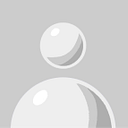

Discover more from The AI Ethics Brief
AI Ethics Brief #130: Cinderella and Soundarya, AI nudging on children, security flaws in GenAI, CV + sustainability, and more.
Whose AI dream? In search of aspiration in data annotation.
Welcome to another edition of the Montreal AI Ethics Institute’s weekly AI Ethics Brief that will help you keep up with the fast-changing world of AI Ethics! Every week, we summarize the best of AI Ethics research and reporting, along with some commentary. More about us at montrealethics.ai/about.
Support our work through Substack
💖 To keep our content free for everyone, we ask those who can, to support us: become a paying subscriber for the price of a couple of ☕.
If you’d prefer to make a one-time donation, visit our donation page. We use this Wikipedia-style tipping model to support our mission of Democratizing AI Ethics Literacy and to ensure we can continue to serve our community.
This week’s overview:
🙋 Ask an AI Ethicist:
What are some steps that we can take to make the current US policymaking approach more inclusive?
✍️ What we’re thinking:
Computer vision and sustainability
Regulating AI to ensure Fundamental Human Rights: reflections from the Grand Challenge EU AI Act
🤔 One question we’re pondering:
What is reasoning in LLMs?
🔬 Research summaries:
Cinderella’s shoe won’t fit Soundarya: An audit of facial processing tools on Indian faces
International Human Rights, Artificial Intelligence, and the Challenge for the Pondering State: Time to Regulate?
An Audit Framework for Adopting AI-Nudging on Children
📰 Article summaries:
What OpenAI Really Wants | WIRED
In Its First Monopoly Trial of Modern Internet Era, U.S. Sets Sights on Google - The New York Times
Generative AI’s Biggest Security Flaw Is Not Easy to Fix | WIRED
📖 Living Dictionary:
What is an example of a smart city initiative?
🌐 From elsewhere on the web:
The rapid rise of AI art
💡 ICYMI
Whose AI Dream? In search of the aspiration in data annotation.
🚨 The Responsible AI Bulletin
We’ve restarted our sister publication, The Responsible AI Bulletin, as a fast-digest every Sunday for those who want even more content beyond The AI Ethics Brief. (Our lovely power readers 🏋🏽, thank you for writing in and requesting it!)
The focus of the Bulletin is to give you a quick dose of the latest research papers that caught our attention in addition to the ones covered here.
🙋 Ask an AI Ethicist:
Every week, we’ll feature a question from the MAIEI community and share our thinking here. We invite you to ask yours, and we’ll answer it in the upcoming editions.
Here are the results from the previous edition for this segment:
So, we have a clear winner here! Our question would then be, what are some steps that we can take to make the current US policymaking approach more inclusive?
An important point for discussion here that we’ve also highlighted in previous editions, is one of the right model of stakeholder engagement. In particular, it is worth revisiting the Arnstein ladder of citizen participation, adapted for the era of AI:
It seems that at the moment, we’re in the first few rungs of the ladder, i.e., in the stage of non-participation, which is even worse than tokenism - something that most people are calling the current level of inclusive engagement (an overstatement upon examining this ladder).
One of the challenges posed in meaningful engagement of the broader public in AI-related policymaking is the complexity of these systems, in particular how wide-spanning their impacts can be, as highlighted in this article. Ultimately, it will take innovation on the front of stakeholder engagement models as well to move the policymaking needle towards more inclusive methods, especially those that meaningfully solicit feedback and inputs from those who might not otherwise have a voice in shaping upcoming legislations and regulations.
What might be ways that we can help boost US policymakers’ understanding of true inclusiveness in the process? Share your thoughts with the MAIEI community:
✍️ What we’re thinking:
Computer vision and sustainability
When thinking about the ethics of AI, one usually considers AI’s impact on individual freedom and autonomy or its impact on society and governance. These implications are important, of course, but AI’s impact on the environment is often overlooked. This fourth column on the ethics of computer vision, therefore, discusses computer vision in light of environmental sustainability.
To delve deeper, read the full article here.
Regulating AI to ensure Fundamental Human Rights: reflections from the Grand Challenge EU AI Act
In this column, the authors report on their experience as one of the teams participating in the innovative “Grand Challenge EU AI Act” event at the University of St. Gallen and provide subsequent reflections on AI applications and the perceived strengths and weaknesses of the Act.
To delve deeper, read the full article here.
🤔 One question we’re pondering:
Our staff this week engaged in thinking more deeply about “What is reasoning in LLMs?” inspired by a recent post by Melanie Mitchell from the Santa Fe Institute. In particular, we spent some time unpacking the following:
The word “reasoning” is an umbrella term that includes abilities for deduction, induction, abduction, analogy, common sense, and other “rational” or systematic methods for solving problems. Reasoning is often a process that involves composing multiple steps of inference. Reasoning is typically thought to require _abstraction_—that is, the capacity to reason is not limited to a particular example, but is more general.
-Melanie Mitchell
In the case of LLMs, ideas like chain-of-thought, tree-of-thought, and others are helping to shed some light on what might be going on when we believe that LLMs might be reasoning. But, ultimately, given the statistical next-token generation behavior of LLMs, it is still very difficult to argue that LLMs have some form of abstract reasoning. Especially when many counter-examples show how systems like ChatGPT fail at solving simple problems or they solve them but have the wrong background reasoning when asked how they arrived at the solution.
The authors of another paper testing LLM reasoning abilities put it this way: “Shortcut learning via pattern-matching may yield fast correct answers when similar compositional patterns are available during training but does not allow for robust generalization to uncommon or complex examples.”
We’d love to hear from you and share your thoughts with everyone in the next edition:
🔬 Research summaries:
Cinderella’s shoe won’t fit Soundarya: An audit of facial processing tools on Indian faces
The widespread adoption of facial processing systems poses several concerns. One of these concerns emanates from claims about the accuracy of these technologies without adequate and context-specific evaluation of what accuracy means or its sufficiency as a metric. Focusing on the Indian context, this paper takes a small step in that direction. It tests the face detection and facial analysis functions of four commercial facial processing tools on a dataset of Indian faces. The goal was to understand the facial processing outcomes for different demographic groups and the likely implications of those differences in the Indian social and legal context.
To delve deeper, read the full summary here.
In both regional and global International Human Rights law, it is firmly established that states have a dual obligation: states are not only to refrain from committing acts in violation of human rights, but they must also ensure that the ‘ …essential rights of the persons under their jurisdiction are not harmed.’ This paper argues that the lack of adequate regulation in the AI sector may violate International Human Rights norms, reflecting a state where governments have failed to uphold their obligations to protect, fulfill, and remedy.
To delve deeper, read the full summary here.
An Audit Framework for Adopting AI-Nudging on Children
This white paper looks at a specific type of AI technology: persuasive design empowered by AI, and we called it AI-nudging (others call it hyper-nudging). We offer a risk factor analysis of adopting this kind of tech in two specific contexts: social media and video games. Finally, we indicate some risk mitigation strategies to deal with the risks of AI nudging for children and teens.
To delve deeper, read the full summary here.
📰 Article summaries:
What OpenAI Really Wants | WIRED
What happened: In November, OpenAI unleashed ChatGPT, a revolutionary AI model, sparking a technological upheaval reminiscent of the internet's impact. OpenAI's mission, led by CEO Altman, extends beyond ChatGPT and GPT-4; they aim to create safe artificial general intelligence (AGI), a concept more associated with science fiction than reality. This endeavor faces the challenge of delivering groundbreaking advancements in every product cycle, satisfying investor demands, and staying competitive while maintaining a mission to “elevate humanity.”
Why it matters: Each iteration of the GPT model outperforms its predecessor due to its access to exponentially more data. OpenAI trained GPT-2 on the internet with 1.5 billion parameters, resulting in significant improvements. However, the company hesitated to release it due to concerns about misuse. GPT-3, with a staggering 175 billion parameters, highlighted OpenAI's transformation from a niche tech company to a global player, emphasizing the importance of AI's impact on society.
Between the lines: As AI's potential drawbacks, such as job displacement and misinformation, became a societal concern, OpenAI positioned itself at the forefront of the discussion. Their visibility made them a primary target for critics and regulators. Yet, as OpenAI expands into various commercial ventures, questions arise about their ability to stay focused on their core mission of mitigating AGI-related risks. Balancing product development, partnerships, and research while avoiding conflicts remains challenging. Altman is committed to maintaining OpenAI's status as a leading research lab, even if it means leveraging others' advancements.
In Its First Monopoly Trial of Modern Internet Era, U.S. Sets Sights on Google - The New York Times
What happened: The U.S. Justice Department has invested three years across two presidential administrations to build a case against Google, alleging illegal abuse of power in online search to stifle competition. A trial in the U.S. District Court for the District of Columbia is commencing, questioning whether today's tech giants achieved dominance by breaking the law. This marks the first federal monopoly trial of the modern internet era, as tech companies like Google, Apple, Amazon, and Meta have acquired significant influence in various aspects of society.
Why it matters: This trial carries significant implications, especially for Google, a company that has grown into a $1.7 trillion behemoth by becoming the go-to platform for online searches. The government seeks to compel Google to change its monopolistic practices, potentially impose penalties, and restructure the company. The case focuses on whether Google illegally solidified its dominance by paying companies like Apple to set its search engine as the default on devices, making it harder for consumers to choose alternatives. Google contends that these deals were not exclusive, allowing consumers to change search engine preferences.
Between the lines: The Google trial has historical parallels to the 1998 case against Microsoft for antitrust violations. Some argue that this lawsuit made Microsoft more cautious, giving rise to competitors like Google. However, differing opinions exist on whether the Microsoft case effectively increased competition. Ultimately, the Google trial will test the applicability of antitrust laws from 1890, designed to break up monopolies in older industries, to the modern tech economy. It is a pivotal moment to evaluate the government's antitrust agenda concerning numerous big tech companies and their monopolistic practices.
Generative AI’s Biggest Security Flaw Is Not Easy to Fix | WIRED
What happened: Security researchers have found vulnerabilities in large language models (LLMs) like OpenAI's ChatGPT and Google's Bard. These vulnerabilities, known as "indirect prompt injection" attacks, allow hackers to manipulate AI systems by concealing instructions in web pages or documents, causing the AI to behave in unintended ways. As LLMs are increasingly used by large corporations and startups, the cybersecurity industry is concerned about the potential risks associated with these attacks. While there is no one-size-fits-all solution, cybersecurity experts emphasize the importance of implementing common security practices to mitigate these risks.
Why it matters: Indirect prompt injection attacks are particularly worrisome in the realm of LLMs. Unlike direct prompt injections, where users intentionally make the AI generate harmful content, indirect attacks involve third-party instructions hidden in sources like websites or PDFs. This poses a significant risk because whoever inputs data into the LLM gains considerable control over its output. Protecting against these attacks is crucial for safeguarding personal and corporate data. While companies like Google and Nvidia are developing security measures, there's no foolproof method, making it essential for developers to follow security best practices when integrating LLMs into their systems.
Between the lines: While AI systems can create security challenges, they also offer potential solutions. Google and Nvidia are implementing specially trained models and open-source guardrails to identify and prevent malicious inputs and unsafe outputs. However, these approaches have limitations, as it's impossible to predict all the ways malicious prompts may be used. Companies should adhere to security industry best practices when integrating LLMs into their systems to minimize the risks of indirect prompt injections. This includes considering the source and design of plug-ins and treating LLMs as untrusted entities when taking input from third parties, thereby establishing trust boundaries to reduce risks.
📖 From our Living Dictionary:
What is an example of a smart city initiative?
👇 Learn more about why it matters in AI Ethics via our Living Dictionary.
🌐 From elsewhere on the web:
Abhishek Gupta, founder and principal researcher at the Montreal AI Ethics Institute, says: “There is an obvious implication for the livelihood of artists, in particular those who rely heavily on funding their creative pursuits through commissioned art like book covers, illustrations, and graphic design. An erosion of avenues to commercial gain for their hard work is sure to have the twin effect of depressing existing artists’ financial means and discouraging newer artists who want to pursue the field as a full-time career.”
To delve deeper, read the full article here.
💡 In case you missed it:
Whose AI Dream? In search of the aspiration in data annotation.
This paper delves into the crucial role of annotators in developing AI systems, exploring their perspectives, aspirations, and ethical considerations surrounding their work. It offers valuable insights into the human element within AI and the impact annotators have on shaping the future of artificial intelligence.
To delve deeper, read the full article here.
Take Action:
We’d love to hear from you, our readers, on what recent research papers caught your attention. We’re looking for ones that have been published in journals or as a part of conference proceedings.
Reasoning is what we do when we lack complete knowledge. For example a chess master needs to reason because she can’t keep the entire decision tree in her head. Once you have complete knowledge you cease the need to reason. So reasoning is inherently a human trick for functioning with incomplete knowledge. I would go a step further and say the ability to reason and wonder and be enchanted with our world is uniquely a gift we have as humans.