AI Ethics #7: AI alignment, Scotland's AI strategy, reproducible ML, online privacy, tailored intelligibility, MAIEI learning communities and more ...
Our seventh weekly edition covering research and news in the world of AI Ethics
Welcome to the seventh edition of our weekly newsletter that will help you navigate the fast changing world of AI Ethics! Every week we dive into research papers that caught our eye, sharing a summary of those with you and presenting our thoughts on how it links with other work in the research landscape. We also share brief thoughts on interesting articles and developments in the field. More about us on: https://montrealethics.ai/about/
If someone has forwarded this to you and you want to get one delivered to you every week, you can subscribe to receive this newsletter by clicking below
We’re excited to bring back our public competence and consultation sessions - we are pooling our efforts (online for the first time) to contribute to Scotland’s AI strategy. You can find information about it at the end of the newsletter!
And, we are launching our learning communities which extend our efforts in building up public competence to a wider community. We operate on the open learning concept where we have a collaborative syllabus on each of the focus areas and meet every two weeks to learn from our peers. We are starting with 5 communities focused on: disinformation, privacy, labor impacts of AI, machine learning security and complex systems theory. You can fill out this form to receive an invite!
In research summaries this week, we dive into the idea of leveraging techniques from human-computer interactions to build better AI partners and intelligibility tailored for different stakeholders.
In article summaries, we talk about hacking autonomous vehicles, covering your tracks when going online, quantifying reproducibility in machine learning, deep learning used to shape Twitter timelines, aligning values in AI systems and how AI will create jobs.
Hoping you stay safe and healthy and looking forward to seeing you at our upcoming public consultation session and our learning communities! Enjoy this week’s content!
Research:
Let's look at some highlights of research papers that caught our attention at MAIEI:
Using Multimodal Sensing to Improve Awareness in Human-AI Interaction by Joshua Newn
With increasing capabilities of AI systems, and established research that demonstrates how human-machine combinations operate better than each in isolation, this paper presents a timely discussion on how we can craft better coordination between human and machine agents with the aim of arriving at the best possible understanding between them. This will enhance trust levels between the agents and it starts with having effective communication. The paper discusses how framing this from a human-computer interaction (HCI) approach will lead to achieving this goal. This is framed with intention-, context-, and cognition-awareness being the critical elements which would be responsible for the success of effective communication between human and machine agents.
To delve deeper, read our full summary here.
Different Intelligibility for Different Folks by Yishan Zhou and David Danks
Intelligibility is a notion that is worked on by a lot of people in the technical community who seek to shed a light on the inner workings of systems that are becoming more and more complex. Especially in the domains of medicine, warfare, credit allocation, judicial systems and other areas where they have the potential to impact human lives in significant ways, we seek to create explanations that might illuminate how the system works and address potential issues of bias and fairness.
However, there is a large problem in the current approach in the sense that there isn’t enough being done to meet the needs of a diverse set of stakeholders who require different kinds of intelligibility that is understandable to them and helps them meet their needs and goals. One might argue that a deeply technical explanation ought to suffice and others kinds of explanations might be derived from that but it makes them inaccessible to those who can’t parse well the technical details, often those who are the most impacted by such systems. The paper offers a framework to situate the different kinds of explanations such that they are able to meet the stakeholders where they are at and provide explanations that not only help them meet their needs but ultimately engender a higher level of trust from them by highlighting better both the capabilities and limitations of the systems.
To delve deeper, read our full summary here.
Articles:
Let’s look at highlights of some recent articles that we found interesting at MAIEI:
We hacked a Ford Focus and a Volkswagen Polo (Which)
Vehicle safety is something of paramount importance in the automotive industry as there are many tests conducted to test for crash resilience and other physical safety features before it is released to people. But, the same degree of scrutiny is not applied to the digital and connected components of cars. Researchers were able to demonstrate successful proof of concept hacks that compromised vehicle safety. For example, with the Polo, they were able to access the Controller Area Network (CAN) which sends signals and controls a variety of aspects related to driving functions. Given how the infotainment systems were updated, researchers were able to gain access into the personal details of the driver. They were also able to utilize the shortcomings in the operation of the key fob to gain access to the vehicle without leaving a physical trace.
Other hacks that were tried included being able to access and influence the collision monitoring radar system and the tire-pressure monitoring system which both have critical implications for passenger safety. On the Focus, they found WiFi details including the password for their production line in Detroit, Michigan.
On purchasing a second-hand infotainment unit for purposes of reverse-engineering the firmware, they found the previous owner’s home WiFi details, phone contacts and a host of other personal information.
Cars store a lot of personal information including tracking information which, as stated on the privacy policy, can be shared with affiliates which can have other negative consequences like changes in insurance premiums based on driving behaviour. Europe will have some forthcoming regulations for connected car safety but those are currently slated for release in 2021.
How to Cover Your Tracks Every Time You Go Online (Wired)
An increasing number of tools and techniques are being used to track our behaviour online and while some may have potential benefits, for example, the use of contact tracing to potentially improve public health outcomes, if this is not done in a privacy-preserving manner, there can be severe implications for your data rights. We’ve covered some of that in a recent research summary on the work from the OpenMined team. But, barring special circumstances like the current pandemic, there are a variety of simple steps that you can take to protect your privacy online. These range from simple steps like using an incognito browser window which doesn’t store any local information about your browsing on your device to using things like VPNs which protect snooping of your browsing patterns even from your ISP. When it comes to using the incognito function of your browser, if you’re logged into a service online, there isn’t any protection though it does prevent storing cookies on your device. With VPNs, there is an implicit trust placed in the provider of that service to not store logs of your browsing activity. An even more secure option is to use a privacy-first browser like Tor which routes your traffic requests through multiple locations making tracking hard. There is also an OS built around this called TailsOS that offers tracking protection from the device perspective as well not leaving any trace on the host machine allowing you to boot up from a USB. The EFF also provides a list of tools that you can use to get a better grip on your privacy as you browse online.
Quantifying Independently Reproducible Machine Learning (The Gradient)
Reproducibility is of paramount importance to doing rigorous research and a plethora of fields have suffered from a crisis where scientific work hasn’t met muster in terms of reproducibility leading to wasted time and effort on the part of other researchers looking to build upon each other’s work. The article provides insights from the work of a researcher who attempted a meta-science approach to trying to figure out what constitutes good, reproducible research in the field of machine learning. There is a distinction made early on in terms of replicability which hinges on taking someone else’s code and running that on the shared data to see if you get the same results but as pointed out in the article, that suffers from issues of source and code bias which might be leveraging certain peculiarities in terms of configurations and more. The key tenets to reproducibility are being able to simply read a scientific paper and set up the same experiment, follow the steps prescribed and arrive at the same results. Arriving at the final step is dubbed as independent reproducibility. The distinction between replicability and reproducibility also speaks to the quality of the scientific paper in being able to effectively capture the essence of the contribution such that anyone else is able to do the same.
Some of the findings from this work include that having hyperparameters well specified in the paper and its ease of readability contributed to the reproducibility. More specification in terms of math might allude to more reproducibility but it was found to not necessarily be the case. Empirical papers were inclined to be more reproducible but could also create perverse incentives and side effects. Sharing code is not a panacea and requires other accompanying factors to make the work really reproducible. Cogent writing was found to be helpful along with code snippets that were either actual or pseudo code though step code that referred to other sections hampered reproducibility because of challenges in readability.
Simplified examples while appealing didn’t really aid in the process and spoke to the meta-science process calling for data-driven approaches to ascertaining what works and what doesn’t rather than relying on hunches. Also, posting revisions to papers and being reachable over email to answer questions helped the author in reproducing the research work. Finally, the author also pointed out that given this was a single initiative and was potentially biased in terms of their own experience, background and capabilities, they encourage others to tap into the data being made available but these guidelines provide good starting points for people to attempt to make their scientific work more rigorous and reproducible.
Using Deep Learning at Scale in Twitter’s Timeline (Twitter’s Blog)
Prior to relevance based timeline, the Twitter newsfeed was ordered in reverse chronological order but now it uses a deep learning model underneath to display the most relevant tweets that are personalized according to the user's interactions on the platform. With the increasing use of Twitter as a source of news for many people, it's a good idea for researchers to gain an understanding of the methodology that is used to determine the relevance of tweets, especially as one looks to curb the spread of disinformation online. The article provides some technical details in terms of the deep learning infrastructure and the choices made by the teams in deploying computationally heavy models which need to be balanced with the expediency of the refresh times for a good experience on the platform. But, what's interesting from an AI ethics perspective are the components that are used to arrive at the ranking which constantly evolves based on the user's interaction with different kinds of content.
The ranked timeline consists of a handful of the tweets that are the most relevant to the user followed by others in reverse chronological order. Additionally, based on the time since one's last visit on the platform, there might be an ICYMI (“in case you missed it”) section as well. The key factors in ranking the tweets are their recency, presence of media cards, total interactions, history of engagement with the creator of the tweet, the user's strength of connection with the creator and the user's usage pattern of Twitter. From these factors, one can deduce why filter bubbles and echo chambers form on the platform and where designers and technologists can intervene to make the platform a more holistic experience for users that doesn't create polarizing fractions which can promote the spread of disinformation.
Automation will take jobs but AI will create them (Wired)
While the dominant form of discussion around the impacts of automation have been that it will cause job losses, this work from Kevin Scott offers a different lens into how jobs might be created by AI in the Rust Belt in the US where automation and outsourcing have been gradually stripping away jobs. Examples abound of how entrepreneurs and small business owners with an innovative mindset have been able to leverage advances in AI, coupling them with human labor to repurpose their businesses from areas that are no longer feasible to being profitable.
Precision farming utilizes things like drones with computer vision capabilities to detect hotspots with pests, disease, etc. in the big farms that would otherwise require extensive manual labor which would limit the size of the farms. Self-driving tractors and other automated tools also augment human effort to scale operations. The farm owners though highlight the opaqueness and complexity of such systems which make them hard to debug and fix themselves which sometimes takes away from the gains.
On the other hand, in places like nursing homes that get reimbursed based on the resource utilization rates by their residents, tools using AI can help minimize human effort in compiling data and let them spend more of their effort on human contact which is not something that AI succeeds on yet. While automation has progressed rapidly, the gains haven't been distributed equally.
In other places where old factories were shut down, some of them are now being utilized by ingenious entrepreneurs to bring back manufacturing jobs that cleverly combine human labor with automation to deliver high-quality, custom products to large enterprises. Thus, there will be job losses from automation but the onus lies with us in steering the progress of AI towards economic and ethical values that we believe in, something we covered in last week's research summary.
Aligning AI to human values means picking the right metrics (Partnership on AI)
AI value alignment is typically mentioned in the context of long-term AGI systems but this also applies to the narrow AI systems that we have today. Optimizing for the wrong metric leads to things like unrealistic and penalizing work schedules, hacking attention on video platforms, charging more money from poorer people to boost the bottomline and other unintended consequences. Yet, there are attempts by product design and development teams to capture human well-being as metrics to optimize for. “How does someone feel about how their life is going?” is a pretty powerful question that gives a surprising amount of insight into well-being distanced from what might be influencing them at the moment because it makes them pause and reflect on what matters to them. But, capturing this subjective sentiment as a metric in an inherently quantitative world of algorithms is unsurprisingly littered with mines. A study conducted by Facebook and supported by external efforts found that passive use of social media triggered feelings of ennui and envy while active use including interactions with others on the network led to more positive feelings. Utilizing this as a guiding light, Facebook strove to make an update that would be more geared towards enabling meaningful engagement rather than simply measuring the number of likes, shares and comments. They used user panels as an input source to determine what constituted meaningful interactions on the platform and tried to distill this into the well-being metrics. Yet, this suffered from several flaws, namely that the evaluation of this change was not publicly available and was based on the prior work comparing passive vs. active use of social media.
This idea of well-being optimization extends to algorithmic systems beyond social media platforms, for example, with how gig work might be better distributed on a platform such that income fluctuations are minimized for workers who rely on it as a primary source of earnings. Another place could be amending product recommendations to also capture environmental impacts such that consumers can incorporate that into their purchasing decisions apart from just the best price deals that they can find.
Participatory design is going to be a key factor in the development of these metrics; especially given the philosophy of “nothing about us without us” as a north star to ensure that there isn’t an inherent bias in how well-being is optimized for. Often, we’ll find that proxies will need to stand in for actual well-being in which case it is important to ensure that the metrics are not static and are revised in consultation with users at periodic intervals. Tapping into the process of double loop learning, an organization can not only optimize for value towards its shareholders but also towards all its other stakeholders. While purely quantitative metrics have obvious limitations when trying to capture something that is inherently subjective and qualitative, we need to attempt something in order to start and iterate as we go along.
From the archives:
Here’s an article from our blogs that we think is worth another look:
How Kathleen Siminyu created Kenya’s go-to space for Women in Machine Learning
Kathleen Siminyu is a data scientist & machine learning engineer who is Regional Coordinator for the Artificial Intelligence for Development – Africa Network. She is the Co-Founder of the Nairobi Women in Machine Learning & Data Science community, and part of the Deep Learning Indaba Steering Committee. Her other interests include natural language processing for African languages and low-cost hardware robotics.
We share this story as a demonstration of how AI can indirectly bring people together and empower communities instead of downgrade, divide, or discriminate against them.We believe that community leaders have an important role to play in defining humanity’s place in a world of algorithms.
Guest contributions:
We invite researchers and practitioners working in different domains studying the impacts of AI-enabled systems to share their work with the larger AI ethics community, here’s this week’s featured post:
Why the contemporary view of the relationship between AI’s moral status and rights is wrong By Thomas O’Callaghan-Brown (Philosophy & Biology, McGill University)
Authors such as John P. Sullins and Colin Allen suggest that AI of all kinds have moral status. Following from this we can assume that they are owed moral rights. This paper argues that it is indeed true that AI deserve moral rights, but that they cannot be said to have moral status. Therefore, a change to the framework ought to be carried out. This change is necessary due to the confusion that arises out of labelling AI and other entities as moral agents, which can be avoided by arguing that no entity needs moral status to be treated morally. Following this, I will amalgamate the two views to show why AI, despite their non-moral status, ought to be owed moral rights. Finally, I suggest emendations to the contemporary framework and the consequences this has for other non-moral agents.
If you’re working on something interesting and would like to share that with our community, please email us at support@montrealethics.ai
Events:
As a part of our public competence building efforts, we host events frequently spanning different subjects as it relates to building responsible AI systems, you can see a complete list here: https://montrealethics.ai/meetup
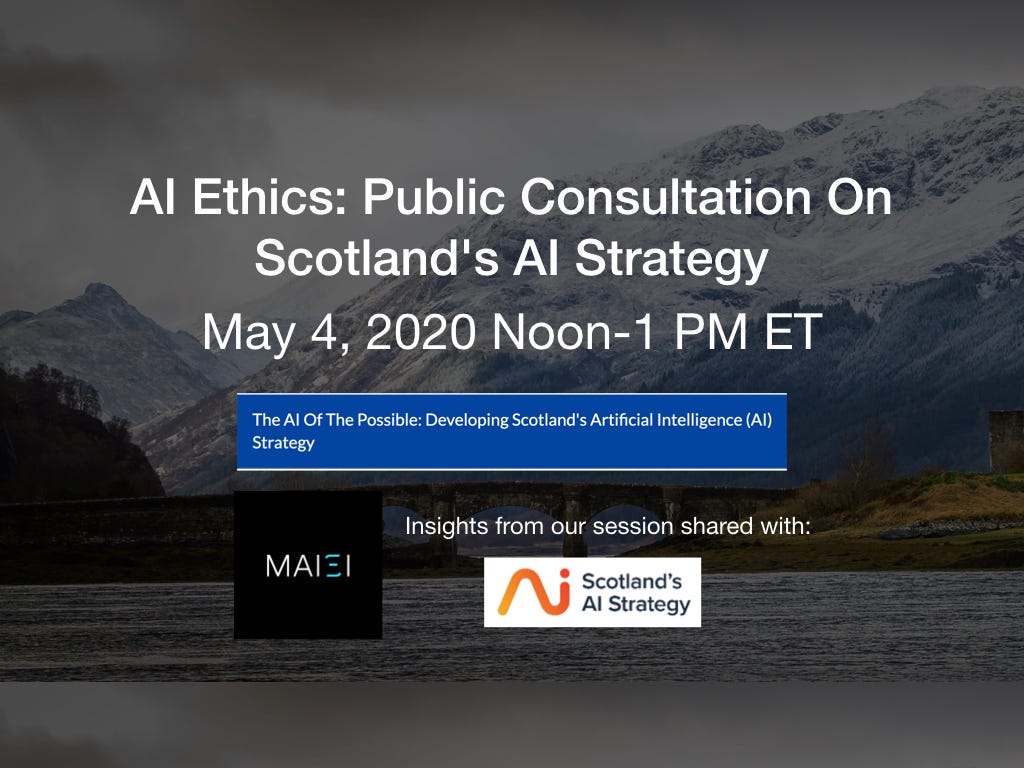
We are hosting our first online session to share our insights with the government of Scotland on their AI Strategy drawing from our diverse perspectives and experience and having done so before for various other public consultations. Given that this will be a shorter session and focused on providing concrete recommendations, we encourage you to read the document beforehand and frame your contributions in line with the questions. You can find all the details on the event page, please make sure to register as we have limited spots (because of the online hosting solution).
Please sign up via the link here!
From elsewhere on the web:
Things from our network and more that we found interesting and worth your time.
An interesting piece of work called DP3T on contact tracing technology that offers a privacy-preserving solution in a decentralized manner:
The repository contains a proposal for a secure and decentralized privacy-preserving proximity tracing system. Its goal is to simplify and accelerate the process of identifying people who have been in contact with an infected person, thus providing a technological foundation to help slow the spread of the SARS-CoV-2 virus. The system aims to minimize privacy and security risks for individuals and communities and guarantee the highest level of data protection.
For more information, take a look here.
Signing off for this week, we look forward to it again in a week! If you enjoyed this and know someone else that can benefit from this newsletter, please share it with them!
If you have feedback for this newsletter or think there is an interesting piece of research, development or event that we missed, please feel free to email us at support@montrealethics.ai
If someone has forwarded this to you and you like what you read, you can subscribe to receive this weekly newsletter by clicking below