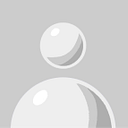

Discover more from The AI Ethics Brief
AI Ethics Brief #147: Pitfalls in RAI programs, responsible internal AI rollouts, ethics of audio models, watermarking in the sand, and more.
How can we build long-term accountability in the absence of truly independent observation tools for social media platforms?
Welcome to another edition of the Montreal AI Ethics Institute’s weekly AI Ethics Brief that will help you keep up with the fast-changing world of AI Ethics! Every week, we summarize the best of AI Ethics research and reporting, along with some commentary. More about us at montrealethics.ai/about.
Support our work through Substack
💖 To keep our content free for everyone, we ask those who can, to support us: become a paying subscriber for the price of a couple of ☕.
If you’d prefer to make a one-time donation, visit our donation page. We use this Wikipedia-style tipping model to support our mission of Democratizing AI Ethics Literacy and to ensure we can continue to serve our community.
This week’s overview:
🚨 Quick take on last week in Responsible AI:
Meta retiring CrowdTangle
🙋 Ask an AI Ethicist:
How to roll out an AI system for internal employee use even when there are known issues such as those of hallucinations and bias, amongst others?
✍️ What we’re thinking:
Report prepared by the Montreal AI Ethics Institute (MAIEI) on Publication Norms for Responsible AI
🤔 One question we’re pondering:
How can we build long-term accountability in the absence of truly independent observation tools for social media platforms?
🪛 AI Ethics Praxis: From Rhetoric to Reality
Common pitfalls in Responsible AI program implementations and what you can do about them
🔬 Research summaries:
The Ethical Implications of Generative Audio Models: A Systematic Literature Review
Right to be Forgotten in the Era of Large Language Models: Implications, Challenges, and Solutions
Never trust, always verify: a roadmap for Trustworthy AI?
📰 Article summaries:
Watermarking in the sand - Kempner Institute
Taylor Swift, the pope, Putin: in the age of AI and deepfakes, who do you trust?
How AI is transforming the business of advertising
📖 Living Dictionary:
What are the privacy implications of current AI companions?
🌐 From elsewhere on the web:
Your AI products’ values and behaviors could make or break your business. Here’s what you need to do to get them right
💡 ICYMI
Bound by the Bounty: Collaboratively Shaping Evaluation Processes for Queer AI Harms
🚨 Meta retiring CrowdTangle - here’s our quick take on what happened recently.
The removal of access to the CrowdTangle tool from Meta will have significant impacts on the responsible tech community, particularly in terms of transparency, accountability, and the ability to monitor misinformation effectively. CrowdTangle has been a crucial tool for researchers, journalists, and members of civil society to understand the spread of content on Meta’s platforms, including Facebook and Instagram. It allowed for real-time analysis of trends on these platforms, which was instrumental in identifying and tracking misinformation, as well as understanding the dynamics of content engagement and spread.
With CrowdTangle set to be discontinued on August 14, 2024, just weeks before the US presidential election, there are concerns about the preservation and expansion of visibility into Meta's platforms. Meta is encouraging researchers to use its Meta Content Library as an alternative. However, while the Content Library may improve on CrowdTangle in some aspects, such as including data about reach, it is much less broadly available than CrowdTangle was. This limitation is particularly concerning because, aside from certain fact-checkers, non-profit organizations, and academic institutions, others won't have direct access to the Content Library.
The responsible tech community is likely to face challenges in maintaining the same level of oversight and accountability without the broad access that CrowdTangle provided. CrowdTangle's ability to offer insights into the performance of social posts in real-time was unparalleled and its discontinuation could lead to a poorer public understanding of what happens on Meta’s platforms. This is especially problematic in the context of major elections, where the spread of misinformation can have significant implications.
Moreover, the decision to shut down CrowdTangle, despite its utility, reflects broader concerns about tech companies' commitment to transparency. The tool's discontinuation could be seen as a step back in efforts to ensure that social media platforms are accountable for the content they host and the algorithms that govern content distribution. The responsible tech community, including journalists and researchers, relied on CrowdTangle to hold Meta accountable for enforcing its policies and to track the spread of misinformation.
Did we miss anything?
Sign up for the Responsible AI Bulletin, a bite-sized digest delivered via LinkedIn for those who are looking for research in Responsible AI that catches our attention at the Montreal AI Ethics Institute.
🙋 Ask an AI Ethicist:
Every week, we’ll feature a question from the MAIEI community and share our thinking here. We invite you to ask yours, and we’ll answer it in the upcoming editions.
Here are the results from the previous edition for this segment:
Thinking about the responses above, given the highly competitive environment with funding constraints and intense wars for talent, it is unsurprising that we need an external mechanism, backed by the authority of the law, i.e., regulatory oversight, to maintain competitiveness in the AI ecosystem.
Over the last month, we’ve had a few questions come in asking about various facets of responsible AI, in particular, reader R.E. asks us how to roll out an AI system for internal employee use even when there are known issues such as those of hallucinations and bias, amongst others.
From our experience, the following 3 actions are a great starting point to adopt a balanced approach for an internal roll-out:
1. Establish Clear Guidelines and Training
Develop comprehensive guidelines that outline the AI system's intended use, its limitations, and the areas where it has known issues. Provide training sessions for all potential users, emphasizing critical thinking and judgment when interpreting AI-generated insights. Training should cover examples of biases and hallucinations the system might produce, preparing employees to recognize and respond to such incidents appropriately. This educative approach ensures users are not overly reliant on AI decisions and can use their discretion effectively, with a stark understanding that they are ultimately responsible for all the work outputs that they put their name on.
2. Foster an Ethical AI Culture
Promote a culture of ethical AI use within the organization. This involves creating an open environment where employees feel comfortable discussing the AI system’s shortcomings without fear of reprisal. Encourage departments to share their experiences and solutions in addressing biases and hallucinations, fostering a collaborative approach to problem-solving. Establish an ethics board or committee responsible for monitoring AI use and addressing any ethical issues that arise, ensuring accountability and continuous improvement in AI deployment. And perhaps most importantly, share both failures and successes when it comes to what is and isn’t working across the organization in this adoption process.
3. Implement Rigorous Testing and Validation Phases
Before full deployment, conduct extensive testing of the AI system under varied scenarios and datasets to identify and mitigate potential issues. This phase should involve not just the technical team but also end-users from different departments who can provide diverse perspectives on the AI's performance. Create a feedback loop where users can report inaccuracies, biases, or hallucinations. Use this data to refine the AI models continually. It’s crucial to treat this as an ongoing process rather than a one-time task to adapt to new challenges and data as they arise.
Ultimately, it's about creating a balanced approach where AI's potential is leveraged responsibly, with a constant eye toward improvement and ethical considerations, i.e., giving equal footing to ethical and business considerations in AI adoption within the organization.
Which of these techniques have been implemented at your organization when it has taken on using GenAI? Please let us know! Share your thoughts with the MAIEI community:
✍️ What we’re thinking:
Report prepared by the Montreal AI Ethics Institute (MAIEI) on Publication Norms for Responsible AI
The history of science and technology shows that seemingly innocuous developments in scientific theories and research have enabled real-world applications with significant negative consequences for humanity. In order to ensure that the science and technology of AI is developed in a humane manner, we must develop research publication norms that are informed by our growing understanding of AI's potential threats and use cases. Unfortunately, it's difficult to create a set of publication norms for responsible AI because the field of AI is currently fragmented in terms of how this technology is researched, developed, funded, etc. To examine this challenge and find solutions, the Montreal AI Ethics Institute (MAIEI) co-hosted two public consultations with the Partnership on AI in May 2020. These meetups examined potential publication norms for responsible AI, with the goal of creating a clear set of recommendations and ways forward for publishers.
In its submission, MAIEI provides six initial recommendations, these include: 1) create tools to navigate publication decisions, 2) offer a page number extension, 3) develop a network of peers, 4) require broad impact statements, 5) require the publication of expected results, and 6) revamp the peer-review process. After considering potential concerns regarding these recommendations, including constraining innovation and creating a "black market" for AI research, MAIEI outlines three ways forward for publishers, these include: 1) state clearly and consistently the need for established norms, 2) coordinate and build trust as a community, and 3) change the approach.
To delve deeper, read the full article here.
🤔 One question we’re pondering:
With the news about the retirement of the CrowdTangle tool from Meta, there has been a lot of consternation on the part of those who relied heavily on it to research and publish findings that helped inform the broader ecosystem how one of the largest social media platforms was influencing important facets of human lives. As is true with any proprietary tool that is built, gated, and maintained by the organization that it is supposed to provide insights into, long-term reliability is always doubtful. And this brings us to the question that we’ve been thinking about this week:
How can we build long-term accountability in the absence of truly independent observation tools for social media platforms?
We’d love to hear from you and share your thoughts with everyone in the next edition:
🪛 AI Ethics Praxis: From Rhetoric to Reality
Somewhat building on how to roll out AI systems internally within an organization when there are unresolved ethical issues, let’s think through some of the places where the program implementation can have some common pitfalls and what we can do about them:
Some of the things that we’ve found in our work that pose hindrances for effective program implementation include (1) lack of understanding and awareness of AI ethics, (2) inadequate policies and governance structures, (3) difficulty in measuring and monitoring compliance, and (4) resistance to change(!).
And the last one is a particularly dicey one, but here are a few steps that can help overcome these hurdles:
Facilitate Open Discussions and Feedback Loops: Begin by cultivating an environment where feedback on the AI ethics program is valued and used to make iterative improvements.
Implement AI Ethics Checkpoints: Integrate ethics checkpoints into project lifecycles to ensure continuous ethical considerations.
Develop Internal Reporting Tools and Metrics: Build tools and metrics for monitoring compliance and progress towards ethical AI goals, leveraging insights from the feedback loops and checkpoints.
Conduct Regular Training Sessions: Invest in comprehensive training programs to raise awareness and understanding of AI ethics across the organization, supported by the infrastructure and culture fostered by the first three steps.
While there are no silver bullets and no one-size-fits-all solutions to any of these issues, the steps outlined above work well generally and get you 90% of the way there in terms of addressing some of those pitfalls.
You can either click the “Leave a comment” button below or send us an email! We’ll feature the best response next week in this section.
🔬 Research summaries:
The Ethical Implications of Generative Audio Models: A Systematic Literature Review
This paper analyzes an exhaustive set of 884 papers in the generative audio domain to quantify how generative audio researchers discuss potential negative impacts of their work and catalog the types of impacts being considered. Jarringly, less than 10% of works discuss any potential negative impacts—particularly worrying because the papers that do so raise serious ethical implications and concerns relevant to the broader field, such as the potential for fraud, deep-fakes, and copyright infringement.
To delve deeper, read the full summary here.
Right to be Forgotten in the Era of Large Language Models: Implications, Challenges, and Solutions
The right to be forgotten (RTBF) is an integral aspect of the right to privacy and was established through a landmark case involving search engines. It is also a demonstration of the emergence of new rights as a result of technological advancements. This paper delves into the legal principles behind RTBF, highlighting that Large Language Models (LLMs), the emerging technology behind popular chatbots, are not exempt from this regulation, and in reality, their practical adherence to the law is highly challenging. The paper identified multiple issues of LLMs related to RTBF and offered potential directions for addressing the challenges.
To delve deeper, read the full summary here.
Never trust, always verify: a roadmap for Trustworthy AI?
Bringing AI systems into practice poses several trust issues, such as transparency, bias, security, privacy, safety, and sustainability. This paper examines trust in the context of AI-based systems, with the aim to understand how it is perceived around the world, and then suggest an end-to-end AI trust (resp. zero-trust) model to be applied throughout the AI project life-cycle.
To delve deeper, read the full summary here.
📰 Article summaries:
Watermarking in the sand - Kempner Institute
What happened: In a recent preprint, researchers investigated the feasibility of watermarking schemes for AI generative models, particularly focusing on text generation. Watermarking, advertised as a crucial safety measure for combating AI-enabled fraud, lacks clear definitions and proven methods for tamper resistance. The study by Hanlin Zhang and colleagues revealed significant challenges in achieving strong and robust watermarking under natural assumptions. Despite efforts to implement watermarking schemes, the research demonstrated their vulnerability to attacks, posing concerns about their effectiveness and reliability.
Why it matters: The paper presents a generic attack on watermarking schemes, challenging their viability in safeguarding against deceptive AI outputs. Assumptions about verification being easier than generation and the richness of high-quality output spaces underscore the inherent difficulties in implementing effective watermarking. The asymmetry favoring attackers suggests a continual struggle in enhancing watermarking schemes against evolving AI capabilities. These findings are crucial for policymakers and organizations to set realistic expectations and develop effective strategies to mitigate misuse of generative models.
Between the lines: The study highlights fundamental weaknesses in watermarking paradigms, emphasizing the need for nuanced approaches to AI safety. As attacks evolve alongside AI advancements, current watermarking schemes may struggle to withstand determined adversaries. Future regulations should acknowledge these limitations and focus on comprehensive strategies for model governance. While the research exposes vulnerabilities, it intends to inform discussions and guide the development of more resilient AI governance frameworks.
Taylor Swift, the pope, Putin: in the age of AI and deepfakes, who do you trust?
What happened: The landscape of information dissemination has evolved drastically with social media, enabling the rapid spread of propaganda and misinformation. Fake news, fueled by advancements in AI, presents a significant challenge, with deepfake technology blurring the lines between reality and fabrication. Additionally, the rise of "newsfluencers" on platforms like TikTok and YouTube further fragments shared realities, exacerbating the spread of disinformation.
Why it matters: These developments seriously threaten democracy, echoing historical instances where rumors and gossip influenced political outcomes. Authoritarian regimes actively exploit information warfare to sow distrust and undermine democratic institutions. The proliferation of false equivalencies and the erosion of truth jeopardize citizens' ability to discern reality, fostering a fertile ground for authoritarianism.
Between the lines: Navigating this complex terrain requires multifaceted approaches. While some advocate for increased transparency and media trust initiatives, others propose “counterfluencer” strategies to combat disinformation directly. However, leaving social media entirely may not be a viable solution, as it abdicates the responsibility to combat falsehoods. Addressing these challenges demands a concerted effort to cultivate resilience against misinformation and uphold the integrity of democratic principles.
How AI is transforming the business of advertising
What happened: At the beginning of the year, Publicis, a major advertising firm, sent personalized video messages to all its employees, which was made possible through artificial intelligence (AI). This approach highlighted the potential for hyper-personalized advertising campaigns enabled by evolving AI technology. Other advertising giants like WPP invest heavily in integrating AI into their operations, recognizing its transformative impact on creative work and customer targeting.
Why it matters: While AI promises innovation and efficiency in advertising, it also raises concerns about job displacement and the disruption of traditional agency models. The rise of AI-driven advertising comes amidst a decline in traditional media platforms, with tech giants like Google and Meta dominating the digital advertising landscape. This shift challenges advertising agencies to adapt quickly to digitalization and automation or risk being left behind.
Between the lines: Traditional advertisers face challenges from tech platforms and potential regulatory pressures, particularly concerning antitrust issues. Despite the growth of digital advertising, traditional forms like TV ads still hold significant cultural and economic sway, as seen in events like the Super Bowl. While AI may lead to job losses, it creates new opportunities in data science and analytics. However, there's a concern that the industry's focus on targeting and cost may overshadow traditional marketing values. The future of advertising agencies hinges on their ability to navigate these technological and cultural shifts.
📖 From our Living Dictionary:
What are the privacy implications of current AI companions?
👇 Learn more about why it matters in AI Ethics via our Living Dictionary.
🌐 From elsewhere on the web:
In the short time since artificial intelligence hit the mainstream, its power to do the previously unimaginable is already clear. But along with that staggering potential comes the possibility of AIs being unpredictable, offensive, even dangerous. That possibility prompted Google CEO Sundar Pichai to tell employees that developing AI responsibly was a top company priority in 2024. Already we’ve seen tech giants like Meta, Apple, and Microsoft sign on to a U.S. government-led effort to advance responsible AI practices. The U.K. is also investing in creating tools to regulate AI—and so are many others, from the European Union to the World Health Organization and beyond.
This increased focus on the unique power of AI to behave in unexpected ways is already impacting how AI products are perceived, marketed, and adopted. No longer are firms touting their products using solely traditional measures of business success—like speed, scalability, and accuracy. They’re increasingly speaking about their products in terms of their behavior, which ultimately reflects their values. A selling point for products ranging from self-driving cars to smart home appliances is now how well they embody specific values, such as safety, dignity, fairness, harmlessness, and helpfulness.
In fact, as AI becomes embedded across more aspects of daily life, the values upon which its decisions and behaviors are based emerge as critical product features. As a result, ensuring that AI outcomes at all stages of use reflect certain values is not a cosmetic concern for companies: Value-alignment driving the behavior of AI products will significantly impact market acceptance, eventually market share, and ultimately company survival. Instilling the right values and exhibiting the right behaviors will increasingly become a source of differentiation and competitive advantage.
But how do companies go about updating their AI development to make sure their products and services behave as their creators intend them to? To help meet this challenge we have divided the most important transformation challenges into four categories, building on our recent work in Harvard Business Review. We also provide an overview of the frameworks, practices, and tools that executives can draw on to answer the question: How do you get your AI values right?
To delve deeper, read more details here.
💡 In case you missed it:
Bound by the Bounty: Collaboratively Shaping Evaluation Processes for Queer AI Harms
AI systems are increasingly being deployed in various contexts; however, they perpetuate biases and harm marginalized communities. An important mechanism for auditing AI is allowing users to provide feedback on systems to the system designers; an example of this is through “bias bounties,” that reward users for finding and documenting ways that systems may be perpetuating harmful biases. In this work, we organized a participatory workshop to investigate how bias bounties can be designed with intersectional queer experiences in mind; we found that participants’ critiques went far beyond how bias bounties evaluate queer harms, questioning their ownership, incentives, and efficacy.
To delve deeper, read the full article here.
Take Action:
We’d love to hear from you, our readers, on what recent research papers caught your attention. We’re looking for ones that have been published in journals or as a part of conference proceedings.
The development and deployment of AI are happening at a global scale. How can we foster international collaboration and establish robust ethical frameworks for governance of AI across different countries and cultures? What challenges do you foresee in achieving this, and what potential solutions can we work towards?
How could someone named Alexa or Siri get a company to take action on changing the name of a voice assistant?