
Discover more from The AI Ethics Brief
AI Ethics #6 : Radioactive data, attacking deep RL, steering AI progress, sucker's list, AI ethics in marketing and more ...
Our sixth weekly edition covering research and news in the world of AI Ethics
Welcome to the sixth edition of our weekly newsletter that will help you navigate the fast changing world of AI Ethics! Every week we dive into research papers that caught our eye, sharing a summary of those with you and presenting our thoughts on how it links with other work in the research landscape. We also share brief thoughts on interesting articles and developments in the field. More about us on: https://montrealethics.ai/about/
If someone has forwarded this to you and you want to get one delivered to you every week, you can subscribe to receive this newsletter by clicking below
As we continue to cope with the changes in our work and life with the ongoing pandemic, this week’s newsletter brings you a brief respite from all the COVID-19 news looking at interesting ideas like radioactive data which helps in tracing if data points have been used in the training of machine learning models, how we can steer the progress in AI leaning on both economic and ethical values, and how we can build more robust deep reinforcement learning systems by taking an adversarial approach among others ideas.
Stay safe and healthy and enjoy this week’s content!
Research:
Let's look at some highlights of research papers that caught our attention at MAIEI:
Integrating Ethical Values and Economic Value to Steer Progress in Artificial Intelligence by Anton Korinek
When pushing for adoption of ethical values and guidelines in the development and deployment of AI systems, we often face resistance by various parts of an organization with rationalizations ranging from how it might negatively impact the business to a deferment strategy making largely hollow promises to address those concerns at an indeterminate time in the future. This paper presents some of the conflicts that arise between the economic and ethical perspectives and how taking a single-minded approach will lead to solutions that are skewed and can negatively impact people. While there are a wide variety of economic concerns, what caught our eye was the elucidation of the disparity in economic values which are usually expressed as single dimensional metrics that are easily quantifiable and amenable to decision making and reasoning by humans.
This is contrasted with the multi-dimensional nature of ethical values which are subjective, abstract and require significant effort to reason and hence aren't very amenable to justifiable decision making, a little bit akin to the black box nature of deep learning systems, which is encoded in our brains and isn't easily explainable. The paper also highlights how traditional economic theory and ethical theories in isolation stand at loggerheads and squaring them within the cultural context of a society is crucial to arriving at framework that helps to steer the progress in AI such that it can bring as much societal benefits as possible. It concludes with a look at the far future where we might have superintelligence and how humans might be replaced akin to oxen in the Industrial Revolution who found themselves out competed by machines whose costs were lower than their benefits. To avoid such a fate where we find ourselves snared again in a Malthusian Trap, we have to take an active role in steering the progress of AI rather than succumbing to technological-fatalism.
To delve deeper, read our full summary here.
Suckers List: How Allstate’s Secret Auto Insurance Algorithm Squeezes Big Spenders by Maddy Varner and Aaron Sarkin
Ever wondered what goes into pricing your insurance premiums the way they are and why they are different from others who you know, even when you share a largely similar risk profile? This investigative piece from The Markup delved deep into the discriminatory pricing practice from the US auto insurer Allstate that utilized purportedly complex models to offer fairer prices to their consumers when in reality their model’s complexity boiled down to 2 key things: how price-inelastic the consumer was and how unwilling they are to switch insurance providers. While price discriminaton has been done in a variety of industries and has often been shrugged off as the state of affairs, this case has been particularly impactful because it is doing so in the domain of something that is an essential service in being able to own and operate a vehicle.
Additionally, the impacts were disproportionately on those who had less of an ability to adjust to the changing circumstances, often where the difference of a few hundred dollars meant fewer meals on the table. Opacity is often practiced under the legal cover of wanting to protect privacy and trade secrets, this move by Allstate gave a rare insight into their operating models in part because of the demands from regulators to justify and support their claims for revising pricing policies. The documentation that was provided by the company showed how increases weren’t necessarily limited, in places where they had been overcharging, the discounts offered were only minimal creating a distortion where they limited their downside by offering pennies on the dollar while maximizing their upside. Resigning to this unfair practice, consumers are forced to bear the burden of having to constantly check prices from competitors to ensure that they aren’t being price-gouged. Regulators, at the moment, stand toothless because they lack the data and aren’t asking the right questions that would unearth some of these unfair practices.
To delve deeper, read our full summary here.
Articles:
Let’s look at highlights of some recent articles that we found interesting at MAIEI:
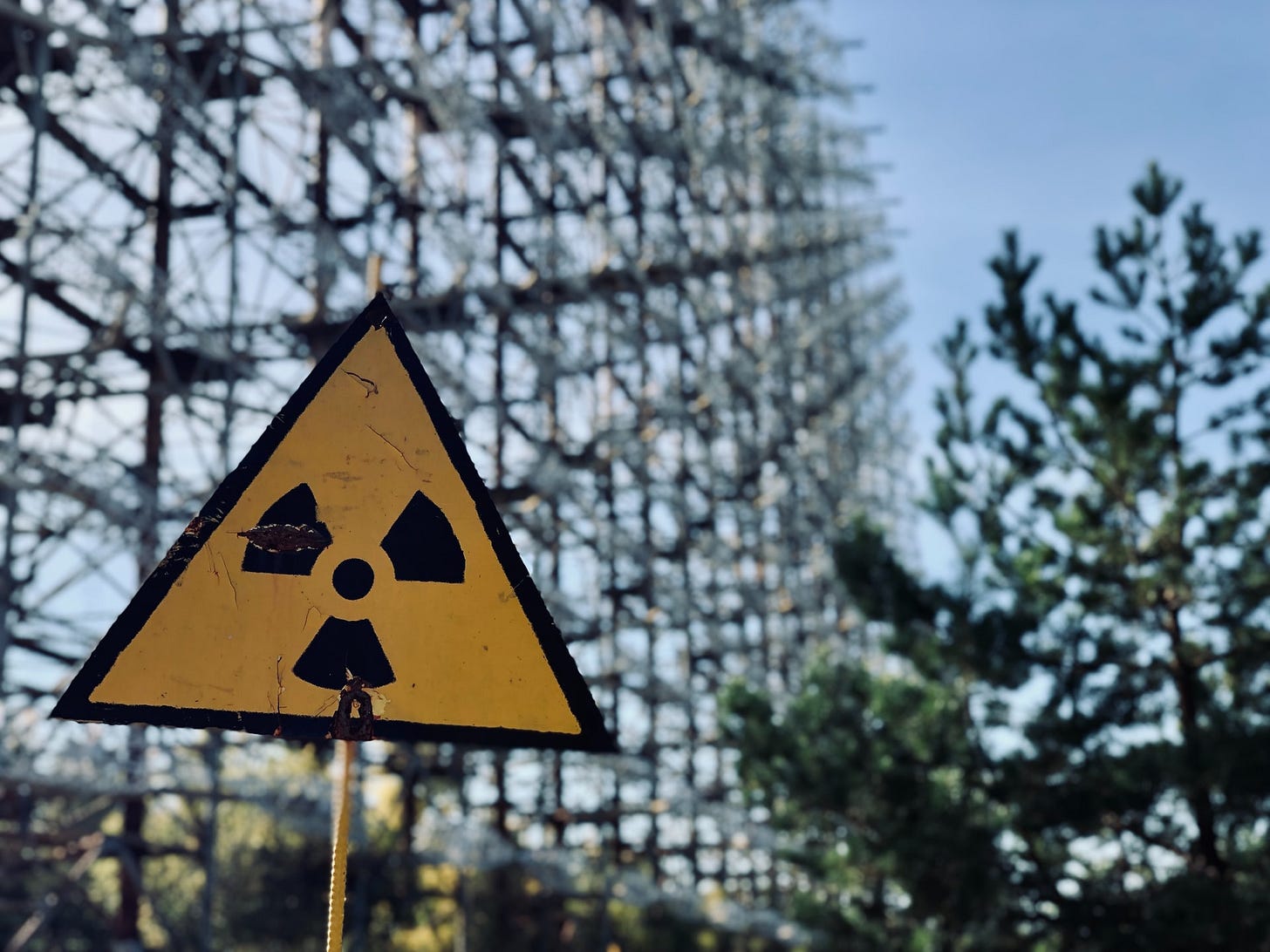
Photo by Vladyslav Cherkasenko on Unsplash
Radioactive data: tracing through training (Facebook AI Research)
In modern AI systems, we run into complex data and processing pipelines that have several stages and it becomes challenging to trace the provenance and transformations that have been applied to a particular data point. This research from the Facebook AI Research team proposes a new technique called radioactive data that borrows from medical science where compounds like BaSO4 are injected to get better results in CT scans. This technique applies minor, imperceptible perturbations to images in a dataset by causing shifts within the feature space making them “carriers”.
Different from other techniques that rely on poisoning the dataset that harms classifier accuracy, this technique instead is able to detect such changes even when the marking and classification architectures are different. It not only has potential to trace how data points are used in the AI pipeline but also has implications when trying to detect if someone claims not to be using certain images in their dataset but they actually are. The other benefit is that such marking of the images is difficult to undo thus adding resilience to manipulation and providing persistence.
Tech’s Shadow Workforce Sidelined, Leaving Social Media to the Machines (Bloomberg)
With a rising number of people relying on social media for the news, the potential for hateful content and misinformation spreading has never been higher. Content moderation on platforms like Facebook and YouTube is still largely a human endeavor where there are legions of contract workers that spend their days reviewing whether different pieces of content meet the community guidelines of the platform. Due to the spread of the pandemic and offices closing down, a lot of these workers have been asked to leave (they can’t do this work from home as the platform companies explained because of privacy and legal reasons), leaving the platforms in the hands of automated systems.
The efficacy of these systems has always been questionable and as some examples in the article point out, they’ve run amok taking down innocuous and harmful content alike, seeming to not have very fine-tuned abilities. The problem with this is that legitimate sources of information, especially on subjects like COVID-19, are being discouraged because of their content being taken down and having to go through laborious review processes to have their content be approved again. While this is the perfect opportunity to experiment with the potential of using automated systems for content moderation given the traumatic experience that humans have to undergo as a part of this job, the chasms that need to be bridged still remain large between what humans have to offer and what the machines are capable of doing at the moment.
Why countries need to work together on AI (World Economic Forum)
A lot of articles pitch development, investment and policymaking in AI as an arms race with the US and China as front-runners. While there are tremendous economic gains to be had in deploying and utilizing AI for various purposes, there remain concerns of how this can be used to benefit society more than just economically. A lot of AI strategies from different countries are thus focused on issues of inclusion, ethics and more that can drive better societal outcomes yet they differ widely in how they seek to achieve those goals. For example, AI has put forth a national AI strategy that is focused on economic growth and social inclusion dubbed #AIforAll while the strategy from China has been more focused on becoming a global dominant force in AI which is backed by state investments.
Some countries have instead chosen to focus on creating strong legal foundations for the ethical deployment of AI while others are more focused on data protection rights. Canada and France have entered into agreements to work together on AI policy which places talent, R&D and ethics at the center. The author of the article makes a case for how global coordination of AI strategies might lead to even higher gains but also recognizes that governments will be motivated to tailor their policies to best meet the requirements of their countries first and then align with others that might have similar goals.
Here’s What Happens When an Algorithm Determines Your Work Schedule (Vice)
Workplace time management and accounting are common practices but for those of us who work in places where schedules are determined by automated systems, they can have many negative consequences, a lot of which could be avoided if employers paid more attention to the needs of their employees. Clopening is the notion where an employee working at a retail location is asked to not only close the location at the end of the day but also arrive early the next day to open the location. This among other practices like breaks that are scheduled down to the minute and on-call scheduling (something that was only present in the realm of emergency services) wreak havoc on the physical and mental health of employees. In fact, employees surveyed have even expressed willingness to take pay cuts to have greater control over their schedules.
In some places with ad-hoc scheduling, employees are forced to be spontaneous with their home responsibilities like taking care of their children, errands, etc. While some employees try to swap shifts with each other, often even that becomes hard to do because others are also in similar situations. Some systems track customer demand and reduce pay for hours worked tied to that leading to added uncertainty even with their paychecks. During rush seasons, employees might be scheduled for back to back shifts ignoring their needs to be with families, something that a human manager could empathize with and accommodate for.
Companies supplying this kind of software hide behind the disclaimer that they don’t take responsibility for how their customers use these systems which are often black-box and inscrutable to human analysis. This is a worrying trend that hurts those who are marginalized and those who require support when juggling several jobs just to make ends meet. Relying on automation doesn’t absolve the employers of their responsibility towards their employees.
Adversarial Policies: Attacking Deep Reinforcement Learning (University of California, Berkeley)
Reinforcement systems are increasingly moving from applications to beating human performance in games to safety-critical applications like self-driving cars and automated trading. A lack of robustness in the systems can lead to catastrophic failures like the $460m lost by Knight Capital and the harms to pedestrian and driver safety in the case of autonomous vehicles. RL systems that perform well under normal conditions can be vulnerable to adversarial agents that can exploit the brittleness of the systems when it comes to natural shifts in distributions and more carefully crafted attacks.
In prior threat models, the assumptions for the adversary are that they can modify directly the inputs going into the RL agent but that is not very realistic. Instead, here the authors focus more on a shared environment through which the adversary creates indirect impact on the target RL agent leading to undesirable behavior. For agents that are trained through self-play (which is a rough approximation of Nash equilibrium), they are vulnerable to adversarial policies. As an example, masked victims are more robust to modifications in the natural observations by the adversary but that lowers the performance in the average case. Furthermore, what the researchers find is that there is a non-transitive behavior between self-play opponent, masked victim, adversarial opponent and normal victim in that cyclic order. Self-play being normally transitive in nature, especially when mimicking real-world scenarios is then no doubt vulnerable to these non-transitive styled attacks.
Thus, there is a need to move beyond self-play and apply iteratively adversarial training defense and population based training methods so that the target RL agent can become robust to a wider variety of scenarios.
Racial disparities in automated speech recognition (Proceedings of the National Academy of Sciences)
This recent work highlights how commercial speech recognition systems carry inherent bias because of a lack of representation from diverse demographics in the underlying training datasets. What the researchers found was that even for identical sentences spoken by different racial demographics, the systems had widely differing levels of performance. As an example, for black users, the error rates were much higher than those for white users which probably had something to do with the fact that there is specific vernacular language used by black people which wasn’t adequately represented in the training dataset for the commercial systems. This pattern has a tendency to be amplifying in nature, especially for systems that aren’t frozen and continue to learn with incoming data. A vicious cycle is born where because of poor performance from the system, black people will be disincentivized from using the system because it takes a greater amount of work to get the system to work for them thus lowering utility. As a consequence of lower use, the systems get fewer training samples from black people thus further aggravating the problem. This leads to amplified exclusionary behavior mirroring existing fractures along racial lines in society. As a starting point, collecting more representative training datasets will aid in mitigating at least some of the problems in these systems.
From the archives:
Here’s an article from our blogs that we think is worth another look:
3 activism lessons from Jane Goodall you can apply in AI Ethics
Jane Goodall, one of the world’s most influential and beloved advocates for nature conservation, delivered the 2019 Beatty Lecture at McGill University on Thursday, September 26. Dr. Goodall delivered her first Beatty Lecture in 1979, where she shared stories about her groundbreaking research on chimpanzee behaviour in Gombe, Tanzania. To celebrate the 65th anniversary of the Beatty Lecture, Dr. Goodall returned to McGill forty years later to talk about the critical need for environmental stewardship and the power each individual has to bring about change. She is the first repeat lecturer in the Beatty’s history.
Guest contributions:
We invite researchers and practitioners working in different domains studying the impacts of AI-enabled systems to share their work with the larger AI ethics community, here’s this week’s featured post:
AI and Marketing: Why We Need to Ask Ethical Questions by Volha Litvinets (Ph.D. student at Sorbonne University in Paris, philosopher & tech ethicist with a digital marketing background)
The approach to marketing itself needs to change. By working together, philosophers, technicians, data engineers, marketers and programmers are able to create values and develop industries while respecting the principle of sustainable development.The only way to escape the risk of human obsolescence is to act with responsibility and overcome our ignorance so as not to lose our humanity.
If you’re working on something interesting and would like to share that with our community, please email us at support@montrealethics.ai
Events:
As a part of our public competence building efforts, we host events frequently spanning different subjects as it relates to building responsible AI systems, you can see a complete list here: https://montrealethics.ai/meetup
Given the advice from various health agencies, we’re avoiding physical events to curb the spread of COVID-19. Stay tuned for updates!
From elsewhere on the web:
Things from our network and more that we found interesting and worth your time.
If you’re looking for ways to contribute to the ongoing fight against the pandemic and want to utilize AI to aid in some of the research work going on, take a look at the following call to action on Kaggle: (We also recommend reading our research summary on the work from the OpenMined team to build solutions that are ethical, safe and inclusive)
This dataset was created by the Allen Institute for AI in partnership with the Chan Zuckerberg Initiative, Georgetown University’s Center for Security and Emerging Technology, Microsoft Research, and the National Library of Medicine - National Institutes of Health, in coordination with The White House Office of Science and Technology Policy.
Signing off for this week, we look forward to it again in a week! If you enjoyed this and know someone else that can benefit from this newsletter, please share it with them!
If you have feedback for this newsletter or think there is an interesting piece of research, development or event that we missed, please feel free to email us at support@montrealethics.ai
If someone has forwarded this to you and you like what you read, you can subscribe to receive this weekly newsletter by clicking below