and more ...
Our twelfth weekly edition covering research and news in the world of AI Ethics
Welcome to the twelfth edition of our weekly newsletter that will help you navigate the fast changing world of AI Ethics! Every week we dive into research papers that caught our eye, sharing a summary of those with you and presenting our thoughts on how it links with other work in the research landscape. We also share brief thoughts on interesting articles and developments in the field. More about us on: https://montrealethics.ai/about/
If someone has forwarded this to you and you want to get one delivered to you every week, you can subscribe to receive this newsletter by clicking below
These past few weeks, our team worked around the clock to complete our analysis of the European Commission’s AI Whitepaper and supplemented it with the insights from our community and produced this report which we have submitted to the EC, some of the recommendations that we made are as follows:
Focus on mechanisms that promote private and secure sharing of data in the building up of the European data space, leveraging technical advances like federated learning, differential privacy, federated analytics, and homomorphic encryption.
Add nuance to the discussion regarding the opacity of AI systems, so that there is a graduated approach to how these systems are governed and in which place there is a requirement for what degree of explainability and transparency.
Appoint individuals to the human oversight process who understand the AI systems well and are able to communicate any potential risks effectively with a variety of stakeholders so that they can take the appropriate action.
To delve deeper, please read the entire report here.
We have launched some new initiatives at MAIEI that are aimed at increasing scientific diversity, if you are not already a part of our Slack community this is a great time to join in!
In research summaries this week, we take a look at the future of work in Canada, an overview of AI governance, benefit-risk analysis of machine learning models, a lexicon of lies to better assess false information online, and an analysis of information operations in the context of the BLM discourse.
In article summaries, we covered the limitations of AI systems today, how a citizen council could help to evaluate algorithms, and the impact that Instagram’s policies have on the behaviour of its user base.
Our learning communities continue to receive an overwhelming response! Thank you everyone!
We operate on the open learning concept where we have a collaborative syllabus on each of the focus areas and meet every two weeks to learn from our peers. You can fill out this form to receive an invite!
Hoping you stay safe and healthy and looking forward to seeing you at our upcoming public consultation sessions (virtually!) and our learning communities! Enjoy this week’s content!
Research:
Let's look at some highlights of research papers that caught our attention at MAIEI:
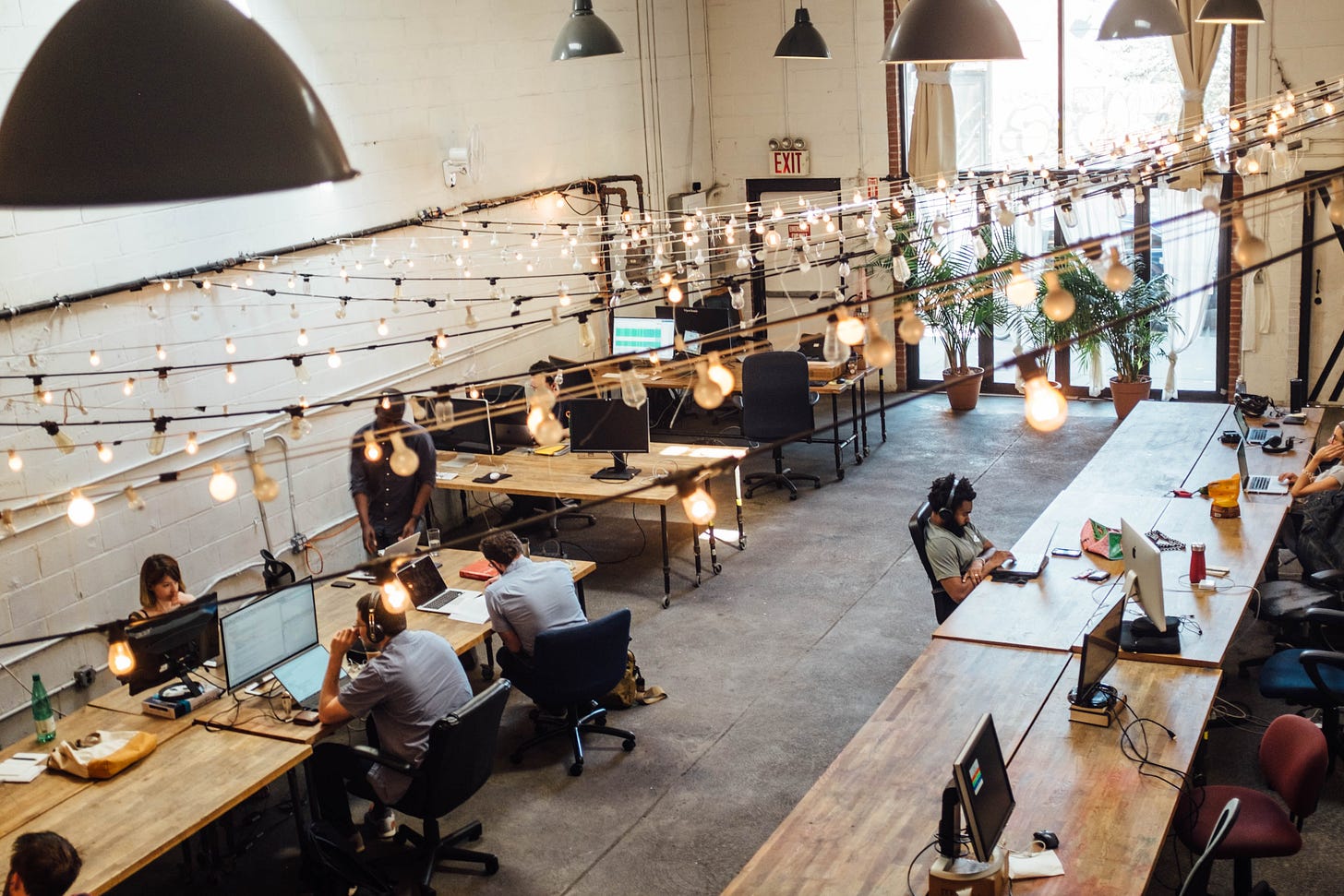
Photo by Shridhar Gupta on Unsplash
On the Edge of Tomorrow: Canada’s AI Augmented Workforce by Ryan McLaughlin and Trevor Quan
Following the 2008 financial crisis, the pursuit of economic growth and prosperity led many companies to pivot from labor intensive to capital intensive business models with the espousal of AI technology. The capitalist’s case for AI centered on potential gains in labor productivity and labor supply. The demand for AI grew with increased affordability of sensors, accessibility of big data and a growth of computational power. Although the technology has already augmented various industries, it has also adversely impacted the workforce. Not to mention, the data, which powers AI, can be collected and used in ways that put fundamental civil liberties at risk. Due to Canada’s global reputation and extensive AI talent, the ICTC recommends Canada take a leadership role in the ethical deployment of this technology.
To delve deeper, read our full summary here.
AI Governance in 2019, A Year in Review: Observations of 50 Global Experts by SHI Qian (Editor-in-Chief), Li Hui (Executive Editor), Brian Tse (Executive Editor)
2019 has seen a sharp rise in interest surrounding AI Governance. This is a welcome addition to the lasting buzz surrounding AI and AI Ethics, especially if we are to collectively build AI that enriches people’s lives.
The AI Governance in 2019 report presents 44 short articles written by 50 international experts in the fields of AI, AI Ethics, and AI Policy. Each article highlights, from its author’s or authors’ point of view, the salient events in the field of AI Governance in 2019. Apart from the thought-provoking insights it contains, this report also offers a great way for individuals to familiarize themselves with the experts contributing to AI governance internationally, as well as with the numerous research centers, think tanks, and organizations involved.
To delve deeper, read our full summary here.
Model Benefit-Risk Analysis by the Future of Privacy Forum
Data transparency is a key goal of the open data movement, and as different federal and municipal governments create open data policies, it’s important that they take into account the risks to individual privacy that come with sharing data publicly. In order to ensure open data privacy, open data managers and departmental data owners within governments need a standardized methodology to assess the privacy risks and benefits of a dataset. This methodology is a valuable component of building what the Future of Privacy Forum (FPF) calls a “mature open data program.”
In their City of Seattle Open Data Risk Assessment report, the FPF presents a Model Benefit-Risk Analysis that can be utilized to evaluate datasets and determine whether or not they should be published openly. This analysis is based on work by the National Institute of Standards and Technology, the University of Washington, the Berkman Klein Center, and the City of San Francisco.
To delve deeper, read our full summary here.
From our learning communities:
Research that we covered in the learning communities at MAIEI this week, summarized for ease of reading:
Lexicon of Lies: Terms for Problematic Information by Caroline Jack
This article seeks to explain the terms used to describe problematic information. They could be inaccurate, misleading, or altogether fabricated. The terms we use in describing information would impact how information spreads, who spreads it, and who receives it as this choice is based solely on the perspective of the descriptor. This makes the labelling of information complex, inconsistent and imprecise.
To delve deeper, read our full summary here.
Acting the Part: Examining Information Operations Within #BlackLivesMatter Discourse by Arif, A., Stewart, L. G., & Starbird, K.
The researchers at the University of Washington analyzed Twitter activity on the #BlackLivesMatter movement and police-related shootings in the United States during 2016 to better understand how information campaigns manipulate the social media discussions taking place. They focused on publicly suspended accounts that were affiliated with the Internet Research Agency, a Russian organization. This organization supports full-time employees to do “professional propaganda” on social media.
Social media has become a platform for information operations, especially for foreign invaders, to alter the infrastructure and spread disinformation. Information operations are used by the United States intelligence community that describes actions that disrupt information systems and streams of geopolitical adversary.
To delve deeper, read our full summary here.
Articles:
Let’s look at highlights of some recent articles that we found interesting at MAIEI:
An understanding of AI’s limitations is starting to sink in (The Economist)
Whispers in terms of limitations of the approaches to achieving the “intelligence” in AI have been abounding for a while. Specifically, over the last few years AI has become a buzzword and more companies claim to use AI than actually do (see here for our coverage on that last week) trying to garner investment and media interest. We acknowledge here that the definition of intelligence itself is quite open to interpretation and everyone has different expectations in terms of what these systems should be capable of achieving to be called intelligent. Yet, in some scenarios where we have seen stellar progress, decades ahead of what experts had thought possible, for example with AlphaGo, there are still scenarios where the intelligence exhibited by these systems is quite limited even within a narrow domain where the system starts to fall apart when presented with data that falls outside of the distribution it expects.
The fundamental promise of AI is that it is great at identifying patterns which makes it a general-purpose technology that has wide applicability across every possible domain. As an example, with the ongoing pandemic, there is a strong case being made for using AI in every part of the viral management lifecycle from drug discovery, inventory management, allocating resources, and more. Especially as it relates to the use of AI in contact-tracing, a lot of promises have been made, but pulling open the hood points to a host of problems that don’t yet have clear answers, as pointed out in this analysis done by the Montreal AI Ethics Institute. This is supplemented also by the views from Eric Topol who says that advances in the hype of AI have far outpaced that in the science of AI. In the past this has led to “AI Winters” which had a tremendously negative impact on the field. While this time around there has been a lot of actual and positive deployment of AI systems, it hasn’t been without a great deal of ethics, safety, and inclusivity issues. One of the themes explored in the work by the Montreal AI Ethics Institute looks at how the large-scale of models and the data requirements make such models inaccessible and deepen inequities in the economy.
Given that a lot of consulting firms make predictions that the widespread use of AI will add trillions of dollars worth of economic value and output to the global economy. Yet, a more realistic view given the current capabilities and speaking with technical experts working in the field brings a dampener on such extravagant claims made by firms that put out such reports.
A Council of Citizens Should Regulate Algorithms (Wired)
One of the phrases used in the article really caught our attention, the serendipitous discovery and reporting of ethical, safety, and inclusivity concerns in the development and use of AI systems. The current responsible AI ecosystem is fragmented and given a whole host of other issues going on in the world right now (read: pandemic!) some issues fade in and out of focus. The problem with that is that while concerns with Big Tech had finally started to reach a crest towards meaningful action at the end of 2019, it seems that given how essential some of the services offered by these firms are, there has been a weak abandonment of talking about some concerns. We note that the recent pullback of facial recognition systems by large providers stands as a counterpoint to this argument, instead we want to emphasize that it was incessant efforts by Joy Buolamwini, Timnit Gebru, and others who pushed for reform in this domain that led to some of the actions taken by companies this past week. We strongly advocate for citizen empowerment as a vehicle for making organizations more accountable in the deployment of AI systems that conform with the values and norms of the local communities. The Montreal AI Ethics Institute has been doing work in this space of engaging citizens in conversations around this through workshops since its inception and seeks to continue doing so in the future.
Pointing to examples from Athenian democracy models, there are certainly precedents in terms of attempting inclusive and pluralistic democratic instruments that can be leveraged to tap into diverse, on-the-ground, citizen expertise that has the promise to find novel ways of addressing problems faced by a society, with the caveat that there were scores of citizens that were excluded from this because of their race, gender, property ownership, etc. but overcoming those shortcomings and including everyday voices in these conversations is quite important because the knowledge that the participants gain creates local champions who are then able to take this back into their communities.
Ultimately, this is a mechanism to not only find creative solutions to hard problems but also equip and empower local communities with the skills and tools to become self-sufficient in governing the creation and use of AI systems.
Undress or fail: Instagram’s algorithm strong-arms users into showing skin (AlgorithmWatch)
The degree to which platforms govern the ecosystem and the impact that they have in shaping the wider ecosystem is evident in this experiment that was carried out by the team at AlgorithmWatch on Instagram users. In trying to ascertain if the platform as a whole had a slant towards a particular kind of content, the team from AlgorithmWatch requested volunteers to install a browser plug-in that monitored the content that was shown to the users in their feed. The team then analyzed the distribution of the types of content in the feeds of these users and found that while personalization based on tastes of users, as expressed by their interactions with content on the platform and past behaviour, factored into what was shown, there was a skew towards content that favored the showing of skin.
Overall, this has had a negative impact on the community for those whose values don’t align with this and those whose businesses have nothing to do with things like swimwear, etc. and from anecdotal evidence collected by the team, they found that creators who ran accounts for things like food blogs also had to resort to these tactics to have their content feature prominently in the feeds of people. The AlgorithmWatch team reached out to the Facebook (owns Instagram) team for comment and they replied saying that the methodology used by the AlgorithmWatch team was flawed.
One of the patents filed in this space by them specifically identified “gender, ethnicity, and “state of undress” could be used in computing the engagement metric which would be used to present items in a person’s feed. Additionally, the items are presented not just based on the prior actions of that user but all users which can lead to a collective shaping of behaviour on the platform. Bias can naturally creep in when supervised computer vision techniques are used to train systems to automatically categorize content and then present it to the users, for example, in using popular crowdsourced platforms to label training data, there is a risk that categorization is done in the coarsest possible manner because of the low rates of pay to users which lowers the amount of effort that workers potentially exert in finding more nuanced categories.
A problem with content creators speaking out is that they fear the “shadow-ban”, a practice exercised by platform owners that pushes down the content from those facing that ban into the depths of an ethereal abyss such that their content doesn’t show up prominently in people’s feeds even when that piece of content might be relevant to that user.
From the archives:
Here’s an article from our blogs that we think is worth another look:
Different Intelligibility for Different Folks by Yishan Zhou and David Danks
Intelligibility is a notion that is worked on by a lot of people in the technical community who seek to shed a light on the inner workings of systems that are becoming more and more complex. Especially in the domains of medicine, warfare, credit allocation, judicial systems and other areas where they have the potential to impact human lives in significant ways, we seek to create explanations that might illuminate how the system works and address potential issues of bias and fairness.
However, there is a large problem in the current approach in the sense that there isn’t enough being done to meet the needs of a diverse set of stakeholders who require different kinds of intelligibility that is understandable to them and helps them meet their needs and goals. One might argue that a deeply technical explanation ought to suffice and others kinds of explanations might be derived from that but it makes them inaccessible to those who can’t parse well the technical details, often those who are the most impacted by such systems. This paper by Yishan Zhou and David Danks offers a framework to situate the different kinds of explanations such that they are able to meet the stakeholders where they are at and provide explanations that not only help them meet their needs but ultimately engender a higher level of trust from them by highlighting better both the capabilities and limitations of the systems.
To delve deeper, read the full article here.
Guest contributions:
A call for a critical look at the metrics for success in the evaluation of AI by Bogdana Rakova
The IEEE Global Initiative on Ethics of Autonomous and Intelligent Systems (A/IS) is a community of experts collaborating on comprehensive, crowd-sourced, and transdisciplinary efforts that aim to contribute to better alignment between AI-enabled technology and society’s broader values. The collaborative efforts of these experts in their work on Ethically Aligned Design [1] has given rise to 15 working groups dedicated to specific aspects of the ethics of A/IS. P7010 is one of these working groups which has published the 7010-2020 – IEEE Recommended Practice for Assessing the Impact of Autonomous and Intelligent Systems on Human Well-Being. It puts forth “wellbeing metrics relating to human factors directly affected by intelligent and autonomous systems and establishes a baseline for the types of objective and subjective data these systems should analyze and include (in their programming and functioning) to proactively increase human wellbeing” [2]. The impact assessment proposed by the group could provide practical guidance and strategies that “enable programmers, engineers, technologists and business managers to better consider how the products and services they create can increase human well-being based on a wider spectrum of measures than economic growth and productivity alone” [3].
To delve deeper, read the full article here.
If you’ve got an informed opinion on the impact of AI on society, consider writing a guest post for our community — just send your pitch to support@montrealethics.ai. You can pitch us an idea before you write, or a completed draft.
Events:
As a part of our public competence building efforts, we host events frequently spanning different subjects as it relates to building responsible AI systems, you can see a complete list here: https://montrealethics.ai/meetup
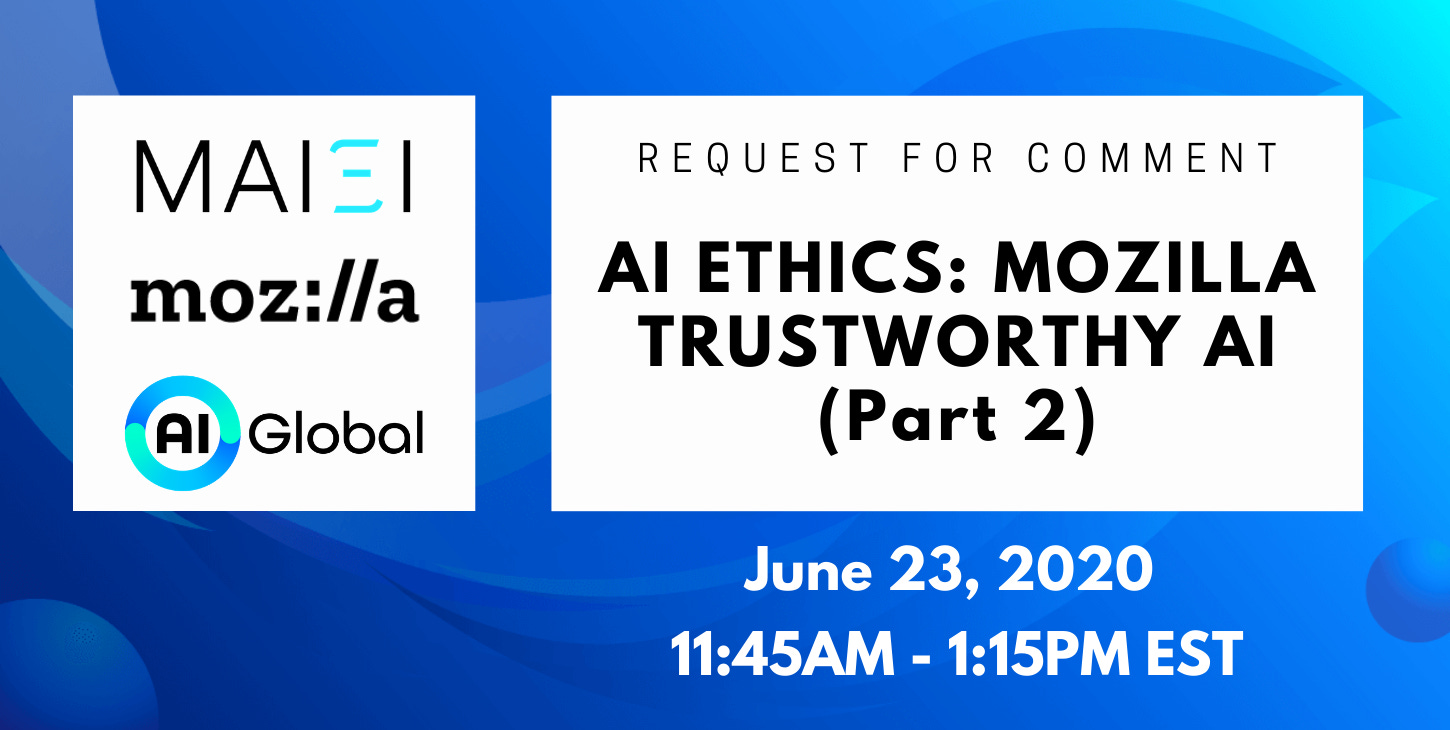
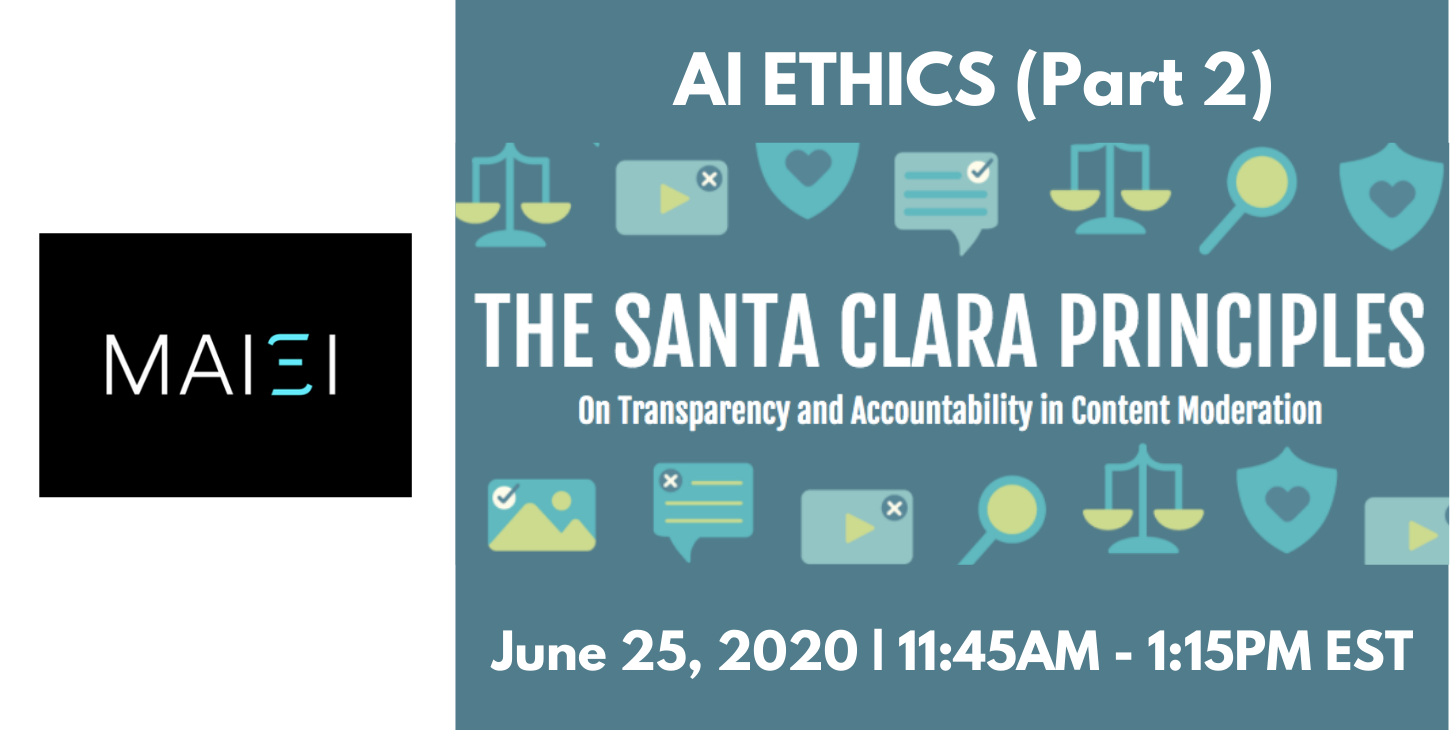
We’ve got 2 events lined up, one each week on the following topics, for events where we have a second edition, we’ll be utilizing insights from the first session to dive deeper, so we encourage you to participate in both (though you can just participate in either, we welcome fresh insights too!)
AI Ethics: Mozilla RFC for Trustworthy AI (Part 2)
June 23, 11:45 AM - 1:15 PM ET (Online)
AI Ethics: Santa Clara Principles for Content Moderation (Part 2)
June 25, 11:45 AM - 1:15 PM ET (Online)
You can find all the details on the event page, please make sure to register as we have limited spots (because of the online hosting solution).
From elsewhere on the web:
Things from our network and more that we found interesting and worth your time.
Researchers propose framework to measure AI’s social and environmental impact by Kyle Wiggers, VentureBeat
In a newly published paper on the preprint server Arxiv.org, researchers at the Montreal AI Ethics Institute, McGill University, Carnegie Mellon, and Microsoft propose a four-pillar framework called SECure designed to quantify the environmental and social impact of AI. Through techniques like compute-efficient machine learning, federated learning, and data sovereignty, the coauthors assert scientists and practitioners have the power to cut contributions to the carbon footprint while restoring trust in historically opaque systems.
Signing off for this week, we look forward to it again in a week! If you enjoyed this and know someone else that can benefit from this newsletter, please share it with them!
If you have feedback for this newsletter or think there is an interesting piece of research, development or event that we missed, please feel free to email us at support@montrealethics.ai
If someone has forwarded this to you and you like what you read, you can subscribe to receive this weekly newsletter by clicking below