AI Ethics #22: Anthropological guide to AI Ethics, algorithmic colonization, digital abundance and scarce genius, safety-critical AI systems, and more ...
National data service, comprehensiveness of archives, AI and IP, fighting back against automated fascism, robots are not eating our jobs, and more from the world of AI Ethics!
Welcome to the twenty-second edition of our weekly newsletter that will help you navigate the fast changing world of AI Ethics! Every week we dive into research papers that caught our eye, sharing a summary of those with you and presenting our thoughts on how it links with other work in the research landscape. We also share brief thoughts on interesting articles and developments in the field. More about us on: https://montrealethics.ai/about/
If someone has forwarded this to you and you want to get one delivered to you every week, you can subscribe to receive this newsletter by clicking below:
Summary of the content this week:
In research summaries this week, we cover the algorithmic colonization of Africa, digital abundance and the scarce genius: its implications on wages, interest rates, and growth, and a look at safety-critical AI systems from the perspective of the aviation industry.
In article summaries this week, we cover how the US and other countries could benefit from a national data service, how councils are scrapping the use of automated systems in making benefits and welfare decisions. how we can fight back against algorithms that are automating fascism, lessons learned from how big oil faded and what those mean for the world of big tech, examining Google’s offer to help other organizations to address AI ethics concerns, and why robots might not be eating our jobs.
In featured work from our staff this week, we have a short guide taking an anthropological perspective on AI ethics, advocacy for an AI-enabled approach to build more comprehensive archives (which was featured by VentureBeat!), MAIEI’s submission to WIPO on the impacts of AI on IP, and MAIEI’s examination of the COVI application that was developed in Canada to provide a contact-tracing solution.
In upcoming events, we have a session being hosted to discuss Facial Recognition Technologies. Scroll to the bottom of the email for more information.
MAIEI Learning Community:
Interested in working together with thinkers from across the world to develop interdisciplinary solutions in addressing some of the biggest ethical challenges of AI? Join our learning community; it’s a modular combination of reading groups + collaborating on papers. Fill out this form to receive an invite!
AI Ethics Concept of the week: ‘Supervised Learning’
This week's Living Dictionary definition is 'Supervised Learning': a technique used to teach AI how to make future predictions using labeled training data.
Learn about the relevance of supervised learning to AI ethics and more in our AI Ethics Living dictionary. 👇
Explore the Living Dictionary!
MAIEI Serendipity Space:
A digital space for unstructured, free-wheeling conversations with the MAIEI Staff and AI Ethics community at large to talk about whatever is on your mind in terms of building responsible AI systems
Next one is on September 3rd from 12:15 pm ET to 12:45 pm ET
Register via Zoom here to get started!
Research:
Let's look at some highlights of research papers that caught our attention at MAIEI:
Algorithmic Colonization of Africa by Abeba Birhane
In this seminal paper, Birhane argues that the current push to digitize Africa is no different from the historic colonization of the continent, now clothed in the modern garb of data-driven solutions and algorithmic decision making. Traditionally, colonizers were political entities but today we see Tech Monopolies wielding the same kind of influence and demonstrating the same appetite for wealth accumulation.
To delve deeper, read our full summary here.
Digital Abundance and Scarce Genius: Implications for Wages, Interest Rates, and Growth by Seth Benzell and Erik Brynjolfsson
A paradox that has been confounding economists in recent years is that both labor and capital are experiencing declines in their share of total income. Traditionally these two factors should account for nearly all of the income produced in an economy, with only a small share being taken by profits. The profit share of income has certainly been increasing, but the reasons for this trend and the differences in profits between different industries has been a contentious topic of debate.
Economists Seth Benzell and Erik Brynjolfsson propose that there is a missing component in these discussions, which they argue has been amassing a growing share of income that would have traditionally gone to labor or capital. They call this the “Genius” share of income, the amount of income that goes to superstar individuals or intangibles, such as a firm’s management practices and ability to leverage new technologies. The scarcity of “Genius” has allowed some firms to skyrocket ahead of their competition with the income distribution of their top employees far outpacing those in laggard industries.
The authors argue that increasing the supply of “Genius” through greater access to elite universities and more high-skilled immigration would not only kick start productivity in the wider economy but increase wages for all. The moral implications of this work are quite severe and the model raises lots of critical questions for researchers grappling with the social impacts of AI.
To delve deeper, read our full summary here.
The Flight to Safety-Critical AI by Will Hunt
The paper looks at the aviation industry as a case study for the adoption of AI safety standards and how a measured approach to first applying them in non-critical contexts like predictive maintenance, route planning, and decision support is a better approach to develop standards and practices that can then be applied to more safety-critical scenarios like flight control. It also stresses the importance of cross-jurisdictional coherence in this domain since flight safety acceptance is a cornerstone for trade and movement of capital and labor across borders.
The paper also applies the paradigmatic framing of different kinds of races and mentions that based on preliminary findings, it is clear that there is a gentle race to the top in terms of safety standards given this domain’s strong emphasis on operational safety over everything else. Finally, making several recommendations along the lines of the roles that regulators can play, how the industry players might interact with each other, and the incentives for the research segment to contribute to this are made that provide a roadmap to potentially apply some of the learnings from this to other domains where safety is of the essence.
To delve deeper, read our full summary here.
What we are thinking:
Op-eds and other work from our research staff that explore some of the most pertinent issues in the field of AI ethics:
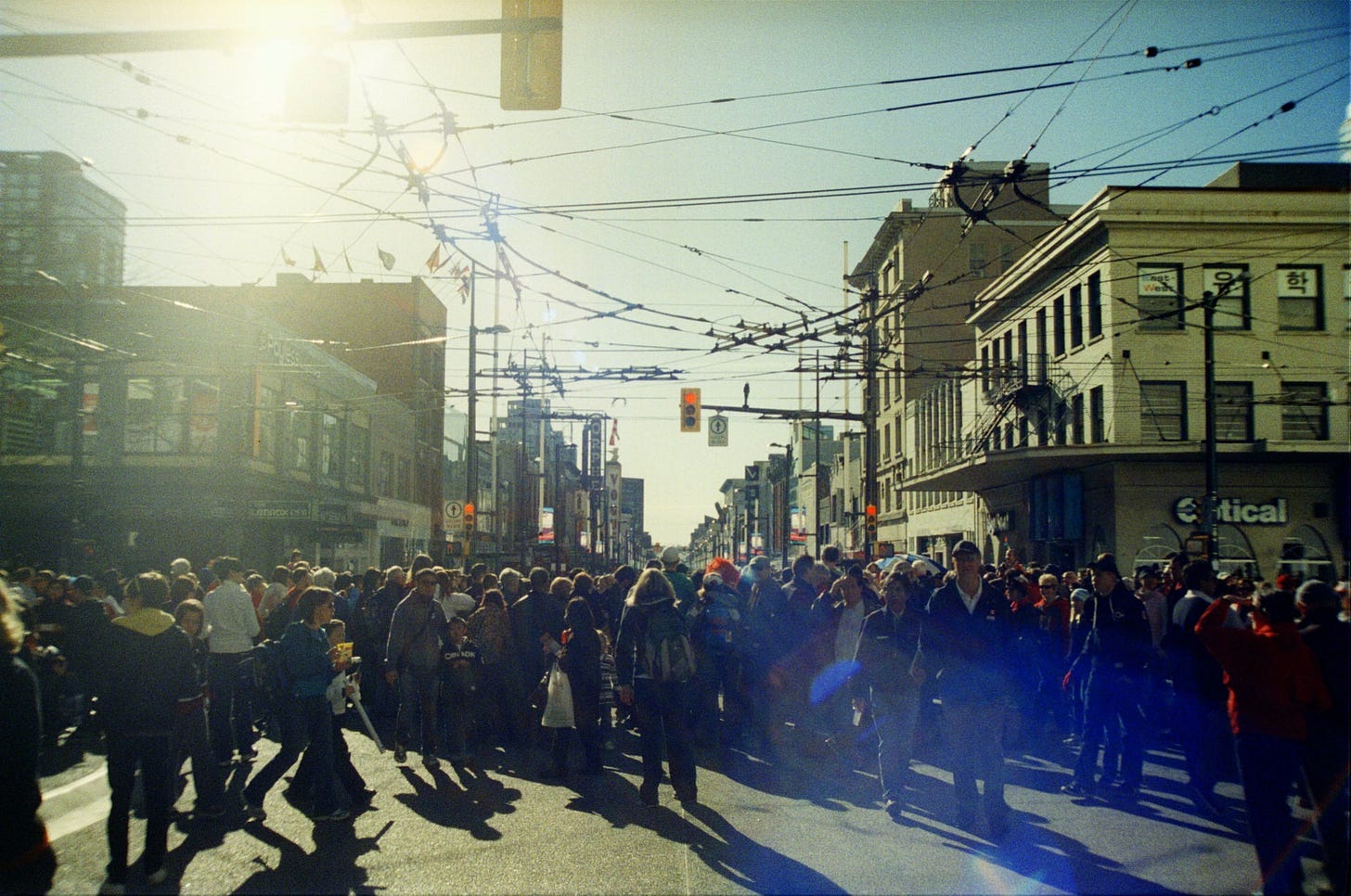
Photo by Randy Laybourne on Unsplash
The Short Anthropological Guide to the Study of Ethical AI by Alexandrine Royer
In the 1950s, computer scientist Alan Turing designed a test; a machine would be considered ‘intelligent’ if a human interacting with it could not tell whether it was a person or a machine. It was the first step in the development of what would become the field of Artificial Intelligence (AI), a term first coined by John McCarthy at the seminal Dartmouth summer research project in 1956. In the short span of seventy years, the production of intelligent machines has evolved beyond the scope of human imagination. No longer limited to sci-fi aficionados and the scientific community, artificial intelligence has become ubiquitous in each of our lives. We interact with AI, whether knowingly or unknowingly, daily when using our phones, digital assistants, applying for loans, undergoing medical treatment, or just browsing the web. Companies across the board are scrambling to adopt AI and machine learning technology. Opinions, hopes, and fears ranging from utopia to catastrophe accompany this growing proximity with artificial intelligence systems – Stephen Hawkings’ infamously prophesied that AI could spell the end of humanity.
The development of technology has brought on a series of significant advances, such as improved medical imaging, new video communication technology, 3-D printed affordable homes, drones for service deliveries in conflict areas, etc. AI has proven it can produce immense social good. However, every new technology comes with considerable caveats, which we tend to observe once set in motion. The rapid expansion of consumer Internet over the past two decades has led to the explosion of algorithmic decision-making and predictions on individual consumers and behaviour. Before we could even agree to the collection of our data, private corporations, banks, and the public sector used it to make crucial decisions on our lives. Over the years, data scientists and social scientists have started to signal incidents where algorithms violate fundamental social norms and values. Algorithms trampled on notions of privacy, fairness, equality, and were revealed to be prone to manipulations by its users. These problems with algorithms have led researchers Michael Kerns and Aaron Roth to state that “it is less a concern about algorithms becoming more powerful than humans, and more about them altering what it means to be human in the first place.”
Over the next few years, society as a whole will need to address what core values it wishes to protect when dealing with technology. Anthropology, a field dedicated to the very notion of what it means to be human, can provide some interesting insights into how to cope and tackle these changes in our Western society and other areas of the world. It can be challenging for social science practitioners to grasp and keep up with the pace of technological innovation, with many being unfamiliar with the jargon of AI. This short guide serves as both an introduction to AI ethics and social science and anthropological perspectives on the development of AI. It intends to provide those unfamiliar with the field with an insight into the societal impact of AI systems and how, in turn, these systems can lead us to rethink how our world operates.
Before delving into anthropology’s contributions to AI, a brief overview of the ethical issues in technology will help situate some of the critical failures of algorithmic design and their integration into high-stakes decision-making areas. Exploring the limitations of ethically fine-tuned, or better-behaved, algorithms in the areas of privacy, fairness, and user model manipulation elucidates how ethical AI requires input from the social sciences. The current controversies in which technology giants are enmeshed show that society cannot entrust Silicon Valley entirely to pave the way to produce ethical AI. Therefore, anthropological studies can assist in determining new avenues and perspectives on how to expand the development of ethical artificial intelligence and machine learning systems. Ethnographic observations have already been used to understand the social contexts in which these systems are designed and deployed. By looking beyond the algorithm and turning to the humans behind it, we can start to critically examine the broader social, economic and political forces at play in the rapid rise of AI and ensure that no population nor individuals are left to bear the negative consequences of technological innovation.
To delve deeper, read the full article here.
From our learning communities:
Research that we covered in the learning communities at MAIEI this week, summarized for ease of reading:
Watch this space for more content soon!
Articles:
Let’s look at highlights of some recent articles that we found interesting at MAIEI:
The U.S. Needs a National Data Service (Scientific American)
The article provides 3 primary recommendations on how a National Data Service might be created and potential benefits associated with its establishment. But before diving into that, it’s important to consider why we even need such a service in place. As frequent readers of this newsletter, you’re no stranger to the requirement of large corpora of data to train machine learning systems. It is without a doubt an invaluable resource that nations are trying their best to structure and make available to glean insights to inform policy. There have been numerous efforts in the US for example where they have attempted to put in place requirements for policymaking to become more data-driven.
With the US Census 2020 this year, the need for data collection has never been more front and center. The pandemic has made in-person collection hard, yet the census data collection process can’t be halted because the insights from this data is used to inform all sorts of downstream decisions including representation in government. So, why do we need a national data service then? (And this applies to not just the US, but other countries too!) The article makes the case that it would help to harmonize, measure, and validate the data that is collected for example in the census to ensure its accuracy; “Trust, but verify.” The recommendations made by the article are: empowering local governments to utilize existing data in their decision making, ensure replicability and comparability across the nation, and finally ensuring privacy and confidentiality of the data collected to engender trust from people. These are crisp and provide a great starting point for countries to consider as a part of their national AI strategies, since without data, it is really hard (at least in the current paradigm of AI) to build meaningful systems.
Councils scrapping use of algorithms in benefit and welfare decisions (The Guardian)
The recent A-levels grades fiasco in the UK highlighted how automated systems can go wrong in a very public way. Chants on the street led to the government having to make a U-turn and get rid of the decisions that were offered by the system. This article highlights how this has triggered, and builds on a trend of, abandonment of the use of automated systems in things like welfare decisions, visa processing, and more. They point to examples in the UK where councils have now given up, for example, the use of such systems to determine if certain claims for welfare benefits are fraudulent. This led to delays for some people without their knowledge, when processing officers were looped in to do an extra review of the application. There was also the case recently when visa applications were denied disproportionately for people who were not white.
In some cases, the organizations using these systems have also realized that they don’t get too many benefits in terms of speed or efficiency in using these systems. They also realized that some of the outcomes are antithetical to the very reason for their existence which has led to abandonment. But, as the article points out, there are still companies that are pitching their products and services to government agencies since these are lucrative contracts. The article argues that having greater transparency in this process along with the push for including public consultation as a part of this process might aid in alleviating some of these concerns.
Algorithms Are Automating Fascism. Here’s How We Fight Back (Vice)
Facial recognition technology is unfortunately utilized in subversive ways, often without transparency, by law enforcement which is one of the drivers for the recent backlash against this technology. While there are glaring issues with bias, and other concerns, the article highlights a recent case where someone faced a siege from police officers after facial recognition was applied to their social media accounts to identify them. Yet, technology is only partly to blame here; systemic problems of racism and brutality underscore the importance of looking at these systems with a sociotechnical lens to ensure that we don’t fall into the situation of analyzing problems in a unidimensional manner. These systems merely amplify the existing biases of the people and institutions that build the systems.
Pointing to an example of a proposed rule a few months ago that instructed federally run shelters to eject transwomen based on features like facial hair, Adam’s apple, etc. This is particularly problematic because these features are well within the capabilities of existing machine vision systems and hence prone to automation. A project highlighted in the article called the White Collar Crime Risk Zones flipped the script on over policing in ethnic and minority neighborhoods via predictive policing by spotlighting zones where financial crimes are likely to take place to show how predictive policing leads to amplification of systemic faults.
A proposed mechanism for rebelling against these injustices is, for example, to use anti-surveillance makeup patterns or wear masks to combat facial recognition systems. The problem is that this a cat-and-mouse game where the surveillance systems learn how to circumvent such protection measures with more training data and hence don’t provide a lasting solution.
The current demand for seemingly magical solutions, based on flawed methods, will always be there which is something that the larger public and those with decision-making powers need to wake up to and realize the flaws. As the article concludes, we need to attack these problems through all vectors possible in the hopes of preventing them from realizing a dystopic future.
Big Oil Faded. Will Big Tech? (NY Times)
The article conveys an eye-opening fact that it was only 10 years ago that Exxon Mobil was the most valuable company in the world and our world was dominated by large energy companies. A key vulnerability of these energy companies that has led to their decline is that they weren’t nimble enough to adapt to the changing energy landscape in the move away from fossil fuels. They are also hostage to the demand forces for the product that are out of their control. It seems that the technology companies don’t face that same struggle. With the technology companies now possessing more market capitalization than the entire European stock market, it calls for a moment to pause and reflect on the magnitude of power that these companies have.
They are able to shape policy, dictate what constitutes anti-trust, our notions of privacy, and to some extent how we live our lives since they are the conduits through which we manage our digital existence. This is exacerbated when governments don’t step up in their role to regulate these players and they run amok reshaping the landscape to suit their monetary goals. Companies like Apple are pushing for changes to how users’ personal data is protected but as the article points out, it takes a lot of effort on the part of the users to check off the right boxes that enable them to reap the benefits of enhanced privacy protections. We are all presented with an interesting conundrum in the sense that we have these large companies who might shape policies to benefit end-users, especially in the absence of government efforts, yet the fact that they have this power in the first place to so significantly impact our lives is in itself quite dangerous.
Google Offers to Help Others With the Tricky Ethics of AI (Wired)
An interesting moniker, Ethics-as-a-service (EaaS), is used in this article to aptly sum up what Google is trying to do with this latest announcement. While there is a fantastic team of folks doing very meaningful research at PAIR (People + AI Research) at Google, when it comes to outsourcing ethics to a for-profit organization, many critics are right to raise concerns about what this means in practice. There are certainly a lot of valuable lessons learned by Google over the past few years with its AI ethics board fiasco, wrongly flagging Black people in photos as gorillas, and others. Yet, there might be a better way to direct these lessons and resources rather than spinning it off as a consultancy.
Specifically, it might be useful to share these ideas openly with others through various channels and creating courses that might be undertaken by people who are in the field deploying these systems. Another avenue might be to fund efforts by other non-profit organisations that are doing work in this space rather than duplicating efforts. But, as the article points out, it might just be a mechanism that the company is using to distinguish itself in a crowded field of actors.
Are Robots Eating Our Jobs? Not According To AI (Forbes)
A few important points made in this article: humans aren’t going anywhere since the current dominant paradigm of machine learning requires large swathes of human efforts to enable their functioning and even if ML systems are good at making predictions, we still need humans to make judgements and act on those predictions. While there are widely varying estimates of the number of jobs that will be lost, a thing that is important to keep in mind is that these were estimates that were made prior to the start of the pandemic and didn’t anticipate the pace of adoption of automation which has quickened.
This might mean that more jobs are lost but some labor economists and keen watchers of the space argue that there is room for new jobs that will be created to support the development of AI systems, through things such as data labelling services. One would be remiss to not mention how forecasting a decade away has severe flaws. There isn’t a doubt that the estimates made on the labor impacts of AI are underscored by research but as Philip Tetlock mentions in Superforecasters, anything past 18 months severely degrades in quality because of the complexity of the world that we inhabit coupled with interactions and technological changes that have the potential to disrupt even the best-laid plans. As we emphasize at MAIEI when it comes to the future of work, the one definite thing that we can do is to remain adaptable and adopt life-long learning to the extent that is possible given our individual circumstances. Beyond that, it is hard to accurately forecast and plan for the pace of technological progress and therefore the accompanying societal changes.
From elsewhere on the web:
Things from our network and more that we found interesting and worth your time.
Could machine learning help bring marginalized voices into historical archives?
Work by our founder Abhishek Gupta was featured by VentureBeat talking about the proposal to use machine learning to build comprehensive archives that could bridge gaps in cultural understanding, knowledge, and views. It asserts that including more voices in archival processes — with the help of machine learning — can have positive effects on communities, particularly those archivists have historically marginalized.
Contact tracing has grown in popularity as a promising solution to the COVID-19 pandemic. The benefits of automated contact tracing are two-fold. Contact tracing promises to reduce the number of infections by being able to: 1) systematically identify all of those that have been in contact with someone who has had COVID; and, 2) ensure those that have been exposed to the virus do not unknowingly infect others. "COVI" is the name of a recent contact tracing app developed by Mila and was proposed to help combat COVID-19 in Canada. The app was designed to inform each individual of their relative risk of being infected with the virus, which Mila claimed would empower citizens to make informed decisions about their movement and allow for a data-driven approach to public health policy; all the while ensuring data is safeguarded from governments, companies, and individuals. This article will provide a critical response to Mila's COVI White Paper. Specifically, this article will discuss: the extent to which diversity has been considered in the design of the app, assumptions surrounding users' interaction with the app and the app's utility, as well as unanswered questions surrounding transparency, accountability, and security. We see this as an opportunity to supplement the excellent risk analysis done by the COVI team to surface insights that can be applied to other contact- and proximity-tracing apps that are being developed and deployed across the world. Our hope is that, through a meaningful dialogue, we can ultimately help organizations develop better solutions that respect the fundamental rights and values of the communities these solutions are meant to serve.
This document posits that, at best, a tenuous case can be made for providing AI exclusive IP over their "inventions". Furthermore, IP protections for AI are unlikely to confer the benefit of ensuring regulatory compliance. Rather, IP protections for AI "inventors" present a host of negative externalities and obscures the fact that the genuine inventor, deserving of IP, is the human agent. This document will conclude by recommending strategies for WIPO to bring IP law into the 21st century, enabling it to productively account for AI "inventions". Theme: IP Protection for AI-Generated and AI-Assisted Works Based on insights from the Montreal AI Ethics Institute (MAIEI) staff and supplemented by workshop contributions from the AI Ethics community convened by MAIEI on July 5, 2020.
From the archives:
Here’s an article from our blogs that we think is worth another look:
3 activism lessons from Jane Goodall you can apply in AI Ethics
Jane Goodall, one of the world’s most influential and beloved advocates for nature conservation, delivered the 2019 Beatty Lecture at McGill University on Thursday, September 26. Dr. Goodall delivered her first Beatty Lecture in 1979, where she shared stories about her groundbreaking research on chimpanzee behaviour in Gombe, Tanzania. To celebrate the 65th anniversary of the Beatty Lecture, Dr. Goodall returned to McGill forty years later to talk about the critical need for environmental stewardship and the power each individual has to bring about change. She is the first repeat lecturer in the Beatty’s history.
To delve deeper, read the full article here.
Guest contributions:
If you’ve got an informed opinion on the impact of AI on society, consider writing a guest post for our community — just send your pitch to support@montrealethics.ai. You can pitch us an idea before you write, or a completed draft.
Events:
As a part of our public competence building efforts, we host events frequently spanning different subjects as it relates to building responsible AI systems, you can see a complete list here: https://montrealethics.ai/meetup
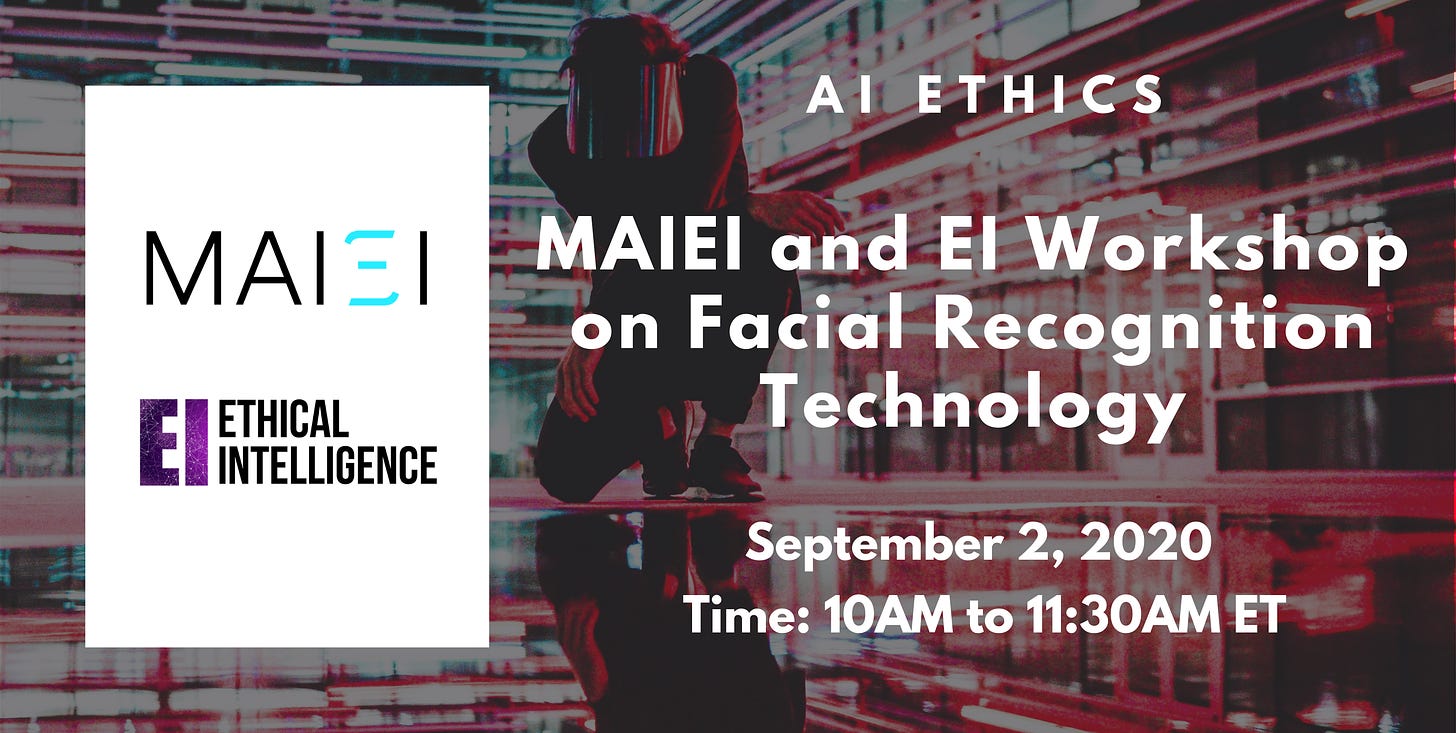
Facial Recognition Technology Workshop (AI Ethics)
September 2, 10 AM - 11:30 AM ET (Online)
You can find all the details on the event page, please make sure to register as we have limited spots (because of the online hosting solution).
Signing off for this week, we look forward to it again in a week! If you enjoyed this and know someone else that can benefit from this newsletter, please share it with them!
If you have feedback for this newsletter or think there is an interesting piece of research, development or event that we missed, please feel free to email us at support@montrealethics.ai