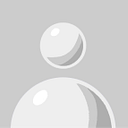

Discover more from The AI Ethics Brief
AI Ethics Brief #150: Secret cyborgs and their AI shadows, prompt middleware, dual governance, Chinese AI regulations ++
What are some odd (and unexpected!) places you’ve seen AI starting to be used?
Welcome to another edition of the Montreal AI Ethics Institute’s weekly AI Ethics Brief that will help you keep up with the fast-changing world of AI Ethics! Every week, we summarize the best of AI Ethics research and reporting, along with some commentary. More about us at montrealethics.ai/about.
Support our work through Substack
💖 To keep our content free for everyone, we ask those who can, to support us: become a paying subscriber for the price of a couple of ☕.
If you’d prefer to make a one-time donation, visit our donation page. We use this Wikipedia-style tipping model to support our mission of Democratizing AI Ethics Literacy and to ensure we can continue to serve our community.
This week’s overview:
🚨 Quick take on recent news in Responsible AI:
GenAI services down!
🙋 Ask an AI Ethicist:
Think further into the future: An approach to better RAI programs
✍️ What we’re thinking:
Secret Cyborgs and Their AI Shadows: Navigating the Copilot+ PCs Frontier
🤔 One question we’re pondering:
What are some odd (and unexpected!) places you’ve seen AI starting to be used?
🪛 AI Ethics Praxis: From Rhetoric to Reality
Some ideas on how to build an AI ethics team
🔬 Research summaries:
Prompt Middleware: Helping Non-Experts Engage with Generative AI
Dual Governance: The intersection of centralized regulation and crowdsourced safety mechanisms for Generative AI
The Unequal Opportunities of Large Language Models: Revealing Demographic Bias through Job Recommendations
📰 Article summaries:
The AI Revolution Is Crushing Thousands of Languages | The Atlantic
Why the Chinese government is sparing AI from harsh regulations—for now | MIT Technology Review
Speed of AI development stretches risk assessments to breaking point | Financial Times
📖 Living Dictionary:
How dynamic pricing hinted at algorithmic pricing
🌐 From elsewhere on the web:
Making Responsible AI the Norm rather than the Exception
💡 ICYMI
Fair allocation of exposure in recommender systems
🚨 GenAI services down! - here’s our quick take on what happened recently.
Bots down: Several major AI services experienced significant outages and disruptions on June 4, 2024. OpenAI's ChatGPT chatbot went down for many users worldwide on June 4, with the first reports of issues surfacing around 2:30am ET. In addition to ChatGPT, several other prominent AI services like Claude, Gemini, Perplexity, and Copilot also experienced downtime and disruptions on June 4.
Speculation galore: This led to speculation about a potential widespread infrastructure issue or internet-scale disturbance affecting multiple AI providers simultaneously. Some theorized that the surge of traffic to alternative AI services in the wake of ChatGPT's outage may have overloaded their systems as well.
Why it matters: The widespread AI service disruptions highlighted the growing reliance on these tools and the significant impact outages can have on businesses and users who depend on them daily. AI service providers need to invest in robust infrastructure, redundancy, failover mechanisms, and improved scalability to handle usage spikes and prevent future outages. The incidents also sparked discussions about the risks of centralized AI systems and the importance of transparency and accountability from providers when failures occur.
Did we miss anything?
Sign up for the Responsible AI Bulletin, a bite-sized digest delivered via LinkedIn for those who are looking for research in Responsible AI that catches our attention at the Montreal AI Ethics Institute.
🙋 Ask an AI Ethicist:
Every week, we’ll feature a question from the MAIEI community and share our thinking here. We invite you to ask yours, and we’ll answer it in the upcoming editions.
Here are the results from the previous edition for this segment:
It is interesting to see here that there is an almost equal balance between those who think that AI ethics processes are too complex vs. those who don’t. It would be enlightening to analyze further what are the factors that separate the two groups. Hopefully, those who answered that they found the processes to be too complex can read and benefit from last week’s article on Keep It Simple, Keep It Right: A Maxim for Responsible AI.
This week Maira G. wrote in to ask us about how to improve the Responsible AI program that they have at their organization, in particular, they found that they were having to undergo quite a few revisions to the setups that they had created in terms of policies and processes due to new releases and capabilities in AI models.
In this week’s article, we try and answer that question for Maira by looking at a framework that we use in our advisory work grounded in (1) proactive risk assessment, (2) scenario planning, and (3) ethical foresight. By extrapolating further than conventional methods and anticipating potential scenarios that might initially seem improbable, organizations can build AI systems that are responsible today and resilient to future challenges. The goal is to create AI governance structures that are adaptable, inclusive, and prepared for a wide range of outcomes. The key takeaways are:
Anticipate Future Challenges: By looking beyond the obvious, you prepare for a wider range of outcomes.
Integrate Diverse Perspectives: Combining insights from various fields helps in understanding complex trends.
Adapt and Evolve: Flexible policies and continuous learning ensure the Responsible AI program remains relevant and effective.
Are there any specific approaches that you’ve found useful in making Responsible AI programs more resilient to changing AI capabilities? Please let us know! Share your thoughts with the MAIEI community:
✍️ What we’re thinking:
Secret Cyborgs and Their AI Shadows: Navigating the Copilot+ PCs Frontier
The introduction of Copilot+ PCs marks a significant shift in the landscape of personal computing and organizational AI usage. While these devices offer tremendous potential for enhancing productivity and enabling advanced AI applications, they also present novel challenges like Shadow AI and secret cyborgs. As AI capabilities become more deeply embedded into everyday tools, organizations must proactively adapt their governance frameworks to ensure responsible and transparent usage.
Ultimately, the rise of Shadow AI and secret cyborgs underscores the need for a proactive and adaptive approach to AI governance. By embracing this challenge head-on, organizations can position themselves to thrive in an increasingly AI-driven world, ensuring that the power of AI is wielded responsibly and in alignment with their core values and objectives.
To delve deeper, read the full article here.
🤔 One question we’re pondering:
Given the above article about secret cyborgs and their AI shadows, what are some odd (and unexpected!) places you’ve seen AI starting to be used given that it is now so democratized in terms of access because of applications like ChatGPT?
We’d love to hear from you and share your thoughts with everyone in the next edition:
🪛 AI Ethics Praxis: From Rhetoric to Reality
So you're working on AI systems and are interested in Responsible AI? Have you run into challenges in making this a reality? Many articles mention a transition from principles to practice but end up falling flat when you try to implement them in practice. So what's missing? Here are some ideas on building an AI ethics team that I think will help you take the first step in making it a reality.
Get leadership buy-in
Set up feedback mechanisms
Empower people to make decisions
Align with organizational values
Make Responsible AI the norm rather than the exception
You can either click the “Leave a comment” button below or send us an email! We’ll feature the best response next week in this section.
🔬 Research summaries:
Prompt Middleware: Helping Non-Experts Engage with Generative AI
Generative AI models, like ChatGPT, have facilitated widespread access to advanced AI assistants for individuals lacking AI expertise. Yet, a persistent challenge remains: the absence of essential domain knowledge required to effectively instruct these agents and to skillfully elicit good responses through the “art” of prompt engineering. This work explores the question of how interfaces can best convey expert practices to users when using generative AI models.
To delve deeper, read the full summary here.
The increasing prominence of Generative Artificial Intelligence is evidenced by its use in consumer-facing, multi-use text and image-generating models. However, this is accompanied by a wide range of ethical and safety concerns, including privacy violations, misinformation, intellectual property theft, and the potential to impact livelihoods. To mitigate these risks, there is a need not just for policies and regulations at a centralized level but also for crowdsourced safety tools. In this work, we propose Dual Governance, a framework that combines centralized regulations in the U.S. and safety mechanisms developed by the community to tackle the harms of generative AI. We posit that implementing this framework promotes innovation and creativity while ensuring generative AI’s safe and ethical deployment.
To delve deeper, read the full summary here.
Large language models (LLMs) fuel transformative changes across multiple sectors by democratizing AI-powered capabilities; however, a critical concern is the impact of internal biases on downstream performance. Our paper examines biases within ChatGPT and LLaMA in the context of job recommendations, identifying clear biases, such as consistently steering Mexican workers toward low-paying positions and suggesting stereotypical secretarial roles to women. This research underscores the significance of evaluating LLM biases in real-world applications to comprehend their potential for perpetuating harm and generating inequitable outcomes.
To delve deeper, read the full summary here.
📰 Article summaries:
The AI Revolution Is Crushing Thousands of Languages - The Atlantic
What happened: The dominance of English in digital spaces, coupled with the surge in generative AI technology, poses a significant threat to linguistic diversity. Despite the vast number of languages spoken globally, the majority of online content and AI applications are in English or a select few other languages. This trend risks further marginalizing Indigenous and low-resource languages, potentially erasing them from the digital landscape altogether.
Why it matters: Efforts are underway to address this imbalance, with initiatives like Masakhane striving to develop AI tools for underrepresented languages. There's hope that AI models, trained on more commonly spoken languages, can exhibit some degree of universality, aiding in preserving minority languages. However, the challenges are immense, requiring extensive resources and collaboration to create and curate training data for thousands of languages.
Between the lines: The rapid spread of technology and the internet may exacerbate the decline of minority languages, with younger generations losing interest in their ancestral tongues. To combat this, it's crucial to engage native speakers in discussions about how AI can effectively serve their linguistic needs. Building platforms tailored to these languages' linguistic and cultural contexts could ensure their presence and relevance in the digital sphere for future generations.
Why the Chinese government is sparing AI from harsh regulations—for now | MIT Technology Review
What happened: Angela Huyue Zhang, a law professor, outlines a three-phase pattern in China's tech regulation: initial leniency, sudden crackdowns, and eventual relaxation. This cycle is exemplified by the trajectory of tech giants like Alibaba and Tencent, which faced minimal scrutiny as they rapidly expanded, only to be hit with severe fines and antitrust investigations later.
Why it matters: Zhang's analysis underscores the volatile nature of China's tech regulation, where shifts between laxity and stringency can profoundly impact companies and markets. Despite persistent issues like competition obstruction and privacy violations, regulators historically turned a blind eye until sudden crackdowns occurred. The government's current emphasis on AI reflects its strategic importance for China's economic growth, leading to comparatively loose regulation compared to Western counterparts.
Between the lines: The Cyberspace Administration of China (CAC) plays a key role in AI regulation, prioritizing political control over technological innovation. While some restrictions aim to curb undesirable content, they may stifle innovation and competitiveness. Zhang suggests that significant AI misuse could trigger a sudden, severe regulatory response, highlighting the unpredictability and potential consequences of China's regulatory landscape.
Speed of AI development stretches risk assessments to breaking point
What happened: As AI technology rapidly advances, traditional methods for evaluating its performance, accuracy, and safety are struggling to keep pace. The emergence of more powerful AI models, fueled by significant investment from tech giants and venture capitalists, has rendered many older evaluation criteria obsolete in a matter of months rather than years.
Why it matters: With AI systems constantly evolving, existing benchmarks quickly become outdated as new models effortlessly surpass them. This shift has moved the challenge of evaluating AI from academia to boardrooms, with CEOs prioritizing generative AI investments. Ensuring trustworthy AI products is crucial for gaining user trust, driving companies and governments to grapple with deploying and managing the risks associated with these advanced technologies.
Between the lines: While platforms like Hugging Face offer user-driven leaderboards to assess AI models, companies require more tailored evaluations to meet specific needs. Building internal test sets and prioritizing human evaluation methods are becoming essential strategies for businesses navigating the complex landscape of AI model selection. Choosing the right AI model is as much about intuition and real-world testing as it is about metrics and benchmarks.
📖 From our Living Dictionary:
How dynamic pricing hinted at algorithmic pricing
👇 Learn more about why it matters in AI Ethics via our Living Dictionary.
🌐 From elsewhere on the web:
Making Responsible AI the Norm rather than the Exception
This report prepared by the Montreal AI Ethics Institute provides recommendations in response to the National Security Commission on Artificial Intelligence (NSCAI) Key Considerations for Responsible Development and Fielding of Artificial Intelligence document. The report centers on the idea that Responsible AI should be made the Norm rather than an Exception. It does so by utilizing the guiding principles of: (1) alleviating friction in existing workflows, (2) empowering stakeholders to get buy-in, and (3) conducting an effective translation of abstract standards into actionable engineering practices. After providing some overarching comments on the document from the NSCAI, the report dives into the primary contribution of an actionable framework to help operationalize the ideas presented in the document from the NSCAI. The framework consists of: (1) a learning, knowledge, and information exchange (LKIE), (2) the Three Ways of Responsible AI, (3) an empirically-driven risk-prioritization matrix, and (4) achieving the right level of complexity. All components reinforce each other to move from principles to practice in service of making Responsible AI the norm rather than the exception.
To delve deeper, read more details here.
💡 In case you missed it:
Fair allocation of exposure in recommender systems
Within the domain of recommender systems, algorithmic decisions regarding content exposure carry significant ethical implications, potentially marginalizing minority or disadvantaged content producers. In a series of works [2,3,4], we propose to define the fairness of ranked recommendations based on principles from economic fair division. Following these principles, we introduce new recommendation algorithms and show that they can distribute exposure more fairly among content producers while preserving the quality of recommendations for users.
To delve deeper, read the full article here.
Take Action:
We’d love to hear from you, our readers, on what recent research papers caught your attention. We’re looking for ones that have been published in journals or as a part of conference proceedings.