
Discover more from The AI Ethics Brief
AI Ethics #19: Children's perspective on creepy technologies, collecting sociocultural data in ML, mass incarceration and the future of AI, and more ...
Merging public data and racial equity implications, HITL systems, data supply chains, differential privacy primer, Baby's First 100 MLSec Words, and more from the world of AI Ethics!
Welcome to the nineteenth edition of our weekly newsletter that will help you navigate the fast changing world of AI Ethics! Every week we dive into research papers that caught our eye, sharing a summary of those with you and presenting our thoughts on how it links with other work in the research landscape. We also share brief thoughts on interesting articles and developments in the field. More about us on: https://montrealethics.ai/about/
If someone has forwarded this to you and you want to get one delivered to you every week, you can subscribe to receive this newsletter by clicking below:
Summary of the content this week:
In research summaries this week, we look at how children perceive creepy technologies, lessons from archives and strategies for collecting sociocultural data in machine learning, mass incarceration and the future of AI, a case for human-in-the-loop and making decisions in the presence of erroneous algorithmic scores, and a framework for security and privacy in machine learning.
In article summaries this week, we look at how hackers broke into real news sites to plant fake stories, the consequences of merging public data on racial equity, an introduction to differential privacy as a privacy-preserving mechanism in machine learning, how not to know ourselves, five reasons why autonomous vehicles are still not on our roads, and how we can protect our essential workers in the data supply chain.
MAIEI Community Initiatives:
Our learning communities and the Co-Create program continue to receive an overwhelming response! Thank you everyone!
We operate on the open learning concept where we have a collaborative syllabus on each of the focus areas and meet every two weeks to learn from our peers. You can fill out this form to receive an invite!
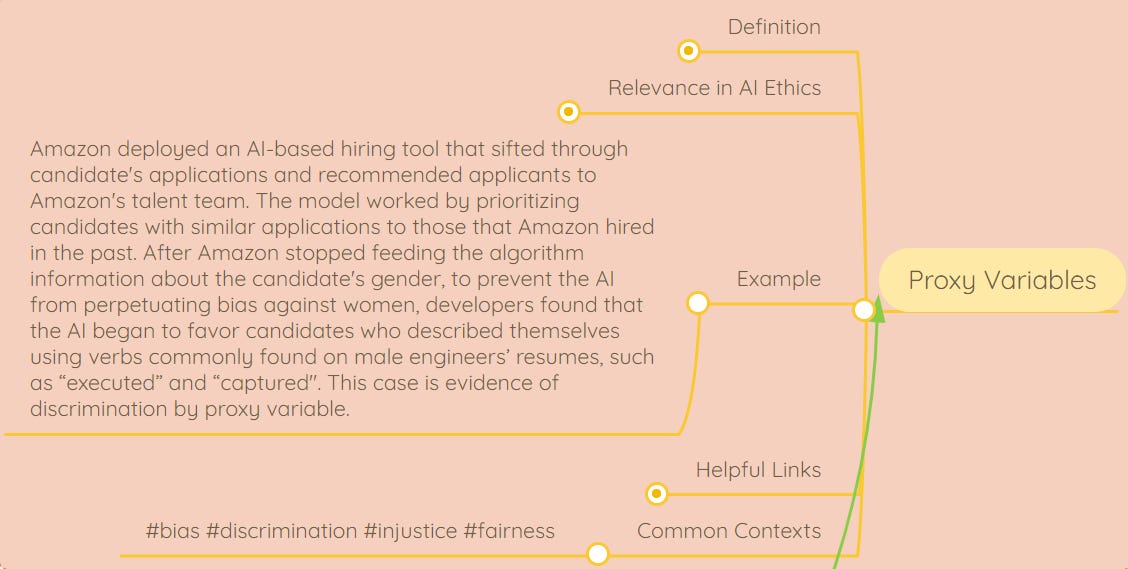
AI Ethics Concept of the week: ‘Proxy Variables’
Proxy variables are ostensibly neutral data points that provide sensitive information by serving as a stand-in for other variables, often perpetuating inequities. See Amazon-related example in the screenshot above, pulled from our AI Ethics Living Dictionary, a resource we’ve created to make AI ethics knowledge more accessible.
Learn about the relevance of proxy variables to AI ethics, helpful related links, and more in the full dictionary. 👇
Explore the AI Ethics Living Dictionary!
MAIEI Serendipity Space:
The first session was a great success and we encourage you to sign up for the next one!
This will be a 30 minutes session from 12:15 pm ET to 12:45 pm ET so bring your lunch (or tea/coffee)! Register here to get started!
Research:
Let's look at some highlights of research papers that caught our attention at MAIEI:
Laughing is Scary, but Farting is Cute: A Conceptual Model of Children’s Perspectives of Creepy Technologies by Jason C. Yip, Kiley Sobel, Xin Gao, Allison Marie Hishikawa, Alexis Lim, Laura Meng, Romaine Flor Ofiana, Justin Park, Alexis Hiniker
Designing AI technologies for children often focuses on adult concerns for children rather than examining the problem from a child’s perspective. In this recent paper from researchers at the University of Washington, in-depth user research led to the development of a conceptual model that provides important considerations and insights for parents and designers. By focusing on solely how children view technology as creepy or trustworthy, the research team pointed out problematic designs in apps or toys designed for children.. By using dark patterns, designers create technologies that children will trust when they shouldn’t and create applications that simply frighten children in ways that adults do not consider. Using this model as a basis, designers, parents, and advocacy groups can more formally define ethical considerations in technological designs for children.
To delve deeper, read our full summary here.
Lessons from Archives: Strategies for Collecting Sociocultural Data in Machine Learning by Eun Seo Jo (Stanford University) and Timnit Gebru (Google)
It’s no secret that there are significant issues related to the collection and annotation of data in machine learning (ML). Many of the ethical issues that are discussed today in ML systems result from the lack of best practices and guidelines for the collection and use of data to train these systems. For example, Professor Eun Seo Jo (Stanford University) and Timnit Gebru (Google) write, “Haphazardly categorizing people in the data used to train ML models can harm vulnerable groups and propagate societal biases.”
In this article, Seo Jo and Gebru set out to examine how ML can apply the methodologies for data collection and annotation utilized for decades by archives: the “oldest human attempt to gather sociocultural data.” They argue that ML should create an “interdisciplinary subfield” focused on “data gathering, sharing, annotation, ethics monitoring, and record-keeping processes.” In particular, they explore how archives have worked to resolve issues in data collection related to consent, power, inclusivity, transparency, and ethics & privacy—and how these lessons can be applied to ML, specifically to subfields that use large, unstructured datasets (e.g. Natural Language Processing and Computer Vision).
The authors argue that ML should adapt what archives have implemented in their data collection work, including an institutional mission statement, full-time curators, codes of conduct/ethics, standardized forms of documentation, community-based activism, and data consortia for sharing data.
To delve deeper, read our full summary here.
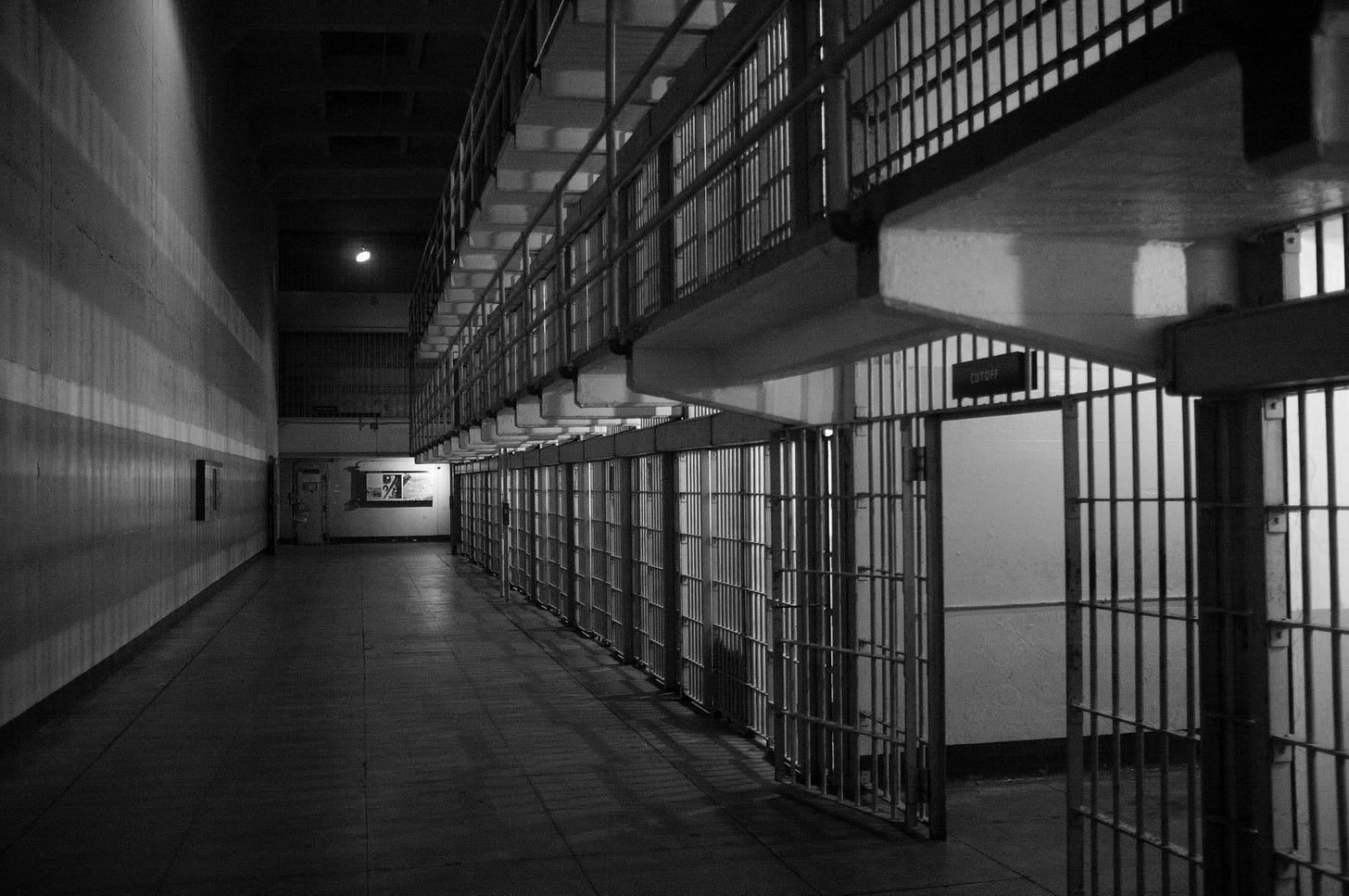
Photo by Emiliano Bar on Unsplash
Mass Incarceration and the Future of AI by Teresa Y. Hodge and Laurin Leonard
The US, with a staggering 25% of the world’s prison population, has been called the incarceration nation. For millions of Americans, background checks obstruct their social mobility and perpetuate the stigma around criminal records. The digitization of individual records and the growing use of online background checks will lead to more automated barriers and biases that can prevent equal access to employment, healthcare, education and housing. The easy access and distribution of an individual’s sensitive data included in their criminal record elicits a debate on whether public safety overrides principles of privacy and human dignity. The authors, in this preliminary discussion paper, address the urgency of regulating background screening and invite further research on questions of data access, individual rights and standards for data integrity.
To delve deeper, read our full summary here.
A Case for Humans-in-the-Loop: Decisions in the Presence of Erroneous Algorithmic Scores by Maria De-Arteaga, Riccardo Fogliato, and Alexandra Chouldechova
The paper highlights important considerations in the design of automated systems when they are used in “mission-critical” contexts, for example, in places where such systems are making decisions that will have significant impacts on human lives. The authors use the case study of a risk-assessment score system that helps to streamline the screening process for child welfare services cases. It considers the phenomena of algorithmic aversion and automation bias keeping in mind omission and commission errors and the ability of humans to acknowledge such errors and act accordingly. It goes into detail on how designing the systems where humans are empowered with the autonomy to consider additional information and override the recommendations made by the system lead to demonstrably better results. It also points out how this is more feasible in cases where humans have training and experience in making decisions without the use of an automated system.
To delve deeper, read our full summary here.
From our learning communities:
Research that we covered in the learning communities at MAIEI this week, summarized for ease of reading:
Check back here next week!
Articles:
Let’s look at highlights of some recent articles that we found interesting at MAIEI:
Hackers Broke Into Real News Sites to Plant Fake Stories (Wired)
The success of disinformation lies in its ability to deceive the intended audiences into believing the content is trustworthy and truthful. While it might be hard to generate content and have it spread via social media to gain enough legitimacy to move the needle, there is tremendous potential for harm if such content gets wrapped in the veneer of respected news outlets. In particular, information operatives have leveraged the technique of hacking into content management systems (CMS) of media houses to plant fake news stories.
Researchers identified the use of this technique leading to divisiveness in eastern Europe, targeting NATO related news items along with relations of those countries with the US. While there are reporting mechanisms, if the content stays up long enough, it has the potential to be copied and impact people nonetheless. Emphasis on cybersecurity becomes critical in combating such attacks. In addition to fighting disinformation along the lines of content, provenance, and other dimensions, all of these efforts get subverted if appropriate guards are not in place.
Merging Public Data Sets Has Implications for Racial Equity (Built In)
The article begins by pointing out an example where Detroit PD pushed to separate the racial impacts of facial recognition technology (FRT) from the city's video surveillance program. The thrust of their argument is that FRT is one among many tools used by detectives, and hence it doesn't have a huge impact. But, on every step in the data lifecycle, there are pitfalls in terms of exacerbating bias, especially when data gets integrated across multiple agencies and creates richer profiles.
The racial equity toolkit created by the researchers in the article advocates for the interrogation of these issues in the planning, data collection, data access, algorithms and statistical tools selection, data analysis, and reporting phases of the life cycle.
Data integration is a turbocharged version of data sharing whereby more invasive analysis can be carried out on the resulting, merged datasets. The researchers point to a case in North Carolina whereupon combining data from multiple agencies, citizens who utilized several government aid programs in parallel were targeted and subsequently lost access as they were seen to be high-risk individuals. Involving a data custodian — someone who owes a responsibility to the data subjects, is a great way to ensure that the rights of citizens are respected. Even at the conception stage, there needs to be a reflection on whether the project is the best way to achieve a social outcome or if it is just being carried out because there are grant dollars available. Augmenting existing data with qualitative analysis and surveys can unearth potential problems with the quantitative data on hand.
Disaggregating data presents a promising approach, yet it shouldn't be relied upon solely as the means to address problems with racial equity. Finally, the researchers say that ignorance is no longer a defensible position, the use of a toolkit like this forces difficult conversations that will guide people in building inclusive AI systems.
Differential Privacy for Privacy-Preserving Data Analysis: An Introduction to our Blog Series (National Institute of Standards and Technology)
Doing data analysis on personally identifiable information (PII) is rife with privacy challenges. One promising technique that seeks to overcome many of the shortcomings of other popular methods is called differential privacy. In particular, we would like to do our data analysis such that we can unearth trends without learning anything new about specific individuals. De-identification methods are vulnerable to database linkage attacks. Restriction to aggregated queries is also only feasible when the groups are large enough, and even then, they might be subject to privacy-compromising attacks.
Differential privacy is the mathematical formulation of what it means to have privacy. It is a property of a process rather than a method itself. It guarantees that the output of a differentially private analysis will be roughly the same whether or not your data gets included in the dataset. The strength of privacy gets controlled by a parameter called epsilon that is the privacy loss or the privacy budget. The lower the value of epsilon, the higher the degree of protection of the individual's data. But, queries with higher sensitivity require the addition of more noise, thus potentially diminishing the quality of the results.
The advantages of differentially private methods are as follows: the assumption that all information is private, resistance to privacy attacks based on auxiliary information, and compositionality of different differentially private methods. A minor drawback at the moment is the lack of many well-tested frameworks for implementing differentially private methods in practice but that is changing rapidly.
Autonomous cars: five reasons they still aren’t on our roads (The Conversation)
The pandemic has certainly thrown a wrench in terms of testing of autonomous vehicles (AV) that, at present, require the presence of human overseers. This article highlights some of the main challenges that the industry will have to overcome in making the presence of AVs in everyday settings a reality.
The current crop of sensors used on AVs is susceptible to failure in bad weather, heavy traffic, unexpected situations, graffiti on traffic signs, and other naturally occurring adversarial items. These sensors will need to be universal in their performance so that systems trained in one place might be amenable to deployment in another with different circumstances. The underlying machine learning systems that do object detection, path planning, and other operations don't yet have standardized approaches for training, validation, and testing, which is essential for benchmarking and safety. In an online learning context where these systems will learn on the open road, we need mechanisms that we can certify in terms of their safety even after updates to them through the learning that they have post-deployment.
Recognized regulations and standards across the industry that square with existing vehicle safety checks need implementation before there is widespread deployment. Finally, people need convincing from a social acceptance standpoint that the systems are safe for use. Without that, we risk abandonment and distrust that will kill the industry.
How Not to Know Ourselves (Data & Society)
Data from large, online platforms provide convenient data fodder for algorithmic analysis, especially given the penchant for deep learning to lean on big data for high predictive performance. Yet, this is plagued by what researchers in this article called "measurement bias." It refers to the collection of metrics that are fueled by the platforms' desire to run behavioral experiments in a bid to improve stickiness in user retention. Non-public actors increasingly control a large portion of this data with the sole intention of making profits and building their image.
The ascendance of platforms' power has left researchers asking critical questions on the representativeness of the data and privacy concerns. The captured data is "administrative data"; helping platforms meet goals of harvesting intermediation fees, advertiser revenue, and venture capital funding. The social science research implications are incidental to this process.
The subsequent data generation through user interactions is thus highly biased: it gets shaped by iterative experimentation carried out by the platforms to meet the goals stated above. Whether this data is a representation of user behavior or a result of the successful manipulation of users' behavior by the platform is hard to tell. Treating it as a representation of human society is reductionist. It risks putting platforms in a place where they are the entirety of the world, handing over even more power to these large, opaque organizations.
Protecting the Essential Workers of the Data Supply Chain (IDinsight)
In-person data collection is an essential tool for understanding our world. With 2020 being the year for the census in the US, this article makes concrete arguments in asking the WHO to come forth with guidelines on safe in-person survey methodologies. Not only is this data critical for administrative purposes, but the SDGs from the UN are also highly dependent on household survey information.
Some might argue for the use of phones and other technologies to capture equivalent data. But this is fraught with problems since technology penetration is uneven and tends to exclude the already marginalized from adequate representation. For example, studies have demonstrated that women get systematically less representation when relying solely on data collected via phone surveys. Such second-best alternatives are very risky when making consequential decisions.
Evidence-based guidelines from the WHO can help bridge this gap allowing surveyors to return to in-person data collection, protecting their health and of the interviewees. Some suggestions made in the article include shortening the surveys to focus on questions that require in-person conversations, providing workers with private transport, regular testing, carrying additional supplies of face masks and sanitizers that could be handed out as tokens of appreciation, and more. Bolstering these recommendations with the advice from medical experts will help us get back to high-quality data collection that is critical to the proper functioning of our society.
From elsewhere on the web:
Things from our network and more that we found interesting and worth your time.
The team from the Montreal AI Ethics Institute including Abhishek Gupta, Camylle Lanteigne, Muriam Fancy, and Ryan Khurana were featured in this podcast put together by Andrey Kurenkov from Stanford University where they talked about the latest State of AI Ethics Report June 2020.
Staff Researcher Erick Galinkin spoke at DEFCON 2020 on Baby’s First 100 MLSec Words with the following abstract:
Adversarial example, Deepfake, model inversion, model theft, data poisoning - these are just some of the terms that are thrown around casually in machine learning security but often do not register for security practitioners. As models get deployed more broadly across industry, it is incumbent upon security professionals on both red and blue teams, as well as artificial intelligence researchers who want to protect their systems, to be familiar with these terms and techniques. In this talk, we survey the landscape of threats, reference proofs of concept, discuss possible mitigation strategies, and call for more interdisciplinary research between machine learning and security
Staff Researchers Ryan Khurana, Alexandrine Royer, Muriam Fancy, and Abhishek Gupta spoke at this workshop hosted by AI4People on the areas of Labor Market Impacts of AI, Ethnography in Ethical AI, Governance approaches for AI, and Machine Learning Security.
Our team will be hosting a workshop with the CMU AI Audit Lab on responsible AI - this is a follow up to the workshop that the team had hosted for the CIFAR and OSMO AI4Good Lab. If you’d like for us to host a workshop for your organization, please don’t hesitate in reaching out to us.
Staff Researcher Connor Wright will be speaking on Mitigating bias in Facial Recognition Systems at this session being hosted by IndiaAI (a MEITY, NEGD, and NASSCOM India initiative).
From the archives:
Here’s an article from our blogs that we think is worth another look:
SoK: Security and Privacy in Machine Learning by Nicolas Papernot, Patrick McDaniel, Arunesh Sinha, and Michael P. Wellman
Despite the growing deployment of machine learning (ML) systems, there is a profound lack of understanding regarding their inherent vulnerabilities and how to defend against attacks. In particular, there needs to be more research done on the “sensitivity” of ML algorithms to their input data. In this paper, Papernot et. al. “systematize findings on ML security and privacy,” “articulate a comprehensive threat model” and “categorize attacks and defenses within an adversarial framework.”
To delve deeper, read the full article here.
Guest contributions:
If you’ve got an informed opinion on the impact of AI on society, consider writing a guest post for our community — just send your pitch to support@montrealethics.ai. You can pitch us an idea before you write, or a completed draft.
Events:
As a part of our public competence building efforts, we host events frequently spanning different subjects as it relates to building responsible AI systems, you can see a complete list here: https://montrealethics.ai/meetup
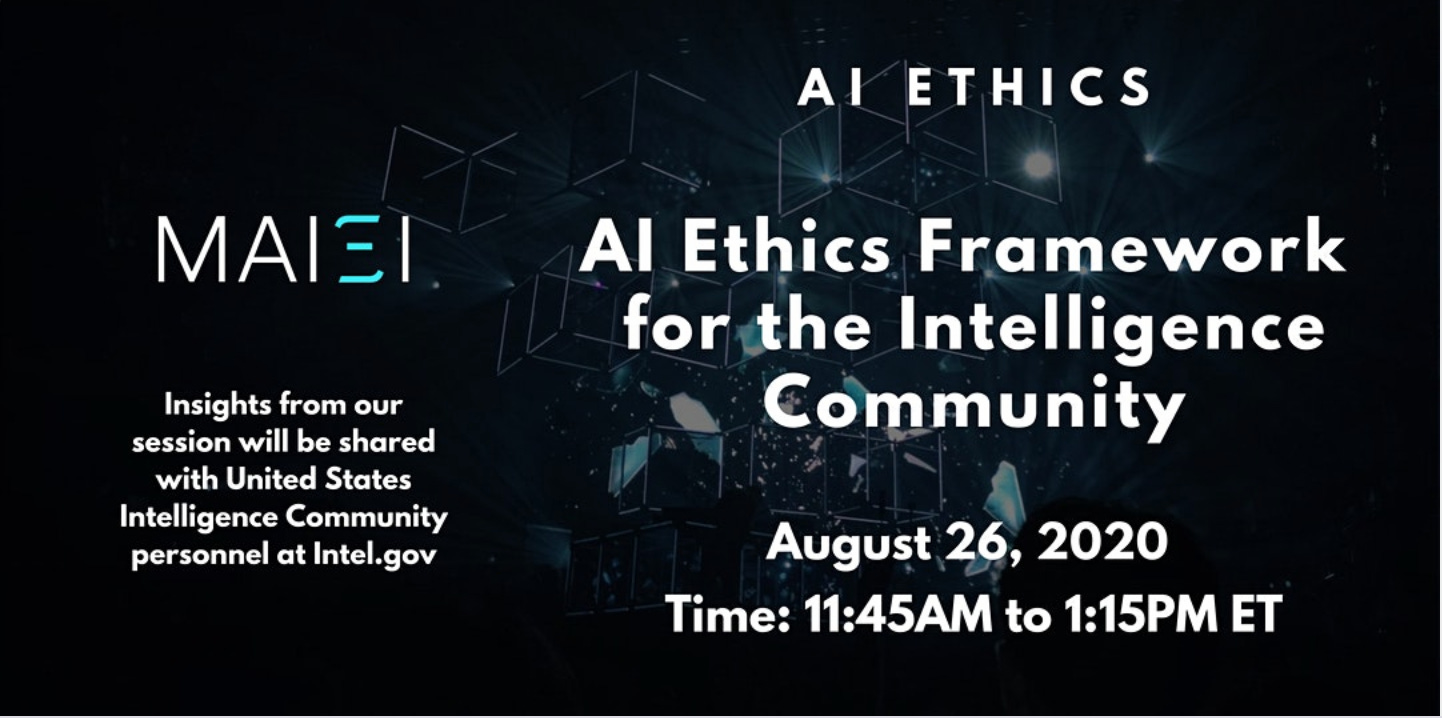
AI Ethics Framework for the US Intelligence Community
August 26, 11:45 AM - 1:15 PM ET (Online)
You can find all the details on the event page, please make sure to register as we have limited spots (because of the online hosting solution).
Signing off for this week, we look forward to it again in a week! If you enjoyed this and know someone else that can benefit from this newsletter, please share it with them!
If you have feedback for this newsletter or think there is an interesting piece of research, development or event that we missed, please feel free to email us at support@montrealethics.ai