
Discover more from The AI Ethics Brief
AI Ethics #15: Classical ethics, climate denial on Facebook, speech recognition bias, comparing human and machine perception, and more ...
Fairness in AI for people with disabilities, SOTA in countering information influence activities, accidentally triggering Amazon Echo, and other highlights from the world of AI Ethics!
Welcome to the fifteenth edition of our weekly newsletter that will help you navigate the fast changing world of AI Ethics! Every week we dive into research papers that caught our eye, sharing a summary of those with you and presenting our thoughts on how it links with other work in the research landscape. We also share brief thoughts on interesting articles and developments in the field. More about us on: https://montrealethics.ai/about/
If someone has forwarded this to you and you want to get one delivered to you every week, you can subscribe to receive this newsletter by clicking below:
MAIEI at ICML 2020:
ICML is one of the premier machine learning conferences in the world and MAIEI is happy to share that some of our research work has been selected for presentation at workshops there:
Our researchers Abhishek Gupta and Erick Galinkin will be presenting their work on Green Lighting ML: Confidentiality, Integrity, and Availability of Machine Learning Systems in Deployment at the ICML 2020 workshop on Deploying and Monitoring Machine Learning Systems.
Our researchers Abhishek Gupta and Camylle Lanteigne along with Sara Kingsley from Carnegie Mellon University will be presenting their work on SECure: A Social and Environmental Certificate for AI Systems at the ICML 2020 workshop on Deploying and Monitoring Machine Learning Systems.
State of AI Ethics June 2020 report:
We released our State of AI Ethics June 2020 report which captured the most impactful and meaningful research and development from across the world and compiled them into a single source. This is meant to serve as a quick reference and as a Marauder’s Map to help you navigate the field that is evolving and changing so rapidly. If you find it useful and know others who can benefit from a handy reference to help them navigate the changes in the field, please feel free to share this with them!
Summary of the content this week:
In research summaries this week, we dive into how classical ethics fits into AI, using chess as a model system to analyze how super human AI might align with human behaviour, a research roadmap for analyzing fairness concerns in AI systems as it relates to people with disabilities, looking at the state of the art in countering information influence activities, and bias in recruitment algorithms.
In article summaries this week, we take a look at how climate change related misinformation spreads as scientists debunking this face restrictions, how speech recognition technology is still laden with biases, research into accidental triggering of smart voice assistants that can compromise your privacy, how end-of-life care conversations can be nudged on doctors by AI, how power shifts occur because of AI, and comparing human and machine perception.
Our learning communities and the Co-Create program continue to receive an overwhelming response! Thank you everyone!
We operate on the open learning concept where we have a collaborative syllabus on each of the focus areas and meet every two weeks to learn from our peers. You can fill out this form to receive an invite!
Research:
Let's look at some highlights of research papers that caught our attention at MAIEI:
Classical Ethics in A/IS in Ethically Aligned Design: A Vision for Prioritizing Human Well-being with Autonomous and Intelligent Systems, First Edition.
The ethical implications of autonomous and intelligent systems (A/IS) are, by now, notably numerous and complex. This chapter of Ethically Aligned Design: A Vision for Prioritizing Human Well-being with Autonomous and Intelligent Systems by The IEEE Global Initiative on Ethics of Autonomous and Intelligent Systems simultaneously adds some definition to the issues surrounding the ethics of autonomous systems by providing clear analysis and recommendations. The topics of inquiry covered in the paper are wide, and each is given between two and four pages of background and subsequent recommendations. At the end of each section, readers will be happy to find a list of further readings if they wish to dive deeper into a specific topic.
To delve deeper, read our full summary here.
Aligning Super Human AI with Human Behavior: Chess as a Model System by Reid McIlroy-Young, Siddhartha Sen, Jon Kleinberg, and Ashton Anderson.
Artificial Intelligence (AI) is becoming smarter and smarter every day. In some cases, AI is achieving or surpassing superhuman performance. AI systems typically approach problems and decision making differently than the way people do (McIlroy-Young, Sen, Kleinberg, Anderson, 2020). The researchers in this study (McIlroy-Young, Sen, Kleinberg, Anderson, 2020) created a new model that explores human chess players’ behavior at a move-by-move level and the development of chess algorithms that match the human move-level behavior. In other words, the current systems for playing chess online is designed to play the game to win.
To delve deeper, read our full summary here.
Toward Fairness in AI for People with Disabilities: A Research Roadmap by Anhong Guo, Ece Kamar, Jennifer Wortman Vaughan, Hanna Wallach, Meredith Ringel Morris
In this position paper, the authors identify potential areas where Artificial Intelligence (AI) may impact people with disabilities (PWD). Although AI can be extremely beneficial to these populations (the paper provides several examples of such benefits), there is a risk of these systems not working properly for PWD or even discriminating against them. This paper is an effort towards identifying how inclusion issues for PWD may impact AI, which is only a part of the authors’ broader research agenda.
To delve deeper, read our full summary here.
From our learning communities:
Research that we covered in the learning communities at MAIEI this week, summarized for ease of reading:
Countering Information Influence Activities: The State of the Art by James Pamment, Howard Nothhaft, Henrik Agardh-Twetman, Alicia Fjällhed
In the national security context, information influence operations often take place alongside concerted military, diplomatic, and economic activities – they are part of a hybrid approach to warfare. The report frames the defense challenge in three parts, understanding the nature of the threat, learning to identify it it, and the development and application of countermeasures .There are three broad characteristics that can be used to define and operationalize the concept of information influence activities:
Legitimacy: They are illegitimate attempts to change opinions in democratic states
Intention: They are conducted to benefit foreign powers (state, non-state, or proxy)
Ambiguity: They are conducted in a gray zone of hybrid threat between peace and war
To delve deeper, read our full summary here.
Articles:
Let’s look at highlights of some recent articles that we found interesting at MAIEI:
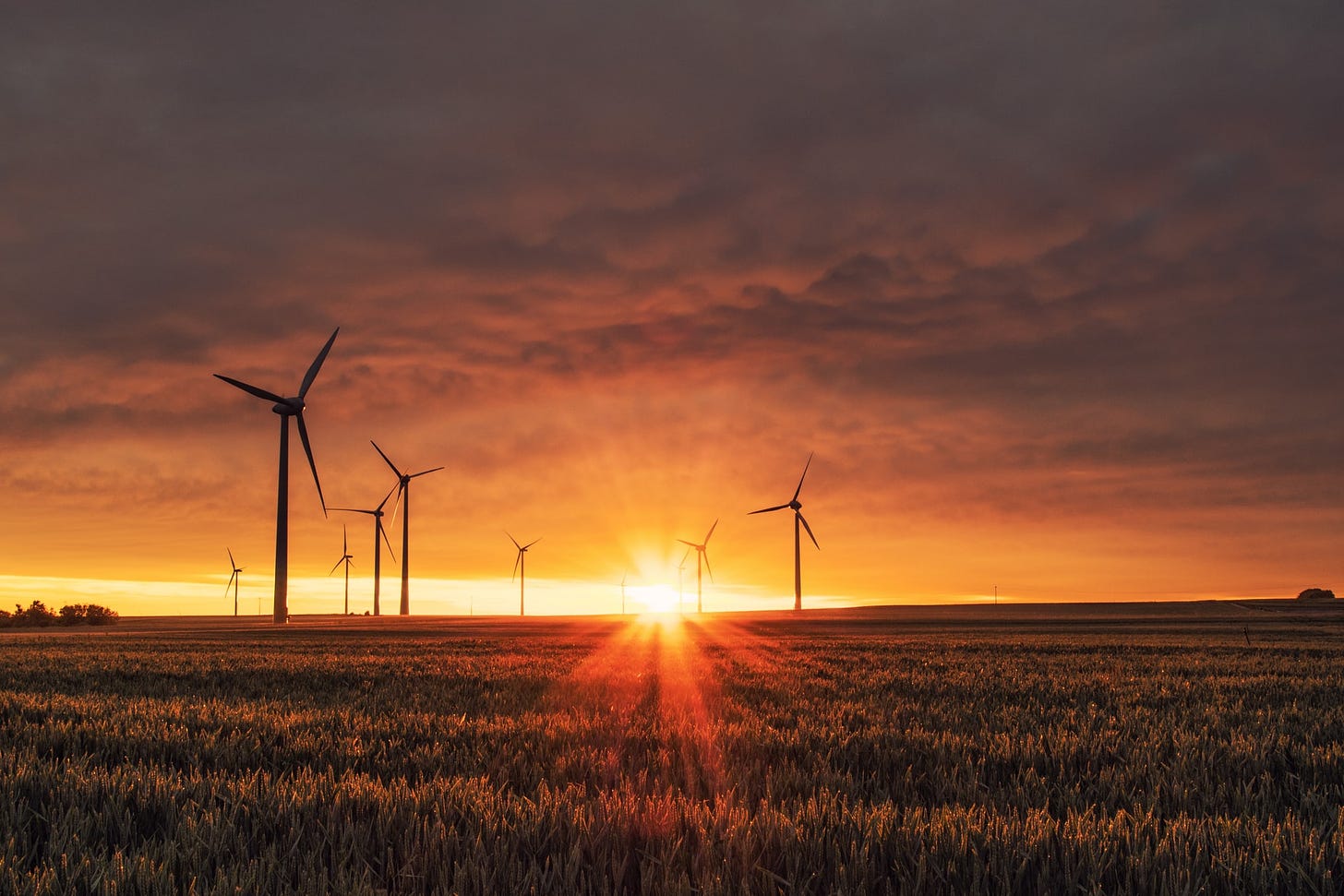
Photo by Karsten Würth on Unsplash
Climate Denial Spreads on Facebook as Scientists Face Restrictions (Scientific American)
In a time when the Earth has had a brief respite from carbon emissions due to a slowdown in human activity, leveraging disinformation tactics online to further polarize the climate change debate is particularly problematic. Facebook has weakened protections against the spread of climate change related disinformation. The fact-checking mechanisms that are put in place to prevent the abuse of the platform faced some serious threats in terms of what might be a bad precedent for the future of the platform when Facebook overruled the decision provided by an independent fact-checking body. Essentially, it seems that they are now allowing disinformation to spread if they are labeled as opinions which leads the content moderation space into a very slippery slope. To read more on potential fixes to the current content moderation practices, see this publication from the Montreal AI Ethics Institute in its response to the Santa Clara Principles.
A leading climate scientist’s posts were branded as political thus requiring her to provide more private information before she will be allowed to promote and publish posts that she creates which are educational posts debunking some of the climate change related disinformation on the platform. It places an undue burden on scientists who do this sort of work in public interest while organized groups that target the most susceptible populations on the platform with coordinated disinformation campaigns run rampant without any oversight.
In another case where the platform had reversed the label of “false” on a piece of content after it supposedly received pressure from a conservative that moved them to make the change in the label that was provided by a fact-checking organization in their verified network, observers are wondering if these are one-off incidents or this is a signal towards a broad policy change. In revising how Facebook governs content related to climate change under the “environmental politics” category, the platform is placing undue burdens on good samaritans on the platform while allowing for malicious actors to take advantage of the lax policies allowing disinformation to run unchecked harming millions.
Speech Recognition Tech Is Yet Another Example of Bias (Scientific American)
In numerous talks given by our founder Abhishek Gupta, he has pointed out how speech recognition technologies create nudges that are reshaping our conversation patterns such that we, as humans, have to adopt our speech patterns to meet the needs of the machines rather than have the machines adopt their structures so that they can accommodate human diversity. For those who use English as their second-language or don’t have a “mainstream” accent, chances of having high failure rates by speech recognition systems are pretty high. The study linked in the article points to how black people in the US face a disproportionate burden of such speech-related discrimination that imposes an unnecessary choice for them to either abandon their identity or abandon the use of those devices.
In human speech, we make accommodations for each other on a daily basis to be able to negotiate and navigate different accents and dialects. But, with machines there is no such negotiation and it is a very binary outcome that marginalizes those who don’t conform to what the machines have been trained on. In cases of people with speech disabilities, this is even more problematic since they might rely on such systems to go about their daily lives.
In addition, language is highly contextual and depending on the speaker and the context within which words and phrases are being used determine to a large degree the meaning and response which is something that is still not quite possible with current NLP systems. While adding in more diversity in training datasets is one option, a lot of it will also depend on more inclusive design practices. As a starting point, having more testing being done with those who are going to be the users of these systems will go a long way in making these systems better. Some researchers at the Montreal AI Ethics Institute have advocated for participatory design approaches as a way of making technological solutions more context- and culture-sensitive.
Researchers identify dozens of words that accidentally trigger Amazon Echo speakers (VentureBeat)
If privacy concerns were the only thing that you worried about when bringing home a smart voice assistant, think again! Our researchers have been advocating for thinking more critically about adversarial machine learning and machine learning security as important considerations in the design, development, and deployment of ML systems, something that has been covered quite frequently in our past newsletters. The research work covered in this article talks about LeakyPick, an approach that was used to check accidental triggering of smart voice assistants to start capturing audio snippets even when they weren’t invoked. This has severe privacy implications given that a lot of personal data can be captured inadvertently when the devices are turned on and they send over their information to the central servers for processing.
Using phonemes similar to the wakewords that are used to activate these devices, the researchers tested a wide range of devices and found that they were demonstrable and repeatable instances where the devices woke up and recorded conversations when they weren’t invoked using the actual wakeword. Another thing that was highlighted by the researchers was that even secondary checks on the end of the devices and servers let slip some of these accidental trigger words pointing to glaring problems in these devices as they are implemented at present. A step in the direction for ensuring security and privacy in this context is work being done at the Montreal AI Ethics Institute by Abhishek Gupta and Erick Galinkin that is slated to appear in the DMMLSys workshop at ICML this week.
Bringing the worst sort of dystopias alive from the series Black Mirror, medical institutions are beginning to use AI systems to nudge doctors to have end-of-life conversations with patients that the system deems are at risk of dying. Doctors are put in awkward positions bringing up the most intimate and sensitive thing up to a patient based on the recommendation of a machine.
In some cases, doctors agree with the recommendations coming from the system in terms of which patients they should broach these subjects, the article points to the case of one doctor who mentions that this system has helped to make her judgement sharper. Other doctors make sure to exclude mentioning that it was a machine that prompted them to have that conversation because it is inherently cold and often unexplainable in why it arrived at a certain decision which makes it even more challenging for patients to grasp why this is being discussed.
A particular challenge arises when doctors disagree with the recommendation from the system. In this case, even though the doctor does have the final say, they are weighed down by the consideration of whether or not they are making the right decision in not bringing up these advanced care options with the patient in case they are wrong and the system is indeed right. Another problem to be highlighted is a potential over-reliance on the system for making these decisions and reducing the autonomy that doctors would have, related to the token human problem.
The designers of the systems have taken into consideration many different design choices, especially around the number of notifications and alerts to provide the doctors to avoid “notification fatigue”. Another design consideration is to explicitly not include the probability rating with the patient list, given the understanding that humans are terrible at discerning differences between percentage figures unless they are on the extremes or dead-center. The labeling around the recommendations coming from the system are also framed as those requiring “palliative care” rather than talking about “will die” which can subtly create different expectations. One of the benefits of a system like this, as documented in an associated study, is that it has helped to boost the number of conversations around this subject that are being had with the patients which are essential and are sometimes ignored due to competing priorities and time burdens on the doctors.
Don’t ask if artificial intelligence is good or fair, ask how it shifts power (Nature)
A very timely article that sheds light on the power dynamics that exist in the field of AI and how they help to reinforce the status quo in society at large rendering harm on those who are already marginalized. Instead of empowering data subjects to have greater control over how these systems make decisions about them, the systems abstract away that power into even more concentrated interests. The author rightly points out some of the concerns around the definitions of “fair”, “transparent” and calls attention to the potential problem of “ethics-washing” that might be causing more pollution in the ecosystem in terms of legitimate vs. superficial initiatives that can help to address some of the problems with AI systems today.
Concluding the article by mentioning the following, we believe that this is increasingly important for everyone working on technology solutions that integrate and interact with people in a societal context to take into consideration: “When the field of AI believes it is neutral, it both fails to notice biased data and builds systems that sanctify the status quo and advance the interests of the powerful. What is needed is a field that exposes and critiques systems that concentrate power, while co-creating new systems with impacted communities: AI by and for the people.”
Challenges of Comparing Human and Machine Perception (The Gradient)
As we have covered in past newsletters, there are a lot of problems in how reporting is done around progress made in the field of ML. The authors of this research highlight some common pitfalls to help create more realistic expectations by identifying some of the challenges that arise when we try to compare machine perception performance against that of humans.
The first pitfall that they focus on is how humans are too quick to conclude that machines have learned human-like concepts. As an experimental setup, borrowing from the Gestalt theory of contour closeness, they design an experiment to analyze if indeed visual processing is done similar to how humans perceive visuals. What they find is that the machine models actually pick up cues in the data in a very different way compared to how humans process images and one of the measures to counter against coming to conclusions that humans and machine analogue systems works in the same way is to thoroughly examine the model, the decision-making process, and the dataset to arrive at conclusions that are rooted in empirical evidence rather than unnecessarily anthropomorphizing these systems.
The second pitfall that they identify is that it is hard to draw conclusions about the generalizing that the model can do beyond the tested architectures and training procedures. Analyzing same-different and spatial analysis tasks, they show that there is a problem in making unfair comparisons between human and machine perception because the baselines might be very different. They find that the low data regime isn’t all that meaningful in making inferences about what the system performance is going to be like in the wild. The learning speed also greatly depends on the starting conditions of the system. What they mean by that is that there is a lot of context and life-long learning that is done by humans that gives them their perceptual ability and doing a baseline comparison with machines in that setting becomes really hard unless an analogous starting condition can be created for the machine as well.
Building on this, the final pitfall that the researchers explore is finding strong parallels in the experimental setups to compare humans and machines. When doing comparisons on drops in performance on recognizability when images are progressively cropped, aligning the testing conditions so that they are tailored to humans and to machines actually leads to conclusions that are quite different from existing studies.
Keeping these ideas in mind would lend a higher degree of realism to the expectations that we have from the systems and more pragmatically evaluate the capability of these systems.
From the archives:
Here’s an article from our blogs that we think is worth another look:
Our Staff Researcher, Tania De Gasperis completed her Master’s Research Project on Futures of Responsible and Inclusive AI that talks about how might we foster an inclusive, responsible & foresight-informed AI governance approach?
This paper seeks to investigate how we might foster an inclusive, foresight-informed responsible AI governance framework. The paper discusses the gaps and opportunities in current AI initiatives across various stakeholders and acknowledges the importance of anticipation and agility. This paper also posits that it is important for legal, policy, industry and academia to understand the specificities of each other’s domains better to build an inclusive governance framework.
To delve deeper, read the full article here.
Guest contributions:
Why was your job application rejected: Bias in Recruitment Algorithms? (Part 1)
This guest post was contributed by Merve Hickok (SHRM-SCP), founder of AIethicist.org. It is part 1 of a 2-part series on bias in recruitment algorithms.
Humans are biased. The algorithms they develop and the data they use can be too, but what does that mean to you as a job applicant coming out of school or looking to move up to the next step in career ladder or considering a change in roles or industry?
In this two-part article, we walk through each stage of recruitment (targeting, sourcing/matching, screening, assessment and social background checks) and explore how some of the AI-powered commercial software used in each stage can lead to unfair and biased decisions.
To delve deeper, read the full article here.
If you’ve got an informed opinion on the impact of AI on society, consider writing a guest post for our community — just send your pitch to support@montrealethics.ai. You can pitch us an idea before you write, or a completed draft.
Events:
As a part of our public competence building efforts, we host events frequently spanning different subjects as it relates to building responsible AI systems, you can see a complete list here: https://montrealethics.ai/meetup
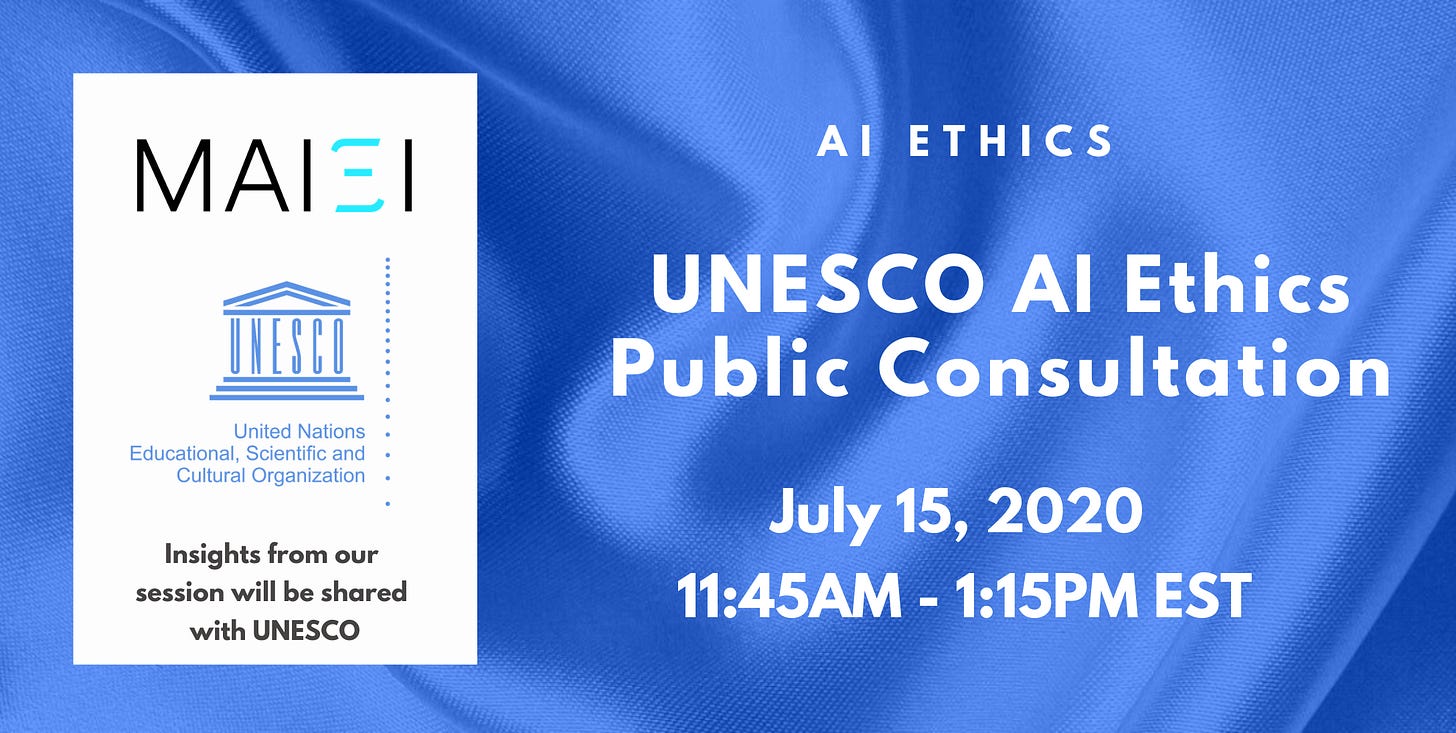
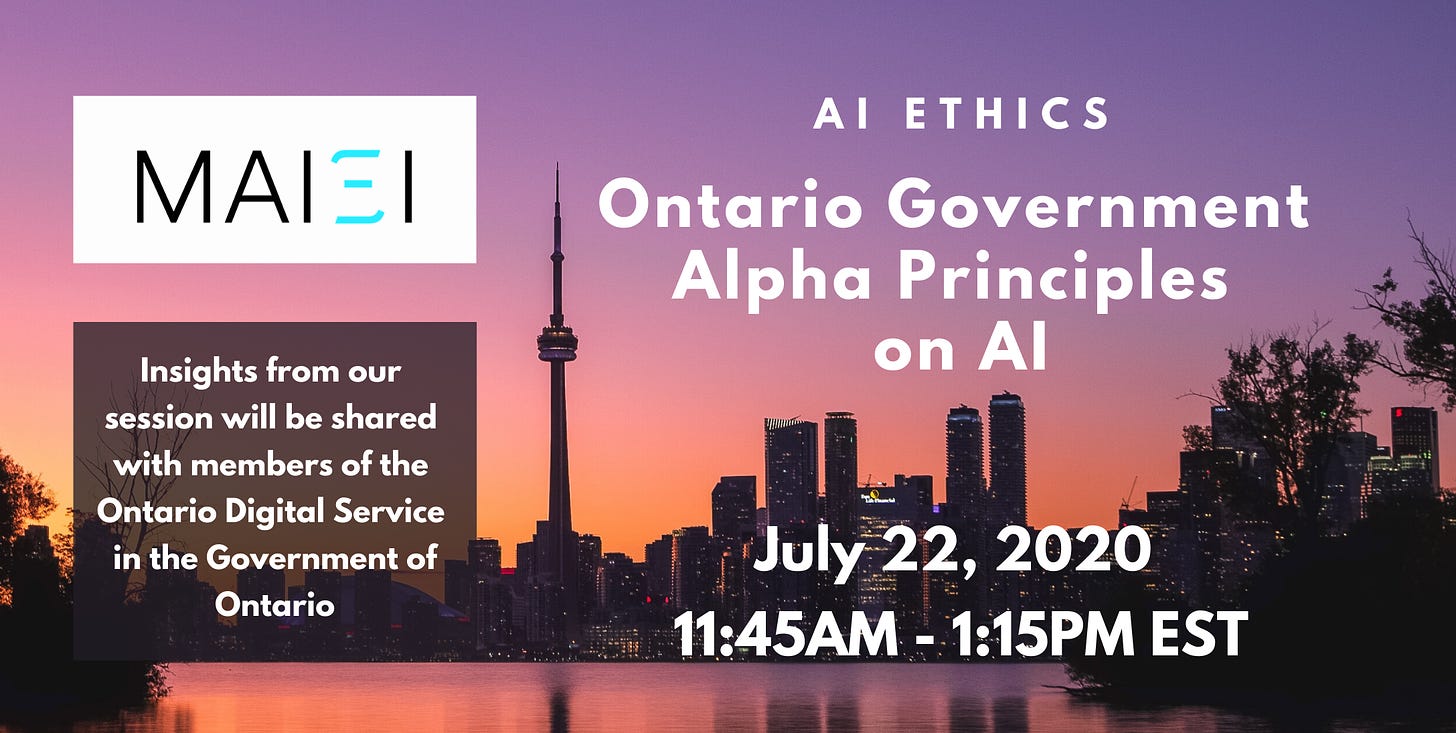
AI Ethics: UNESCO AI Ethics Public Consultation
July 15, 11:45 AM - 1:15 PM ET (Online)
AI Ethics: Ontario Government Alpha Principles on AI
July 22, 11:45 AM - 1:15 PM ET (Online)
You can find all the details on the event page, please make sure to register as we have limited spots (because of the online hosting solution).
From elsewhere on the web:
Things from our network and more that we found interesting and worth your time.
SECure: A Social and Environmental Certificate for AI Systems was featured in the video series by Alex Castrounis on YouTube explaining in easy to digest language and terms the SECure framework and what it entails for building more eco-socially responsible AI systems.
Montreal AI Ethics Institute Hosts a TechAIDE Café Session: On July 7th, the Montreal AI Ethics Institute had the privilege of hosting a TechAIDE Café session. The aim of the café is to raise funds for Centraide Montréal, a philanthropic organization that raises money to help fight poverty, homelessness, and social exclusion. Funds are raised when participants pledge, either on Twitter or by email to Centraide, that they will “buy a coffee,” and the size of the coffee bought is proportional to the amount donated. Centraide then reaches out to the individuals who made the pledge and invites them to donate the amount they pledged.
Signing off for this week, we look forward to it again in a week! If you enjoyed this and know someone else that can benefit from this newsletter, please share it with them!
If you have feedback for this newsletter or think there is an interesting piece of research, development or event that we missed, please feel free to email us at support@montrealethics.ai