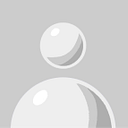

Discover more from The AI Ethics Brief
AI Ethics Brief #152: Goodbye Goodhart, zombie policies, FeedbackLogs, Pope@G7 on AI ++
What structural and process flaws exist within an organization that allow zombie policies to persist?
Welcome to another edition of the Montreal AI Ethics Institute’s weekly AI Ethics Brief that will help you keep up with the fast-changing world of AI Ethics! Every week, we summarize the best of AI Ethics research and reporting, along with some commentary. More about us at montrealethics.ai/about.
Support our work through Substack
💖 To keep our content free for everyone, we ask those who can, to support us: become a paying subscriber for the price of a couple of ☕.
If you’d prefer to make a one-time donation, visit our donation page. We use this Wikipedia-style tipping model to support our mission of Democratizing AI Ethics Literacy and to ensure we can continue to serve our community.
This week’s overview:
🚨 Quick take on recent news in Responsible AI:
Ineffective guardrails in image and video generation
🙋 Ask an AI Ethicist:
Goodbye Goodhart: Escaping metrics and policy gaming in Responsible AI
✍️ What we’re thinking:
Three Strategies for Responsible AI Practitioners to Avoid Zombie Policies
🤔 One question we’re pondering:
What structural and process flaws exist within an organization that allow zombie policies to persist?
🪛 AI Ethics Praxis: From Rhetoric to Reality
Hallucinating and moving fast
🔬 Research summaries:
Demystifying Local and Global Fairness Trade-offs in Federated Learning Using Partial Information Decomposition
FeedbackLogs: Recording and Incorporating Stakeholder Feedback into Machine Learning Pipelines
The path toward equal performance in medical machine learning
📰 Article summaries:
How to Lead an Army of Digital Sleuths in the Age of AI | WIRED
This Is What It Looks Like When AI Eats the World - The Atlantic
Why the Pope has the ears of G7 leaders on the ethics of AI
📖 Living Dictionary:
What is the relevance to AI ethics of hallucinations in LLMs?
🌐 From elsewhere on the web:
MAIEI co-hosted an evening on Responsible AI at Collision Conf 2024 with IBM and Meta!
💡 ICYMI
Adding Structure to AI Harm
🚨 Ineffective guardrails in image and video generation - here’s our quick take on what happened recently.
With the recent release of the Luma Dream Machine and its video generation capabilities (something that OpenAI’s Sora had demonstrated several months ago), there was an immediate concern raised on how this might be used to generate photorealistic NSFW and non-consensual content. The team at Luma mentioned that they have guardrails in place to prevent that very kind of interaction. Yet, some creative or rather malicious users online tried to “jailbreak” the system and succeeded in generating some of that prohibited content.
Techniques such as word order alteration and code-switching between languages can fool the system into generating inappropriate content, such as nudity or unauthorized depictions of celebrities.
This vulnerability underscores a significant issue: the current safeguards are reactive rather than proactive. They are often designed to catch known threats, which means they can be easily circumvented by novel and creative methods. This is particularly concerning given the potential for these tools to be used in harmful ways, including:
Misinformation and Deepfakes: Generative AI can create convincing fake videos that can be used to spread misinformation, manipulate public opinion, and defame individuals.
Privacy Violations: The ability to generate realistic images and videos of individuals without their consent raises significant privacy concerns.
Psychological Impact: Exposure to AI-generated content that appears real can have psychological effects, from trust erosion to emotional distress.
Did we miss anything?
Sign up for the Responsible AI Bulletin, a bite-sized digest delivered via LinkedIn for those who are looking for research in Responsible AI that catches our attention at the Montreal AI Ethics Institute.
🙋 Ask an AI Ethicist:
Every week, we’ll feature a question from the MAIEI community and share our thinking here. We invite you to ask yours, and we’ll answer it in the upcoming editions.
Here are the results from the previous edition for this segment:
The results here are not that surprising given that most new programs, especially something as experimental as Responsible AI, will be rolled out in terms of small pilots to give staff enough time to familiarize themselves and offer those managing the program enough breathing room to course-correct as they learn what works and what doesn’t.
This past week, we had Samaira P. write to us and ask about what they’ve seen within their organization when it comes to people trying to “hack the system” to go about doing what they want despite policies and tracking in place, i.e., they follow the “law” in letter but not in spirit. This is a classic case of gaming the system, something we write about in Goodbye Goodhart: Escaping metrics and policy gaming in Responsible AI.
As Responsible AI (RAI) program implementations mature over time, they often introduce metrics to track how well the organization is doing in terms of its RAI posture. Additionally, to motivate adoption by staff, there are often incentives that are either introduced or altered within existing functions to ensure that there is alignment towards the program’s goals and progress is made steadily towards achieving those goals. Yet, there is a common pitfall that any such approach is susceptible to: Goodhart’s Law, i.e., “When a measure becomes a target, it ceases to be a good measure.”
What are some approaches that you’ve seen that work particularly well to combat the problems we discussed in this section? Please let us know! Share your thoughts with the MAIEI community:
✍️ What we’re thinking:
Three Strategies for Responsible AI Practitioners to Avoid Zombie Policies
In an age in which technology, societal norms, and regulations are evolving rapidly, outdated or ineffective governance policies, often called "zombie policies," risk plaguing Responsible AI program implementations in organizations. By understanding the characteristics and persistence of these policies, we can uncover why addressing them is crucial for fostering innovation, trust, and efficacy in AI deployment and governance.
As AI technologies become increasingly integrated into critical aspects of organizations, the persistence of outdated policies can lead to significant resource drains, hinder progress, and damage institutional credibility. In particular, it can dampen enthusiasm and investments from senior leadership in nascent programs, a death knell for Responsible AI, which requires tremendous support to overcome organizational inertia.
Additionally, these zombie policies not only stifle innovation but also perpetuate inefficiencies that can have far-reaching consequences by perpetuating and encouraging behaviors and ‘gaming-the-system’ that happens when staff follow the policies in letter but not in spirit. By adopting strategies for continuous policy review, evidence-based decision-making, and cultivating an agile organizational culture, Responsible AI practitioners can dismantle obsolete structures, paving the way for more effective, ethical, and responsive governance and policies.
To delve deeper, read the full article here.
🤔 One question we’re pondering:
Given the above article about zombie policies, we’ve been noticing them more and more, not just in AI governance, but in other cases as well, such as filing expense reports and other administrative policies. While we concern ourselves here specifically with things that affect AI, it might be worth examining what structural and process flaws exist within an organization that allow these zombie policies to persist.
We’d love to hear from you and share your thoughts with everyone in the next edition:
🪛 AI Ethics Praxis: From Rhetoric to Reality
As we now get Apple Intelligence (yes, the other AI :D) embedded into the (latest) Apple devices, we’re about to experience a very broad-based usage and exposure to Generative AI, even bringing it to a lot of folks who’ve so far not had any experience with it. This along with Microsoft Copilot+ PCs is really pushing the boundaries for how pervasive Generative AI is going to become. While a lot of it is going to be grounded in RAG-based approaches to minimize hallucinations, it is worthwhile talking about Hallucinating and moving fast as adoption and feature rollouts increase over the coming months.
WHAT’S HAPPENING: "Move fast and break things" is broken. But we've all said that many times before. Instead, I believe we need to adopt the "Move fast and fix things" approach. Given the rapid pace of innovation and its distributed nature across many diverse actors in the ecosystem building new capabilities, realistically, it is infeasible to hope to course-correct at the same pace. Because course correction is a much harder and slow-yielding activity, this ends up amplifying the magnitude of the impact of negative consequences.
FOG OF WAR: What we need to do instead is to think ahead of how the landscape of problems and solutions is going to evolve. For example, when thinking about the problem of hallucinations in GenAI systems, it is unclear at the moment where and how they will manifest. This hinders the adoption of GenAI-powered systems by companies that seek to offer safe and reliable outcomes to their customers, e.g., in customer-service chatbots in financial services or other high-stakes scenarios.
You can either click the “Leave a comment” button below or send us an email! We’ll feature the best response next week in this section.
🔬 Research summaries:
This research presents an information-theoretic formalization of group fairness trade-offs in federated learning (FL) where multiple clients come together to collectively train a model while keeping their own data private. A critical issue that arises is the disagreement between global fairness (overall disparity of the model across all clients) and local fairness (disparity of the model at each client), particularly when the data distributions across each client are different with respect to sensitive attributes such as gender, race, age, etc. Our work identifies three types of disparities—Unique, Redundant, and Masked—contributing to these disagreements. It presents theoretical and experimental insights into their interplay, answering pertinent questions like when global and local fairness agree and disagree.
To delve deeper, read the full summary here.
FeedbackLogs: Recording and Incorporating Stakeholder Feedback into Machine Learning Pipelines
Even though machine learning (ML) pipelines affect an increasing array of stakeholders, there is a growing need for documenting how input from stakeholders is recorded and incorporated. We propose FeedbackLogs, an addendum to existing documentation of ML pipelines, to track the feedback collection process from multiple stakeholders. Our online tool for creating FeedbackLogs and examples can be found here.
To delve deeper, read the full summary here.
The path toward equal performance in medical machine learning
Medical machine learning models are often better at predicting outcomes or diagnosing diseases in some patient groups than others. This paper asks why such performance differences occur and what it would take to build models that perform equally well for all patients.
To delve deeper, read the full summary here.
📰 Article summaries:
How to Lead an Army of Digital Sleuths in the Age of AI | WIRED
What happened: Bellingcat, a leading open-source intelligence agency, is directed by Eliot Higgins from the UK, overseeing around 40 employees. They utilize online forensic techniques to investigate events ranging from the 2014 downing of Malaysia Airlines Flight 17 to assassination attempts on Russian dissident Alexei Navalny. Bellingcat's work highlights the complexities of truth in the digital age, where factual information often loses value amidst competing narratives. Their mission includes not only uncovering the truth but also seeking platforms where truth holds significance, empowering the weak, and holding wrongdoers accountable.
Why it matters: Bellingcat's role has expanded with the increasing frequency of conflicts and the rise of falsified digital content. They are currently focusing on conflicts in Ukraine and Gaza and monitoring election-related misinformation globally. The advent of AI-generated content presents a significant challenge, as it can easily deceive the public and be used to dismiss genuine information. This scenario poses a threat to informed public discourse and democratic processes, as it can lead to widespread confusion and mistrust in verified facts.
Between the lines: Bellingcat's verification process involves rigorous analysis of various data points, including geo-location, shadows for time determination, and metadata. Despite their expertise, the proliferation of AI-generated imagery could eventually complicate their work. Coordinated disinformation campaigns using AI could create significant disruptions, such as influencing stock markets or misleading news organizations. To combat this, Higgins advocates for legislative measures requiring social media companies to implement AI detection systems, stressing that voluntary efforts are insufficient to prevent potential crises.
This Is What It Looks Like When AI Eats the World - The Atlantic
What happened: Recent weeks have highlighted the significant integration of AI into everyday online life. Major tech companies have introduced various AI-driven features: Meta added an AI chatbot to Instagram and Facebook, OpenAI released GPT-4o, and Google experimented with "AI Overviews" in its search engine. Additionally, the reported record earnings propelled some companies into a market capitalization exceeding $3 trillion. The tech industry's rapid AI advancements, coupled with strategic media partnerships, underscore the escalating presence of AI in numerous domains, often leaving users with little choice but to adapt.
Why it matters: These developments illustrate a power dynamic where tech companies dominate by utilizing media content without explicit permission, leveraging it to train their AI models. Media companies are often left with limited choices: accept financial partnerships with AI companies or risk having their data used without compensation. Legal battles, such as those initiated by The New York Times against OpenAI and Microsoft, are costly and uncertain, making it challenging for smaller organizations to pursue this route. The pervasive use of AI, coupled with minimal oversight, threatens to destabilize traditional business models for media and creative industries, creating an environment of ambiguity and inconsistency.
Between the lines: Despite the challenges, there is a potential opportunity for media organizations to negotiate better terms with tech companies, setting precedents for compensation for their content. Access to real-time news data is crucial for AI companies aiming to enhance their search tools, giving media organizations some leverage in these negotiations. However, the shift towards AI-driven information dissemination raises concerns about the future of creative work and the broader information ecosystem. The disruption caused by transitioning from traditional search engines to AI chatbots could undermine the sustainability of creative industries, as the foundational business models that support these sectors may become obsolete.
Why the Pope has the ears of G7 leaders on the ethics of AI
What happened: After discussing how to finance a prolonged war, G7 leaders in Puglia turned to Pope Francis for advice. Invited by Italian Prime Minister Giorgia Meloni, the Pope attended the summit. This marked the first time a religious leader participated in such an event, where the Pope offered his insights on the future, including a focus on AI. The Pope’s presence was a significant departure from the usual economic and political experts typically consulted by the G7.
Why it matters: The Pope's participation highlights the increasing significance of ethical and moral considerations in global governance, especially concerning AI. The G7 leaders are grappling with how to regulate AI, following Japan's Hiroshima Process International Guiding Principles, which, despite lacking legal standing, emphasize the need for ethical oversight. The EU and Canada are leading in regulation, while the UK and the US are less prescriptive. Pope Francis, influenced by Franciscan Friar Paolo Benanti, underscores the anthropological and societal challenges posed by AI, stressing the importance of maintaining human decision-making authority.
Between the lines: AI's impact extends beyond replacing physical labor to potentially supplanting human intellect, raising ethical concerns about its unchecked development. Friar Benanti, a key advisor to Meloni and the Pope, emphasizes the concept of "algor-ethics," advocating for a balanced approach where technology enhances rather than replaces human capabilities. Meloni's alignment with the Pope's views on AI indicates a shift toward integrating ethical considerations into technological advancements. The Pope's engagement with contemporary issues like AI reflects his broader mission to address humanity's frontier challenges, making his guidance sought after by world leaders.
📖 From our Living Dictionary:
What is the relevance to AI ethics of hallucinations in LLMs?
👇 Learn more about why it matters in AI Ethics via our Living Dictionary.
🌐 From elsewhere on the web:
MAIEI co-hosted an evening on Responsible AI at Collision Conf 2024 with IBM and Meta!
Here’s what Deb Pimentel, President and GM Technology, IBM Canada, had to say about the event:
Just wrapped up an incredible evening to kick off Collision Conf this year with our AI Alliance counterparts including Meta and the Montreal AI Ethics Institute. We discussed the value of open source innovation, responsible AI adoption and the bright future for #GenerativeAI leadership in Canada. The energy in the room was electric, and I'm more confident than ever that Canada is poised well to take the lead in this space.
💡 In case you missed it:
As AI systems are deployed across all domains of daily life, harmful incidents involving the technology become more frequent. This paper presents a framework for defining, identifying, classifying, and understanding harms from AI systems to facilitate effective monitoring and risk mitigation. Understanding whom, how, and when AI systems cause harm is critical for AI’s responsible design and use.
To delve deeper, read the full article here.
Take Action:
We’d love to hear from you, our readers, on what recent research papers caught your attention. We’re looking for ones that have been published in journals or as a part of conference proceedings.