AI Ethics #24: Science fiction to teach AI ethics, unnoticed cognitive biases, post-pandemic university, face-mask recognition, political databases, and more ...
Machine translation for African languages, grassroot efforts to combat misinformation, AI regulations for children, NSCAI responsible AI principles and more from the world of AI Ethics!
Welcome to the twenty-fourth edition of our weekly newsletter that will help you navigate the fast changing world of AI Ethics! Every week we dive into research papers that caught our eye, sharing a summary of those with you and presenting our thoughts on how it links with other work in the research landscape. We also share brief thoughts on interesting articles and developments in the field. More about us on: https://montrealethics.ai/about/
If someone has forwarded this to you and you want to get one delivered to you every week, you can subscribe to receive this newsletter by clicking below:
Summary of the content this week:
In research summaries this week, we look into a participatory approach to document machine translation for African languages, teaching AI ethics using science fiction, the unnoticed bias that is secretly shaping the AI agenda, and what a co-designed post-pandemic university might look like where there is a continual learning and participatory approach to address the future of work.
In article summaries this week, we look into who should be held accountable when something goes wrong in using AI for healthcare applications, what political databases know about you, grassroots efforts to combat the spread of COVID-19 related misinformation, why we need more protection for children when they are the targets of AI applications, and face mask recognition.
In featured work from our staff this week, Abhishek and Victoria published a piece for the MIT Technology Review on how we might be repeating some of society’s classic mistakes in the domain of AI ethics, our report on publication norms for responsible AI prepared for Partnership on AI, a call for a workshop being organized on navigating the broader impacts of AI research, and finally a call for submissions for a NeurIPS 2020 workshop being co-organized by Abhishek.
In upcoming events, we will be hosting a workshop on the key considerations in building responsible AI systems in partnership with the NSCAI. Scroll to the bottom of the email for more information.
MAIEI Learning Community:
Interested in working together with thinkers from across the world to develop interdisciplinary solutions in addressing some of the biggest ethical challenges of AI? Join our learning community; it’s a modular combination of reading groups + collaborating on papers. Fill out this form to receive an invite!
AI Ethics Concept of the week: ‘Regression’
Regression analysis is an approach to machine learning that teaches a machine learning model about a causal relationship between variables.
Learn about the relevance of regression to AI ethics and more in our AI Ethics Living dictionary. 👇
Explore the Living Dictionary!
Consulting on AI Ethics by the research team at the Montreal AI Ethics Institute
In this day and age, organizations using AI are expected to do more than just create captivating technologies that solve tough social problems. Rather, in today’s market, the make or break feature is whether organizations using AI espouse concepts that have existed since time immemorial, namely, principles of morality and ethics.
The Montreal AI Ethics Institute wants to help you ‘make’ your AI organization. We will work with you to analyze your organization and/or product offerings for moral and ethical weaknesses and make sure your business model is air tight. By undergoing a thorough, multidisciplinary review of your AI tool, we will provide ethical, social, and technical feedback on your work and research, which will allow you to proactively address your own blindspots and maximize your potential before ever undergoing a third party ethics review.
To find out more, please take a look at this page.
Research:
Let's look at some highlights of research papers that caught our attention at MAIEI:
Teaching AI Ethics Using Science Fiction by Emmanuelle Burton, Judy Goldsmith, Nicholas Mattei
Having caused at least some of the fears surrounding AI, science fiction is being repurposed as the method of increasing the engagement of computer science students in the ethical space. Given how technologists do not work in an ethical vacuum, science fiction’s ability to present current situations in unfamiliar settings and encourage the consideration of different viewpoints represent this practice as ethics’ lifeline towards being properly considered. Ethics can no longer be seen as something to be ticked off the list after one class by technology practitioners, and science fiction can present ethics in a way to strongly prevent that. The more students that engage in this space the better, and science fiction poses a surprising amount of potential.
To delve deeper, read our full summary here.
Lanfrica: A Participatory Approach to Documenting Machine Translation Research on African Languages by Chris C. Emezue, Bonaventure F.P. Dossou
It is no secret that English has dominated the machine learning landscape. Yet, multilingual researchers worldwide are trying to change the narrative and put their language on the digital map. With machine learning research efforts springing up across the continent, which is home to over 1500 languages, it is difficult to coordinate and keep track of current research happening in silos. Emezue et Dossou found that a significant hindrance to the advancement of MT research on African languages is the lack of a central database that gives potential users quick access to benchmarks and resources and enables them to build comparative models. The authors propose an open-source and publicly available database, titled Lanafrica, that will allow users from the scientific and non-scientific community to catalog and track the latest research on machine learning developments in African languages.
To delve deeper, read our full summary here.
What we are thinking:
Op-eds and other work from our research staff that explore some of the most pertinent issues in the field of AI ethics:
The Co-Designed Post-Pandemic University: A Participatory and Continual Learning Approach for the Future of Work by Abhishek Gupta and Connor Wright
The pandemic has shattered the traditional enclosures of learning. The post-pandemic university (PPU) will no longer be contained within the 4 walls of a lecture theatre, and finish once students have left the premises. The use of online services has now blended home and university life, and the PPU needs to reflect this. Our proposal of a continuous learning model will take advantage of the newfound omnipresence of learning, while being dynamic enough to continually adapt to the ever-evolving virus situation. Universities restricting themselves to fixed subject themes that are then forgotten once completed, will miss out on the ‘fresh start’ presented by the virus.
To delve deeper, read the full article here.
The Unnoticed Cognitive Bias Secretly Shaping the AI Agenda by Camylle Lanteigne, AI Ethics Researcher and Research Manager at MAIEI and Ethics Analyst, Algora Lab
This explainer was written in response to colleagues’ requests to know more about temporal bias, especially as it relates to AI ethics.It begins with a refresher on cognitive biases, then dives into: how humans understand time, time preferences, present-day preference, confidence changes, planning fallacies, and hindsight bias.
To delve deeper, read the full article here.
Articles:
Let’s look at highlights of some recent articles that we found interesting at MAIEI:
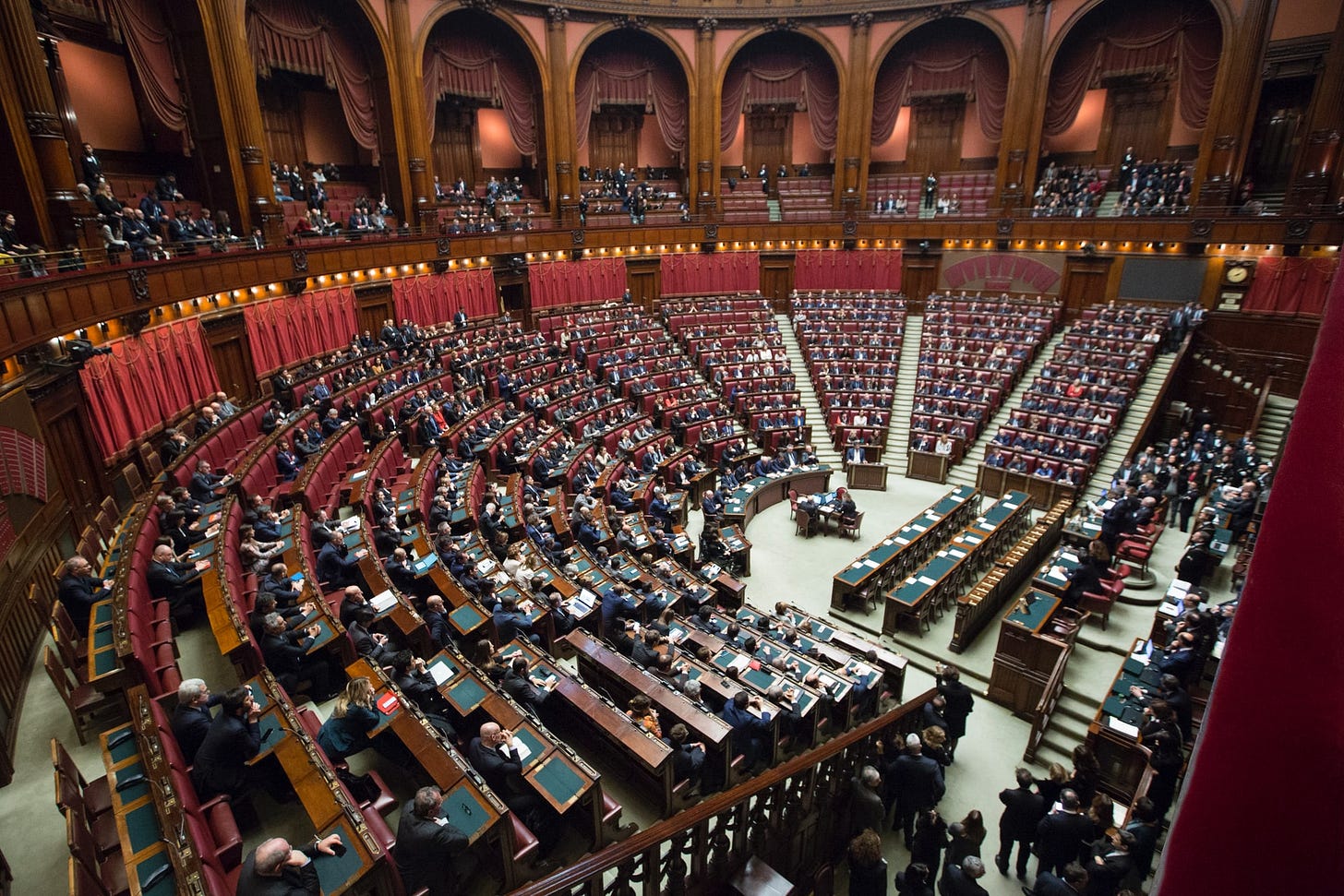
Photo by Marco Oriolesi on Unsplash
When AI in healthcare goes wrong, who is responsible? (Quartz)
As covered by the Montreal AI Ethics Institute before, when it comes to taking a look at the use of automated technology in healthcare, there are many concerns that abound which need to be addressed proactively. In the use of automated systems, where it is clear that product liability applies, there the cases that need to be resolved are fairly obvious (with the usual caveats) as was the case for Da Vinci Robotics and mechanical errors with their surgical assistant robots. But, in the case where there might be software issues in terms of diagnostics, it is less clear on what can be done.
Some advocate that everyone in the value chain be held liable, others advocate for assigning strict liability on the manufacturer, and yet others who advocate for the use of liability mechanisms that place the onus on those who are utilizing the system since they are the final arbiters of use. In cases where automated systems have very high accuracies and a doctor makes another decision, there is an increased legal risk that they take which might disincentivize them from ever disagreeing with the machine. That said, if such legal holes continue to exist, not only will the adoption of these systems slow down but it will also erode trust and patients will be the ultimate losers in this scenario since they are stripped down to even fewer mechanisms for recourse.
As we dive deeper into integrating these systems, it is clear that we need to have stronger accountability measures in place that are enforceable and unambiguous.
Explainer: What do political databases know about you? (MIT Technology Review)
When looking at things like the mosaic effect and the ability to collate large datasets about a person, we notoriously have very little information to inform us as to how we are targeted. With the 2020 election in the US, knowing the targeting mechanisms on the internet is essential to ensuring the integrity of our democracy. Political campaigns engage in persistent messaging to modify behaviour, relying on parallel and continuous texts, calls, and social media messages to sway voting patterns.
Research has shown that most US adults are present in these datasets, with data collated from social media, credit card records, and other public information. Some attributes are inferred from statistical norms but their accuracy is questionable, sometimes even to the point that it hinders their usefulness to the political campaign targeting. But, such data still through mechanisms like A/B testing can be refined over time to the point that it is hyper-specific to the user and ostensibly successful in tweaking their behaviour.
In terms of who is allowed to use the targeting tools on platforms like Facebook, there isn’t complete clarity on that, but it would appear that the rules apply in an uneven fashion and some political organizations are able to skirt scrutiny and evade the consequences of breaking some of the regulations around campaign finance spending. The polluted landscape of information also plays into the hands of those who want to utilize these tools in a savvy manner. This is only worsened by the disparity between the candidates with varying levels of technology savvy who are able to use this pollution to their advantage.
A Grassroots Effort to Fight Misinformation During the Pandemic (Scientific American)
The WHO mentioned that we are facing an infodemic at the same time as the pandemic that has hindered our ability to effectively combat the spread of the virus because of fragmented and conflicting advice on how to best protect ourselves and those around us. Specifically, the rampant misinformation has caused a lot of grief in terms of lives that could have been saved. This initiative mentioned in the article by the Federation of American Scientists (FAS) has been a public and successful demonstration in the power of grassroots initiatives.
With their service to answer the FAQ on this issue through automated means that have answers sourced from domain experts, they have made a true positive impact in the information discourse on this topic. By supplementing this approach with the ability to “learn” over time as more and more people parse the existing database and then asking questions directly for those that haven’t been answered, the information only becomes richer over time.
Finally, the approach undertaken by the FAS and their partner organizations showcase how crowdsourced mechanisms can be mobilized and scaled to meet emergent community needs, something that the field of AI Ethics can also borrow from. To a certain extent, the approach of the Montreal AI Ethics Institute with the Living Dictionary has been the same.
Why kids need special protection from AI’s influence (MIT Technology Review)
Principles abound in the domain of AI ethics, ever since more people started paying attention to them. Yet, some disadvantaged or neglected groups who have special needs haven’t been given due consideration. This article highlights a couple of such efforts: one from UNICEF and the other from the Beijing Academy of Artificial Intelligence (BAAI) to provide additional considerations from an ethical standpoint when going through the design, development, and deployment of AI systems that will be used for children.
AI-enabled systems are used for children in the context of toys with which they interact which might record their personal data, decisions about whether their parents are deemed fit, and most recently in the grades that they may have secured and thus determining their educational and career trajectories. The efforts from UNICEF are meant to augment existing guidelines with specific considerations for interactions with children. An interesting consideration was to make the guidelines explainable to children so that it can empower them with more agency in determining their futures. More education about how AI operates and what capabilities and limitations that it has will actually help to steer the development in a direction that does actually align with their interests and values.
The next steps in both of these initiatives is to run pilot development programs that actually battle-test these guidelines to see how they actually protect the rights of children in practice. Working groups with major industry players is another avenue to source feedback so that the guidelines are practical and will make a tangible difference.
Face-mask recognition has arrived—for better or worse (National Geographic)
The article makes for a fascinating read because it highlights some of the tensions in the field when it comes to using facial recognition technology, especially when it is framed in the context of the benefits that it might provide as it helps to combat the pandemic.
Some developers are arguing that mask recognition, since it differs from facial recognition, doesn’t have many privacy concerns since they are only considering the presence or absence of masks and are doing it purely for the purposes of combating non-compliance. Yet, pervasive monitoring, be that for detecting crime or wearing masks still creates an unnerving context in a physical space, altering the relationships that people have with the spaces around them. This is also problematic since it has the potential to open doors to other forms of surveillance which can be guised under the pretence of doing public good.
One of the companies mentioned in this article has talked about deploying their technology in stealth mode. This is concerning since it inherently creates an asymmetric power dynamic that encourages one person/organization’s viewpoint as superior to others. Asking critical questions should be something that is done more publicly and companies who are in the design, development, and deployment of such technologies should be comfortable in responding to these questions in the interest of transparency, especially when they frame its deployment as a public good.
From elsewhere on the web:
Things from our network and more that we found interesting and worth your time.
AI Ethics groups are repeating one of society’s classic mistakes by Abhishek Gupta and Victoria Heath for the MIT Technology Review
"The problem: AI systems have repeatedly been shown to cause problems that disproportionately affect marginalized groups while benefiting a privileged few. The global AI ethics efforts underway today—of which there are dozens—aim to help everyone benefit from this technology, and to prevent it from causing harm. These groups are well-intentioned and are doing worthwhile work.
However… Without more diverse geographic representation, they’ll produce a global vision for AI ethics that reflects the perspectives of people in only a few regions of the world, particularly North America and northwestern Europe. If unaddressed, they risk developing standards that are, at best, meaningless and ineffective across all the world’s regions. At worst, these flawed standards will lead to more AI systems and tools that perpetuate existing biases and are insensitive to local cultures."
To delve deeper, read the full article here.
The history of science and technology shows that seemingly innocuous developments in scientific theories and research have enabled real-world applications with significant negative consequences for humanity. In order to ensure that the science and technology of AI is developed in a humane manner, we must develop research publication norms that are informed by our growing understanding of AI's potential threats and use cases. Unfortunately, it's difficult to create a set of publication norms for responsible AI because the field of AI is currently fragmented in terms of how this technology is researched, developed, funded, etc. To examine this challenge and find solutions, the Montreal AI Ethics Institute (MAIEI) collaborated with the Partnership on AI in May 2020 to host two public consultation meetups. These meetups examined potential publication norms for responsible AI, with the goal of creating a clear set of recommendations and ways forward for publishers.
In its submission, MAIEI provides six initial recommendations, these include: 1) create tools to navigate publication decisions, 2) offer a page number extension, 3) develop a network of peers, 4) require broad impact statements, 5) require the publication of expected results, and 6) revamp the peer-review process. After considering potential concerns regarding these recommendations, including constraining innovation and creating a "black market" for AI research, MAIEI outlines three ways forward for publishers, these include: 1) state clearly and consistently the need for established norms, 2) coordinate and build trust as a community, and 3) change the approach.
To delve deeper, read the full report here.
A very interesting workshop related to the above idea organized by some of the same folks behind the publication norms work from Partnership on AI among others titled Navigating the Broader Impacts of AI Research that will be taking a look at:
Mechanisms of ethical oversight in AI research
Challenges of AI research practice and responsible publication
Collective and individual responsibility in AI research
Anticipated risks and known harms of AI research
Our founder, Abhishek Gupta, is co-organizing the following workshop that is now accepting submissions: The ML-Retrospectives, Surveys & Meta-Analyses @ NeurIPS 2020 Workshop is about reflecting on machine learning research. This workshop is a new edition of the previous Retrospectives Workshops at NeurIPS’19 and ICML’20 respectively. While earlier the focus of the workshop was primarily on Retrospectives, this time the focus is on surveys & meta-analyses. The enormous scale of research in AI has led to a myriad of publications. Surveys & Meta-Analyses meet the need of taking a step back and looking at a sub-field as a whole to evaluate actual progress. However, we will also accept retrospectives.
In conjunction with NeurIPS, the workshop will be held virtually. Please see our schedule for details.
To delve deeper, take a look at the workshop website here.
From the archives:
Here’s an article from our blogs that we think is worth another look:
Evasion Attacks Against Machine Learning at Test Time by Battista Biggio, Igino Corona, Davide Maiorca, Blaine Nelson, Nedim Srndic, Pavel Laskov, Giorgio Giacinto, and Fabio Roli
Machine learning adoption is widespread and in the field of security, applications such as spam filtering, malware detection, and intrusion detection are becoming increasingly reliant on machine learning techniques. Since these environments are naturally adversarial, defenders cannot rely on the assumption that underlying data distributions are stationary. Instead, machine learning practitioners in the security domain must adopt paradigms from cryptography and security engineering to deal with these systems in adversarial settings.
To delve deeper, read the full article here.
Guest contributions:
If you’ve got an informed opinion on the impact of AI on society, consider writing a guest post for our community — just send your pitch to support@montrealethics.ai. You can pitch us an idea before you write, or a completed draft.
Events:
As a part of our public competence building efforts, we host events frequently spanning different subjects as it relates to building responsible AI systems, you can see a complete list here: https://montrealethics.ai/meetup
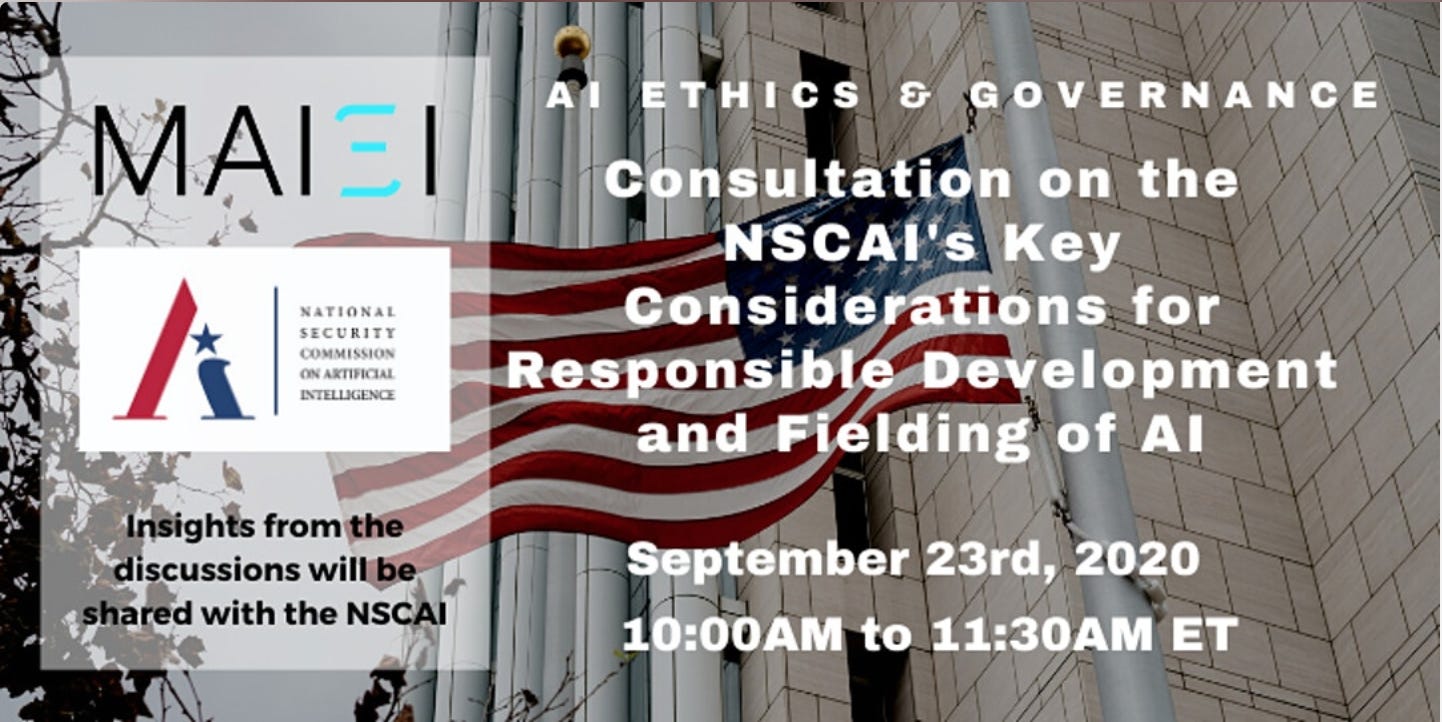
MAIEI Consultation: NSCAI's Key Considerations for Responsible AI
September 23, 10 AM - 11:30 AM ET (Online)
You can find all the details on the event page, please make sure to register as we have limited spots (because of the online hosting solution).
Signing off for this week, we look forward to it again in a week! If you enjoyed this and know someone else that can benefit from this newsletter, please share it with them!
If you have feedback for this newsletter or think there is an interesting piece of research, development or event that we missed, please feel free to email us at support@montrealethics.ai